Introduction
The Central Limit Theorem (CLT) stands as a pillar in the realm of statistics. This theorem explains why we can often treat sample means as if they follow a normal distribution, even when the underlying population doesn’t. Quite the party trick, right?
So, what exactly is the Central Limit Theorem? In simple terms, it states that as we draw larger samples from a population, the distribution of the sample means tends to become normal, regardless of the population’s shape. This is important because it allows statisticians to make reliable inferences about a population using sample data.
For statisticians, the CLT is not just a fancy concept; it’s a lifeline. Imagine trying to understand a population’s characteristics based solely on a handful of observations. Without the CLT, that would be like trying to predict the weather by looking out your window once a week. The CLT provides a framework for making sense of data, especially when dealing with sampling distributions.
Why is this significant? The applications of the CLT are widespread. For instance, it plays a crucial role in hypothesis testing. This means that when researchers want to assert whether a certain claim about a population holds true, they can rely on the properties bestowed by the CLT. Also, confidence intervals—those handy tools for estimating population parameters—are grounded in this theorem.

Understanding the role of the CLT in hypothesis testing can be enhanced by referring to the statistics hypothesis testing cheat sheet.
In this article, we’ll dive into the reasons why the Central Limit Theorem is essential for statisticians and researchers alike. We’ll explore its implications in various statistical methods and how it simplifies the analysis of complex data. Buckle up, as we embark on this statistical adventure!
To prepare for your journey into the world of statistics, consider picking up a copy of Central Limit Theorem Explained. This book breaks down complex concepts into digestible pieces, making it an essential addition to your statistical library.
What is the Central Limit Theorem?
The Central Limit Theorem is a fundamental concept in statistics that describes the characteristics of sampling distributions. To put it simply, it tells us that when we take a sufficiently large number of samples from any population, the mean of those samples will be normally distributed.
But what exactly does this mean? Let’s break it down. Imagine you’re a baker, and you want to know the average weight of your cookies. If you pull a few cookies from the oven and weigh them, you might get a skewed or uneven representation of the entire batch. However, if you take many samples, say 30 or more, and calculate their means, the distribution of those means will tend to resemble a bell-shaped curve, even if the weights of individual cookies are all over the place.
Mathematically, the CLT can be summarized as follows: if you have a set of independent and identically distributed (i.i.d.) random variables, the sampling distribution of the sample mean will approach a normal distribution as the sample size increases. This holds true regardless of whether the original data is normally distributed or not.
For the theorem to hold true, certain conditions must be met. First, the sample size should be sufficiently large; typically, a size of 30 is deemed adequate. Second, the samples need to be independent of each other. Lastly, the population from which samples are drawn should have a finite variance. If these conditions are satisfied, we can confidently apply the CLT.
Understanding the Central Limit Theorem is crucial for any statistician. It not only provides a foundation for statistical inference but also simplifies the analysis of data that may not follow a normal distribution. As we continue this discussion, you’ll see just how vital the CLT is across various statistical applications!
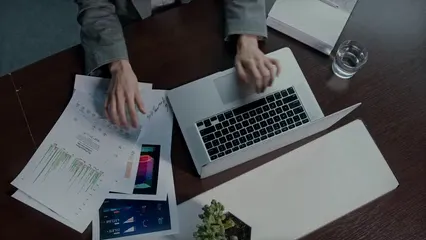
If you’re serious about data analysis, consider adding Statistics for Dummies to your collection. This resource is perfect for beginners and seasoned pros alike, providing clarity and guidance through the often murky waters of statistical analysis.
Key Components of the Central Limit Theorem
The Central Limit Theorem (CLT) is like a magic wand in the world of statistics, transforming the chaotic nature of samples into a more predictable form. At its core, the CLT reveals how the mean and standard deviation of sample distributions behave, particularly as sample size grows. Let’s break down these key components for a clearer understanding.
First, let’s talk about the mean of the sampling distribution. According to the CLT, the mean of the sampling distribution is equal to the mean of the population from which the samples are drawn. This means if you were to take multiple samples and calculate their means, those means would center around the true population mean. It’s like gathering enough friends for a group photo; the more, the merrier, and the closer you get to capturing the true vibe of the party!
