Introduction
In the world of statistics, numbers can be daunting, but summary statistics act as a beacon of clarity. Imagine you’re a detective sifting through mountains of data—summary statistics are your trusty magnifying glass. They help summarize vast amounts of information into digestible insights, making it easier to identify trends, anomalies, and critical insights within datasets. In this article, we will explore various summary statistics examples and how they can be applied across different fields, from academia to business analytics. Buckle up as we embark on a journey to demystify summary statistics and learn to wield them like a pro!
And speaking of wielding knowledge, if you’re looking to deepen your understanding of data and statistics, consider picking up a copy of Statistical Methods for the Social Sciences. This book is a treasure trove of knowledge that will guide you through the complexities of social data analysis!
Summary Section
Summary statistics condense complex data into meaningful insights, allowing us to grasp essential characteristics at a glance. The key areas we will cover include:
- Measures of Central Tendency: These metrics, including mean, median, and mode, reveal where data tends to cluster, offering a snapshot of typical values. For instance, the mean can help you understand the average test score in a class, while the median provides insight into the middle score, highlighting potential outliers. You can learn more about the what does mean identically distributed in statistics.
- Measures of Dispersion: Understanding how data points vary from one another is just as crucial. Metrics like range, variance, and standard deviation help quantify this variability. For example, a high standard deviation in sales figures may indicate fluctuating performance, while a low standard deviation suggests consistent results. To explore the range further, check out the orange county ca crime statistics.
- Graphical Representations: Visual tools like histograms and box plots enhance our understanding of data distributions and reveal patterns that numbers alone may obscure. A box plot can illustrate the range and interquartile range, making it easy to spot outliers quickly.
By the end of this article, you’ll be equipped with examples and practical applications of summary statistics, empowering you to analyze data effectively, whether you’re a student, researcher, or business analyst. With a solid grasp of these concepts, you’ll tackle data analysis with confidence and flair, ready to impress with your newfound knowledge!
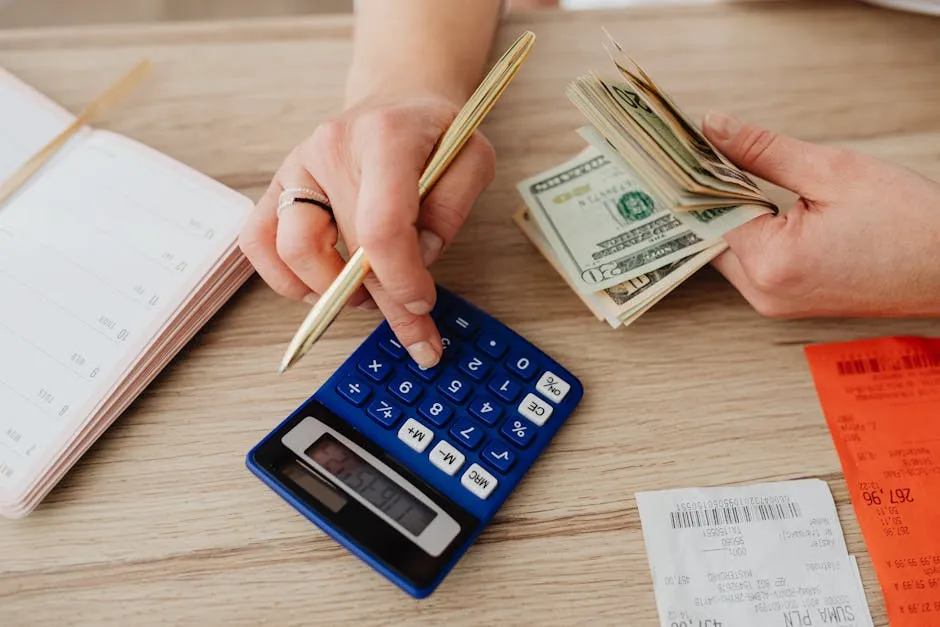
What are Summary Statistics?
Definition and Importance
Summary statistics are powerful tools in the realm of data analysis. They provide a condensed view of a dataset, enabling quick insights into its characteristics. Think of them as the “highlights” reel for your data. Instead of wading through every single entry in a spreadsheet, summary statistics summarize essential information, allowing you to grasp the dataset’s core features at a glance.
Why are these statistics so vital? When faced with complex datasets, it’s easy to feel overwhelmed. Summary statistics break down the noise, simplifying data interpretation. They help in identifying trends, spotting outliers, and understanding distributions. This simplification is particularly crucial in fields like business, healthcare, and academia, where critical decisions are often based on data analysis. A well-chosen summary statistic can reveal patterns that are otherwise obscured, guiding informed decision-making.
