Introduction
Inferential statistics is like the magician of the data world. It takes a handful of data from a sample and makes broad predictions about a larger population. This is crucial in research and data analysis, where gathering data from an entire population is often impractical. By using inferential statistics, researchers can estimate population parameters and test hypotheses, enabling them to make informed decisions based on sample data.
However, as with all magic tricks, there are hidden pitfalls. One major issue is the over-reliance on p-values. Yes, p-values have become the poster child for significance testing, but they can be misleading when misused. This brings us to the second issue: the misuse of statistical tests. Often, researchers treat these tests as sacred cows, applying them without a solid understanding of their assumptions and limitations. The gap between statistical theory and practical application is another problem. Many researchers know the formulas but struggle to interpret the results effectively.
The purpose of this article is to unpack these problems with inferential statistics. We’ll highlight better practices and alternatives to improve data analysis outcomes. After all, understanding the limitations of inferential statistics can help researchers become more effective in their work. Let’s dive into the nitty-gritty and uncover how we can better utilize statistical methods in research.
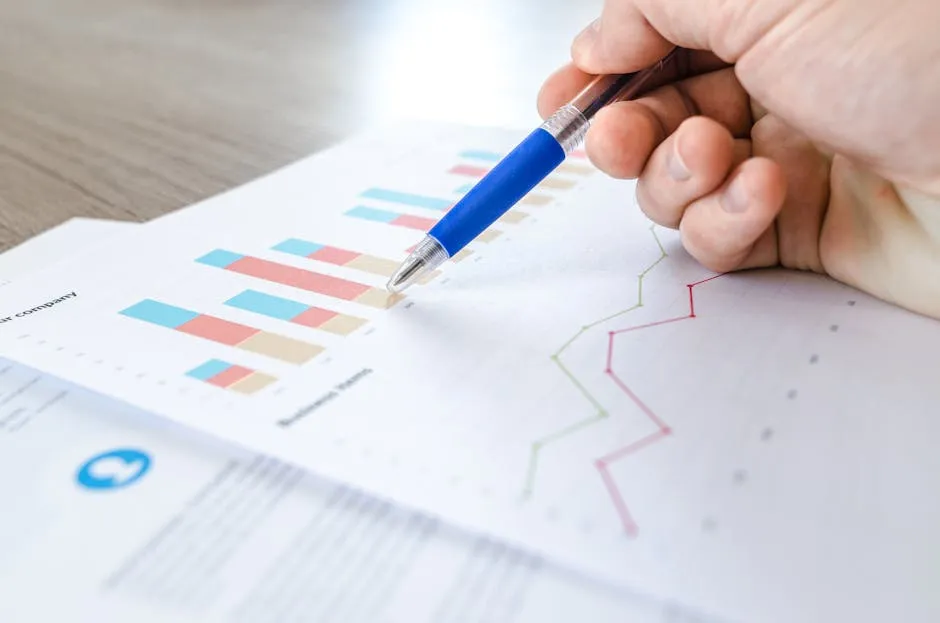
Understanding Inferential Statistics
What is Inferential Statistics?
Inferential statistics allows researchers to make predictions about a population based on a sample. Unlike descriptive statistics, which merely summarize data, inferential statistics takes it a step further. It helps researchers draw conclusions and make estimates about larger groups.
Common methods within inferential statistics include hypothesis testing and the creation of confidence intervals. Hypothesis testing involves formulating a null hypothesis, which states there’s no effect or difference, and an alternative hypothesis, which suggests there is. Researchers then use sample data to determine whether to reject the null hypothesis.
Confidence intervals provide a range of values, offering a degree of certainty about where the true population parameter lies. For instance, if a study shows a confidence interval for the mean height of adult males as 5’8” to 5’10”, researchers can be reasonably confident that the actual mean falls within that range.
In summary, inferential statistics is a powerful tool that allows researchers to extend their findings beyond their immediate data. Just remember, with great power comes great responsibility. Understanding when and how to apply these methods is crucial for valid results.

If you’re looking to deepen your understanding of these concepts, consider picking up “The Art of Statistics: Learning from Data” by David Spiegelhalter. This book is a fantastic resource that demystifies statistics and teaches you how to think critically about data.
The Role of Sampling
Sampling is the backbone of inferential statistics. Choosing the right sample is crucial for valid results. If your sample is a party of one, you’re likely to have a very biased guest list. Think of it this way: if you only ask your best friend about their favorite ice cream flavor, you might think everyone loves chocolate chip mint. But is that really true? Probably not!
A well-chosen sample can represent the larger population. It acts as a mini-version of the whole. This is where randomness comes into play. Random sampling ensures each member of the population has an equal chance of being selected, reducing bias and increasing the reliability of your results. Say goodbye to the days of cherry-picking data!