Next up, we have the standard deviation of the sampling distribution, often called the standard error. This value is calculated by dividing the standard deviation of the population by the square root of the sample size. So, if your population has a standard deviation of 10, and you take a sample size of 25, the standard error would be 10 divided by the square root of 25, which equals 2. This means that as you increase your sample size, the variability of the sample means decreases. It’s like trying to predict the average height of your friends; the more you measure, the closer you get to the real average height!
Now, let’s discuss why a sample size of 30 is often regarded as the magic number. Statisticians generally agree that when the sample size reaches 30 or more, the sampling distribution of the mean will approximate a normal distribution, even if the population itself is not normally distributed. This is significant because it allows researchers to use the robust properties of normal distributions in their analyses. So, if you’ve got 30 or more friends at that party, you can confidently assume they represent the crowd well!
Consider this: if you take a smaller sample size, say 10, the means of those samples might still reflect the population’s quirks and oddities. In contrast, with a larger sample size, the means become more stable and reliable. For example, if you were to sample the ages of 10 randomly selected people in a neighborhood known for its wide age range, you might get a skewed average. But if you sampled 100 people, that average would likely trend closer to the neighborhood’s actual average age.
In conclusion, understanding the mean and standard deviation of sampling distributions is crucial. These components provide a foundation for making statistical inferences, allowing us to treat sample statistics as reliable estimates of population parameters. By ensuring that sample sizes are sufficiently large, we can wield the power of the Central Limit Theorem to simplify our analyses and enhance our understanding of data. So, next time you’re gathering data, remember: more is often merrier!

If you want to dive deeper into statistical methods, check out The Art of Statistics: Learning from Data. It’s an engaging read that helps you understand how statistical concepts apply in real-world scenarios.
Modeling and Simulation
The Central Limit Theorem (CLT) is a superhero in the world of modeling and simulation. Why, you ask? Because it transforms the unwieldy chaos of data into something manageable and predictable! Picture this: you’re trying to model the behavior of a complex system, like predicting the stock market. Without the CLT, you’d be flying blind, navigating a stormy sea of data with no compass.
In modeling and simulation, the CLT plays a crucial role. It allows statisticians to assume that the means of random samples will follow a normal distribution, even if the original data doesn’t. This is especially useful when you’re simulating scenarios based on various random variables. Think about it: if you’re running simulations to predict outcomes, you want a reliable framework to base those predictions on. Here enters our hero, the CLT!
Let’s consider a practical example. Suppose you’re modeling customer spending in a retail store. You collect data from a select group of customers, but their spending habits may vary wildly. By increasing your sample size and applying the CLT, you can confidently assert that the average spending will approximate a normal distribution. This means you can make predictions about overall customer behavior based on this average. Who wouldn’t want to know how much to stock up on that new trendy item?
Moreover, the CLT empowers researchers conducting Monte Carlo simulations. In these scenarios, random sampling plays a pivotal role in estimating complex probabilities. For instance, if you’re estimating the risk of a particular investment, you run thousands of simulations based on different market conditions. Thanks to the CLT, you can treat the average of those results as normally distributed, allowing for more accurate risk assessments.
Let’s not forget about the beauty of the normal distribution! It’s nice, neat, and comes with a plethora of statistical tools and techniques designed to work with it. This is where the CLT shines, providing the underlying structure necessary for many statistical methods. Want to calculate confidence intervals? Need to conduct hypothesis testing? The CLT makes it all possible, giving statisticians the confidence to draw conclusions from their models.
In conclusion, the Central Limit Theorem is the backbone of modeling and simulation in statistics. Its power lies in its ability to allow researchers to assume normality in the sampling distribution of means, regardless of the underlying population distribution. So, whether you’re predicting customer trends or simulating financial markets, the CLT is your trusty sidekick, guiding you through the complexities of data analysis with a wink and a smile.

If you’re looking for a solid reference guide, consider grabbing a copy of Statistical Inference. This book provides a comprehensive overview of the principles and methods of statistical inference, making it invaluable for anyone serious about data analysis.
Central Limit Theorem vs. Other Theorems
The Central Limit Theorem (CLT) is often compared to other key statistical theorems, especially the Law of Large Numbers (LLN). Both play vital roles in statistics, but they serve different purposes and have unique implications.
At its core, the CLT tells us that as the sample size increases, the distribution of the sample mean becomes approximately normal, regardless of the population’s actual distribution. This means that even if you’re sampling from a population that’s as skewed as a banana, the average of those samples will eventually resemble a bell curve. This is a game-changer for statisticians!