To enhance your data analysis toolbox, you might want to consider How to Measure Anything: Finding the Value of “Intangibles” in Business. This enlightening book dives deep into the art of measurement, transforming how you perceive intangible assets in your business.
Categories of Summary Statistics
Summary statistics can be categorized into two main types: measures of central tendency and measures of dispersion.
Measures of Central Tendency: These statistics indicate where data points tend to cluster. The three primary measures in this category are:
- Mean: Often referred to as the average, the mean is calculated by summing all data points and dividing by the number of points. For example, if you have the dataset [10, 20, 30], the mean would be (10 + 20 + 30) / 3 = 20.
- Median: The median is the middle value of a sorted dataset. It’s particularly useful when the data distribution is skewed. For instance, in the dataset [1, 3, 5, 7, 9], the median is 5. If the dataset were [1, 3, 5, 7, 9, 11], the median would be (5 + 7) / 2 = 6. For more insights, check the statistics poland median salary 2024.
- Mode: The mode is the most frequently occurring value in a dataset. For instance, in the dataset [1, 2, 2, 3, 4], the mode is 2, as it appears most often. You can dive deeper into this topic with the statsmodels residuals statistics.
Measures of Dispersion: These statistics showcase how spread out the data points are. They include:
- Range: The difference between the highest and lowest values in a dataset. For example, for the dataset [10, 15, 20, 25], the range is 25 – 10 = 15.
- Variance: Variance measures how far each number in the dataset is from the mean and consequently from every other number. It’s calculated by averaging the squared differences from the mean.
- Standard Deviation: This statistic provides insight into how much variation exists from the average. A low standard deviation indicates that the data points tend to be close to the mean, while a high standard deviation indicates that the data points are spread out over a wider range of values.
Understanding these categories helps in choosing the right summary statistic for your analysis, ensuring clarity and accuracy in data interpretation.
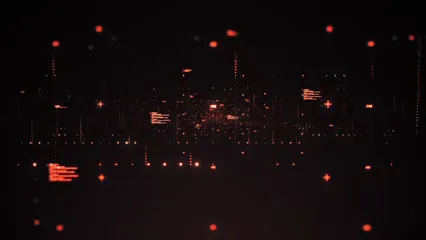
To keep your notes organized while you learn, grab a Graph Paper Notebook. It’s perfect for sketching out graphs and visualizing your data!
Examples of Summary Statistics
Numerical Examples
Example 1: Calculating the Mean
Let’s calculate the mean using the dataset: [10, 20, 30, 40, 50].
- Step 1: Add the numbers together: 10 + 20 + 30 + 40 + 50 = 150
- Step 2: Count the numbers: There are 5 numbers.
- Step 3: Divide the total by the count: 150 / 5 = 30
Thus, the mean of the dataset is 30.
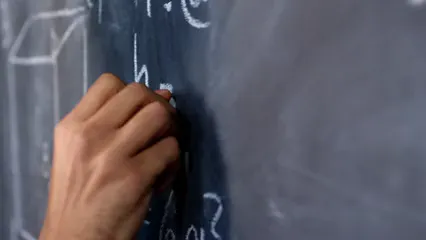
Example 2: Understanding the Median
Now, let’s find the median for the dataset: [7, 3, 9, 1, 5].
- Step 1: Sort the dataset in ascending order: 1, 3, 5, 7, 9
- Step 2: Identify the middle value: The middle value is 5.
Therefore, the median of this dataset is 5.
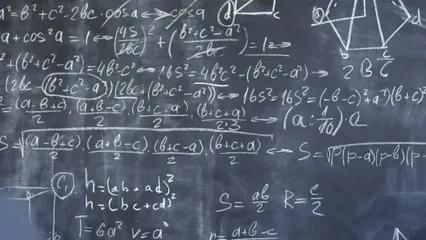
Example 3: Analyzing the Mode
Finally, we will analyze the mode for the dataset: [1, 2, 2, 3, 4].
- Step 1: Identify the most frequent number: The number 2 appears twice, more than any other number.
Thus, the mode of this dataset is 2.
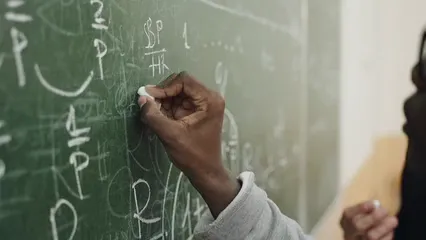
These examples illustrate the fundamental calculations involved in determining summary statistics. They serve as the building blocks for more complex analyses, paving the way for deeper insights into data trends and relationships.