Now, let’s talk about sampling error. This sneaky little rascal happens when there’s a difference between the sample and the actual population. Even with the best intentions and a solid sampling method, some level of error is inevitable. Sampling error can lead to incorrect conclusions, making it essential to understand its implications.
Imagine conducting a survey to find out the average height of players on a basketball team. If you only measure the tallest player and declare that as the average, you might be in for a surprise. The actual average could be much lower. Sampling error can distort research findings, leading to misguided decisions.
So, what can we do about sampling error? For starters, increasing the sample size often helps. The larger the sample, the closer it tends to reflect the population. But, of course, bigger isn’t always better if the sampling method itself is flawed. Quality matters just as much as quantity.
In summary, the role of sampling is pivotal in inferential statistics. A well-chosen sample can provide valuable insights about a larger group. However, researchers must remain vigilant about sampling errors and their consequences. A little caution goes a long way in ensuring that your findings are as accurate and representative as possible. So, next time you set out to gather data, remember: the right sample can make all the difference!
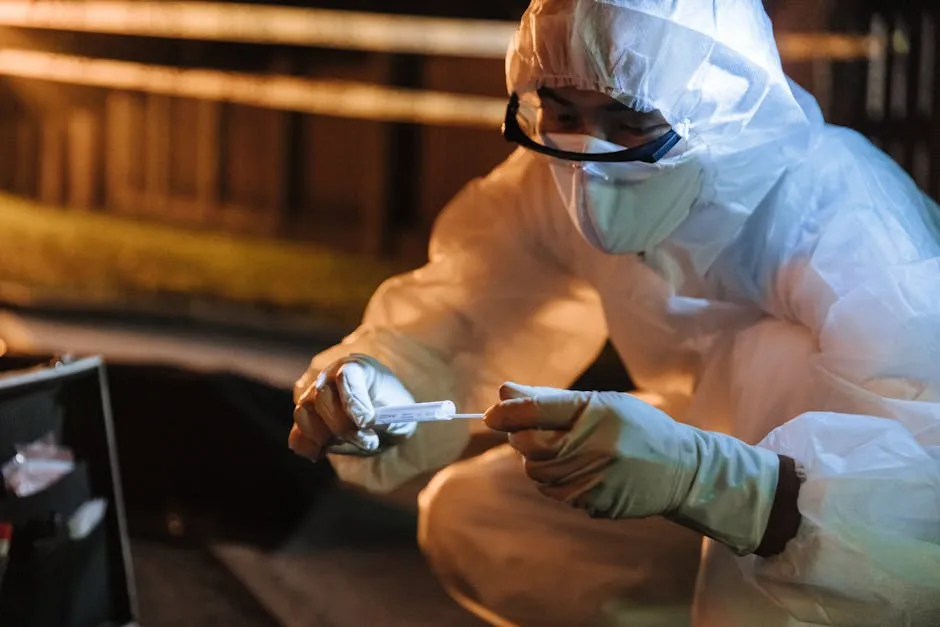
If you want to get a handle on the concepts of sampling and more, check out “Naked Statistics: Stripping the Dread from the Data” by Charles Wheelan. It’s a fun read that makes statistics approachable and enjoyable!
Misinterpretation of Results
Inferential statistics can be a slippery slope. If the models and assumptions behind them are flawed, researchers may reach incorrect conclusions. This misinterpretation can lead to disastrous outcomes, especially when those conclusions influence policy or clinical practices.
Take the OPERA experiment, for instance. Researchers at CERN reported neutrinos traveling faster than light, with a shocking p-value of 0.0000003. This made headlines worldwide, prompting debates about Einstein’s theories. However, the excitement fizzled when it was revealed that a loose cable had skewed the results. The p-value was correct, but the underlying model failed due to incorrect assumptions. This story highlights how easy it is to misinterpret results when we lean too heavily on statistical outputs without questioning their validity.
Another classic example comes from medical research. In a study on a new drug, researchers found a statistically significant improvement in patient outcomes. However, they neglected to consider the patients’ overall health and the context of their conditions. The positive results, while statistically valid, didn’t translate into real-world benefits. This illustrates the danger of taking inferential statistics at face value. Just because the numbers seem promising doesn’t guarantee they reflect reality.
In summary, the misinterpretation of results often stems from a failure to adequately assess the models and assumptions in use. It’s crucial for researchers to scrutinize their methods and ensure that statistical significance aligns with practical significance. Only then can we trust the conclusions drawn from inferential statistics.