Now, let’s give a nod to the Law of Large Numbers. While the CLT focuses on the distribution of sample means, the LLN assures us that as we take more samples, the average of those samples will converge to the population mean. Think of it this way: the LLN is like a wise old owl, telling you that if you keep sampling, your average will get closer and closer to the true average of the population. However, it doesn’t guarantee that the distribution of those averages will be normal.
So, how do these two theorems relate? The LLN lays the groundwork for the CLT. You need a good grasp of the LLN to fully appreciate the CLT. If the LLN tells you that your sample mean will eventually reflect the population mean, the CLT takes it a step further, explaining how the distribution of that mean behaves as your sample size grows.
The differences in implications are significant. The LLN reassures researchers that larger samples yield more accurate estimates of the population mean. In contrast, the CLT allows statisticians to apply normal distribution techniques, like hypothesis testing and confidence intervals, to sample means from non-normally distributed populations. Without the CLT, statisticians would be left floundering in a sea of complexity, unable to make reliable inferences from their data.
In summary, while both the CLT and LLN are essential, they serve distinct purposes. The LLN is about accuracy in estimating population parameters, while the CLT is about understanding how sample means behave. Together, they form a powerhouse duo, making the world of statistics a little less intimidating and a lot more enlightening.
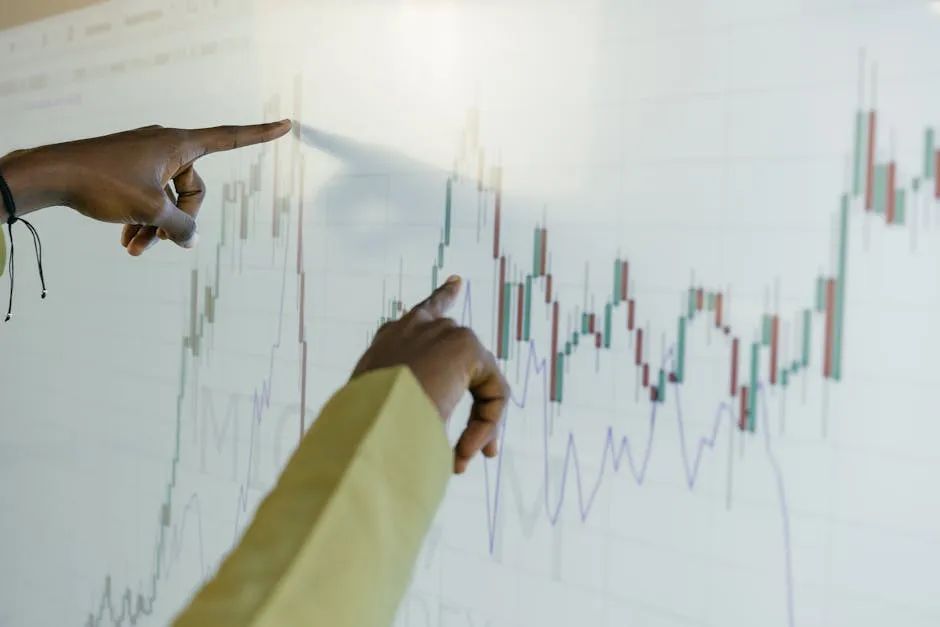
To further enhance your understanding of statistical methodologies, consider exploring The Elements of Statistical Learning. This book delves into advanced statistical techniques and is a must-read for anyone looking to deepen their analytics skills.
Conclusion
In wrapping up our exploration of the Central Limit Theorem (CLT), its significance in statistics cannot be overstated. The CLT serves as a cornerstone for making reliable inferences about populations from sample data. By establishing that the distribution of sample means approaches normality, the CLT empowers researchers to apply various statistical techniques, regardless of the population’s original distribution.
One of the standout features of the CLT is its ability to simplify the analysis of complex data. Even if you’re dealing with a wildly skewed dataset, the CLT assures you that, with a sufficiently large sample size, your sample means will follow that familiar bell curve. This is particularly useful when constructing confidence intervals and conducting hypothesis tests, as it provides a solid foundation for statistical reasoning.
Moreover, understanding the CLT enhances statistical literacy. Knowing how and when to apply the CLT can elevate your research skills, leading to more robust conclusions. In an age where data drives decision-making, having a firm grasp on the CLT is like having a trusty GPS in the world of statistics. It guides you through the complexities of data analysis, ensuring that your estimates are not just educated guesses but informed predictions grounded in statistical theory.