Application Examples
Example 4: Business Analytics
Businesses harness the power of summary statistics to navigate the ever-changing market landscape. When analyzing sales data, companies can quickly identify trends and patterns. For instance, a retail store might track monthly sales figures. By calculating the mean sales, they understand typical performance. However, the median sales figure can reveal a more accurate picture, especially if a few exceptionally high sales skew the mean.
Customer satisfaction surveys also benefit greatly from summary statistics. An organization may collect responses on a scale from 1 to 10. The mean score offers a quick glance at overall satisfaction. But what if the responses are unevenly distributed? Here, the mode—the most frequently given score—might provide insights into the general sentiment. This dual approach empowers businesses to make informed decisions, enhance customer experience, and ultimately drive profits.
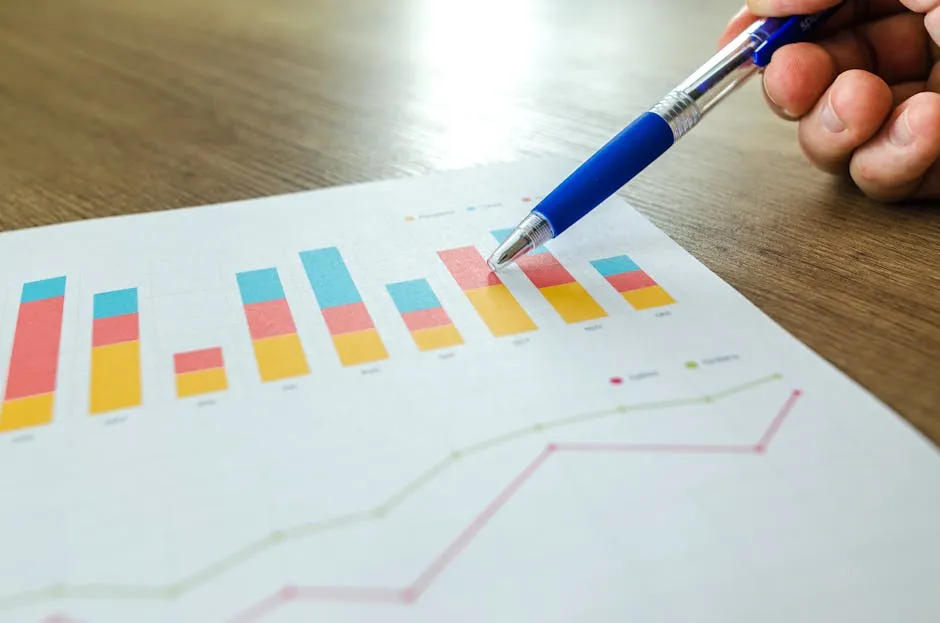
For those diving into business analytics, Business Analytics: Data Analysis and Decision Making is a must-read! It provides practical insights into leveraging data for impactful business decisions.
Example 5: Academic Research
In academia, summary statistics act as essential tools for researchers analyzing data gathered from surveys or experiments. Consider a researcher investigating student performance across various teaching methods. By collecting test scores and demographic information, they can calculate summary statistics to identify patterns.
For example, the mean score might indicate overall performance levels. However, a closer look at the standard deviation reveals how much scores vary. A low standard deviation suggests a consistent performance among students, while a high value indicates differing outcomes. This insight is crucial for understanding the effectiveness of the teaching methods employed.
Additionally, when researchers conduct surveys, summary statistics help them paint a clear picture of their findings. They can summarize demographics, like age and education level, using mean and median values, providing context for their research. By effectively using summary statistics, researchers not only streamline their analysis but also enhance the credibility of their findings, making them more impactful in the academic community.

For those pursuing research, be sure to check out Data Science for Business. It will provide you with the tools necessary to apply data analysis effectively within your research projects.
Graphical Representations
Importance of Graphical Data Representation
Visualizations bring summary statistics to life! They transform dull numbers into captivating visuals that everyone can understand. Imagine trying to explain your favorite dish without tasting it. Tough, right? Similarly, data without visuals is like a meal without flavor. Graphs and charts simplify complex data, making it easier to spot trends and patterns. They also help highlight outliers, which can be crucial in data analysis. For instance, a sudden spike in sales might be a sign of an effective marketing campaign—or perhaps a pricing error! By using graphical representations, you can convey insights more effectively and engage your audience.
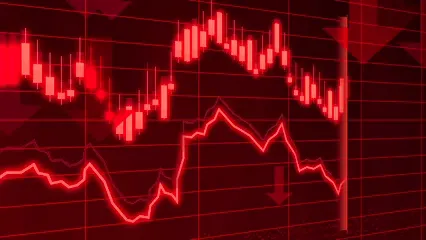
To make your presentations more engaging, consider investing in a Portable Projector for Presentations. This will help you showcase your data visually and make your points resonate with your audience!