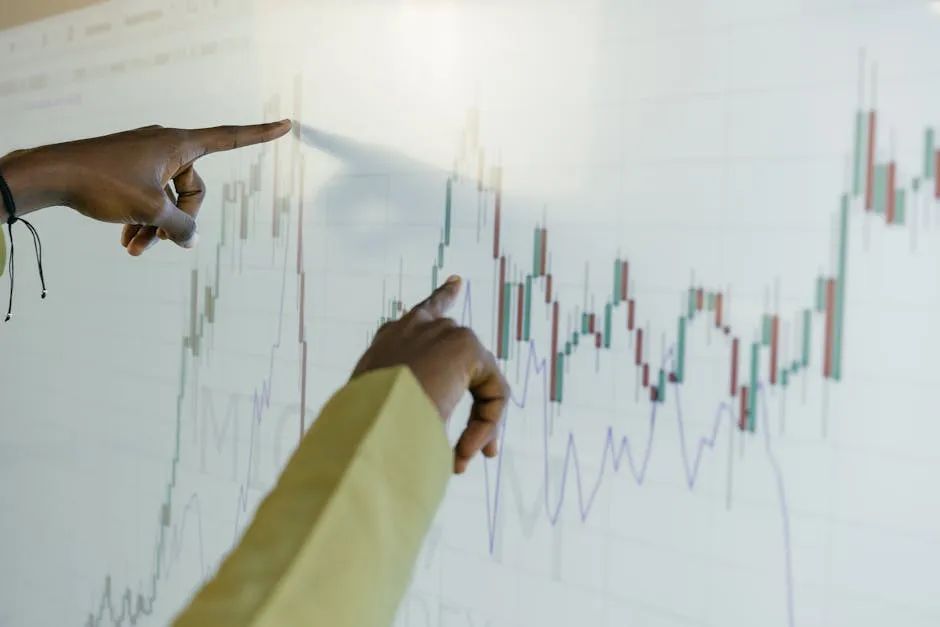
If you want to avoid pitfalls like these, consider reading “Statistics Done Wrong: The Woefully Complete Guide” by Alex Reinhart. This book is a hilarious yet informative exploration of common statistical mistakes and how to avoid them.
Lack of Contextual Understanding
Inferential statistics can sometimes feel like a game of telephone. As researchers pass data through various statistical tests, the unique context of the data often gets lost in translation. This oversight can lead to misguided conclusions that don’t accurately reflect the underlying realities.
Take, for example, a study evaluating the effectiveness of a new educational program. The statistical analysis might show a significant increase in test scores. But without considering the students’ backgrounds, classroom environments, and teaching methods, the results can be misleading. Did the program truly enhance learning, or were the improvements merely the result of other factors? Context is key, and inferential statistics often lack this critical element.
Domain knowledge plays a vital role in interpreting data. Researchers need to be well-versed in the subject matter to understand the nuances behind the numbers. If they aren’t, they risk drawing conclusions that are not only incorrect but can also have significant repercussions. For instance, a public health study might suggest a correlation between a specific diet and reduced disease rates. However, without understanding the broader social and economic factors at play, such conclusions could lead to misguided public health policies.
In conclusion, a lack of contextual understanding can severely limit the effectiveness of inferential statistics. Researchers must integrate domain knowledge into their analyses to ensure that their interpretations are accurate and meaningful. Only then can we harness the true power of statistics to inform decisions and drive progress.

For a deeper dive into understanding context in statistics, you might find “The Signal and the Noise: Why Most Predictions Fail but Some Don’t” by Nate Silver to be enlightening.
The Crisis of Replicability
The replicability crisis is a hot topic in the scientific community. It refers to the alarming trend where many studies fail to produce the same results upon retesting. Like a bad magic trick, the illusion of reliability fades away when scrutinized. Inferential statistics, particularly p-values, play a significant role in this crisis.
Researchers often rely on p-values to determine if their results are statistically significant. However, this reliance can be misleading. A classic case is the infamous OPERA experiment, which claimed neutrinos traveled faster than light. With a p-value of 0.0000003, it seemed groundbreaking. But hold your applause! A loose cable was later found to be the culprit behind the erroneous findings. The p-value was technically correct, but the underlying model fell apart due to faulty assumptions.
Another notable example is the study on antidepressants. Research suggested that these drugs were significantly more effective than placebos. However, when independent teams attempted to replicate the findings, they often fell short. The original studies’ statistical models failed to account for biases and confounding variables, leading to overinflated p-values and results that couldn’t be reproduced.
This crisis raises questions about the validity of research findings. If scientists cannot reproduce results, how can we trust them? The over-reliance on inferential statistics, particularly flawed models, contributes to this uncertainty. Researchers must approach their statistical analyses with caution and a critical eye, ensuring that they understand the implications of their methods and the assumptions they make.