As we navigate the seas of statistical analysis, let’s remember that the Central Limit Theorem is more than just a theorem; it’s a powerful tool that bridges the gap between sample data and population insights. So, whether you’re crunching numbers in a lab or analyzing trends in your business, the CLT is your steadfast companion, helping you make sense of the chaos.
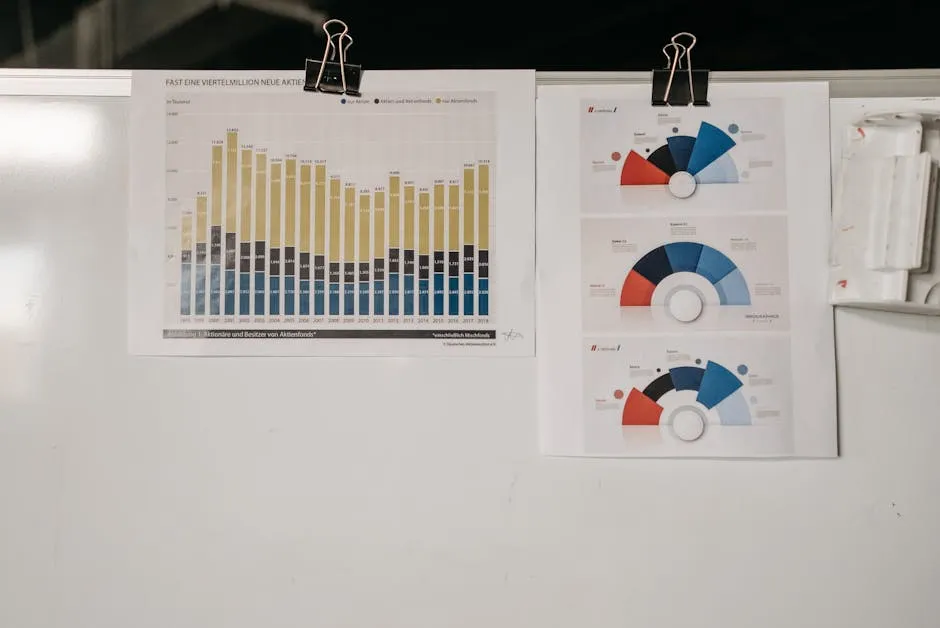
If you’re looking to enhance your data analysis toolkit, consider investing in a Graphing Calculator. It’s an essential tool for visualizing functions and data, making it easier to grasp complex statistical concepts.
FAQs
What is the central limit theorem in simple terms?
The Central Limit Theorem (CLT) suggests that when you take a large number of samples from a population, the average of those samples will resemble a normal distribution, even if the population itself isn’t normally distributed. Think of it as a statistical guarantee that helps us make sense of data. It’s like having a magic wand that transforms chaos into order.
Why is a sample size of 30 commonly used?
Why 30, you ask? Statisticians have found that a sample size of 30 is typically large enough for the CLT to kick in. With 30 or more observations, the distribution of sample means starts to approximate normality. This magic number gives researchers confidence, ensuring their analyses yield reliable results. Imagine trying to predict the average height of a group of friends. If you measure just a few, you might get a weird mix. But get 30 measurements, and you’ll likely hit the nail on the head!
Can the CLT be applied to non-normally distributed populations?
Absolutely! One of the most exciting aspects of the CLT is its versatility. It can be applied to populations that aren’t normally distributed. Whether you’re dealing with a skewed distribution, like income levels, or a bimodal distribution, the CLT ensures that as your sample size increases, the distribution of the sample means will inch closer to normal. Even if your data looks like a Picasso painting, the CLT helps bring a little order to the chaos!
How does the CLT affect confidence intervals?
The CLT plays a crucial role in constructing confidence intervals. When researchers want to estimate a population parameter, they rely on sample means. Thanks to the CLT, they can confidently use the normal distribution to create these intervals. This means that even if the original data is skewed, as long as the sample size is large enough, the confidence intervals around the sample mean will be accurate. It’s like having a trusty umbrella; even if the weather is unpredictable, you can still expect some protection against the rain!
Please let us know what you think about our content by leaving a comment down below!
Thank you for reading till here 🙂
All images from Pexels