Examples of Graphs and Charts
Example 1: Histogram
Creating a histogram from your dataset is a breeze! Here’s how you can do it:
- Step 1: Gather your data. Let’s say you have the following exam scores: [56, 67, 70, 75, 80, 82, 85, 88, 90, 95].
- Step 2: Determine your bins. For this dataset, you might choose bins of 10 points (50-59, 60-69, etc.).
- Step 3: Count the frequency of scores falling into each bin. For example: – 50-59: 1 score – 60-69: 2 scores – 70-79: 3 scores – 80-89: 3 scores – 90-99: 1 score
- Step 4: Draw the histogram. On the x-axis, label your bins. On the y-axis, represent frequency. Each bin is a bar, with height corresponding to the number of scores in that range. Voilà! You’ve created a histogram that visually represents your data distribution.
Example 2: Box Plot
Constructing a box plot? Let’s break it down:
- Step 1: Collect your numerical data. For instance, consider these salaries: [30000, 35000, 40000, 45000, 50000, 55000, 60000].
- Step 2: Sort the data and identify the five-number summary: – Minimum: 30000 – Q1 (25th percentile): 37500 – Median (50th percentile): 45000 – Q3 (75th percentile): 52500 – Maximum: 60000
- Step 3: Draw the box plot. Create a number line and mark the minimum and maximum. Draw a box from Q1 to Q3, and mark the median inside the box. Finally, extend “whiskers” from the box to the minimum and maximum values. Your box plot now reveals the distribution, central tendency, and potential outliers in your salary data!
Conclusion
In summary, summary statistics serve as the backbone of data analysis, providing essential insights that can guide decision-making across various fields. By mastering the concepts and calculations surrounding summary statistics, you can unlock the potential of data and enhance your analytical skills. Whether you’re working on a research project, making business decisions, or simply trying to make sense of everyday data, summary statistics will prove invaluable. They simplify complexities, making it easier to communicate findings and engage your audience effectively. So, embrace the power of summary statistics and let data drive your decisions!
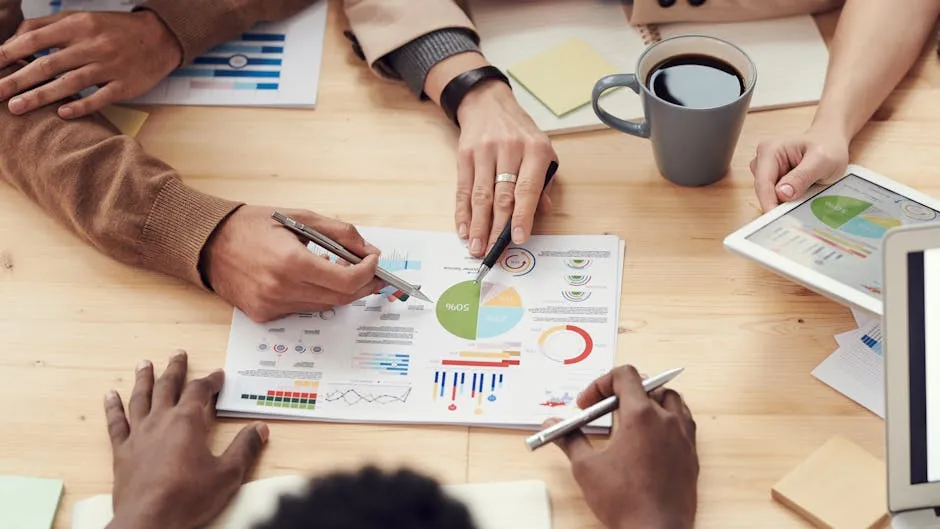
And if you’re looking to improve your data collection methods, don’t forget the importance of having a reliable Digital Scale for Weighing. It’s a great tool for precise measurements, whether for research or everyday use!
FAQs
What are summary statistics?
Summary statistics are numerical values that provide a concise description of a dataset. They help to summarize key features, making complex data more manageable and understandable.
Why are they important?
They simplify complex data, making it easier to identify trends and make decisions. With summary statistics, you can quickly grasp the main characteristics of data without getting lost in the details.
What are the main types of summary statistics?
The main types include measures of central tendency (mean, median, mode) and measures of dispersion (range, variance, standard deviation). These metrics give insights into data distribution and variability.
How can I visualize summary statistics?
Common methods include histograms, box plots, and scatter plots. These visualizations help illustrate the data’s distribution and highlight important patterns at a glance.
In what fields are summary statistics used?
They are widely used in business, academia, healthcare, and social sciences. Summary statistics serve as essential tools across these sectors for informed decision-making and effective data analysis.
Please let us know what you think about our content by leaving a comment down below!
Thank you for reading till here 🙂
All images from Pexels