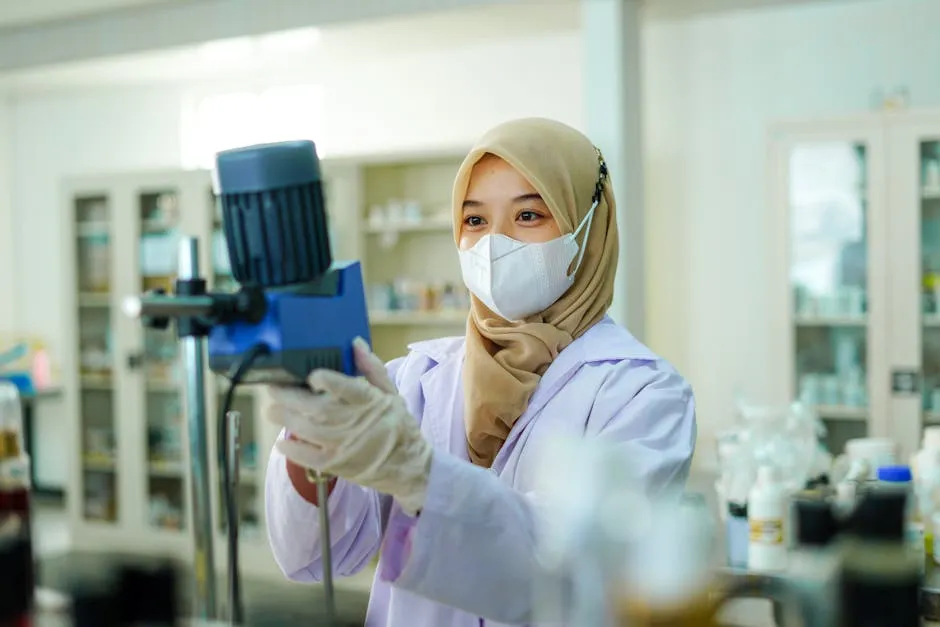
For those interested in exploring how to measure intangibles in business, “How to Measure Anything: Finding the Value of ‘Intangibles’ in Business” by Douglas W. Hubbard is a highly recommended read!
Alternative Approaches to Statistical Inference
As the shortcomings of inferential statistics become clearer, researchers are exploring alternative approaches. Bayesian statistics, for instance, offers a fresh perspective. Unlike traditional methods, it allows for the incorporation of prior knowledge into analyses, making it more flexible and context-sensitive. This is like having a seasoned detective on a case, using past experiences to inform current investigations.
Descriptive statistics also deserve a shout-out! By providing a clear snapshot of the data without making inferences, they can help researchers better understand their findings. Visualizations like histograms and box plots can reveal patterns that could be overlooked in inferential analyses. It’s like reading the room before making a bold statement. Understanding descriptive statistics in manufacturing plant can provide a solid foundation for data analysis.
Machine learning techniques are another exciting avenue to explore. They can analyze large datasets, identify patterns, and make predictions without relying heavily on traditional inferential methods. This is particularly useful in fields like healthcare, where complex interactions among variables can be challenging to navigate.
In summary, embracing these alternative approaches can lead to more accurate and context-sensitive insights. As researchers turn their backs on the pitfalls of inferential statistics, they open the door to a world of possibilities that prioritize understanding over mere numbers. The goal should always be clarity, accuracy, and ultimately, better decisions based on data.
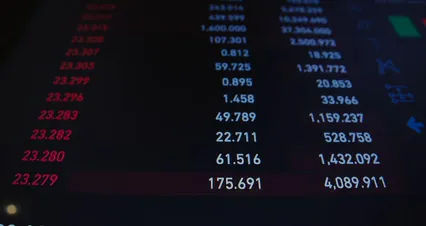
For those interested in a practical guide to statistical concepts, “Practical Statistics for Data Scientists: 50 Essential Concepts” by Peter Bruce and Andrew Bruce is a must-read!
Improving Statistical Literacy
Statistical literacy is crucial for researchers and students alike. It can feel like trying to read a foreign language without a dictionary. So, how do we enhance this understanding?
First, we need more engaging educational resources. Workshops and online courses that incorporate real-world examples can make statistics feel less daunting. Imagine a world where students learn statistical concepts through interactive simulations instead of dry lectures! This approach not only ignites curiosity but also solidifies understanding.
Second, integrating statistical literacy into various disciplines is vital. For instance, a biology course that emphasizes data analysis will empower future scientists. They’ll learn to appreciate the nuances of inferential statistics while studying their subject matter.
Lastly, mentorship plays a pivotal role. Pairing students with experienced researchers can provide invaluable insights. Students learn not just the “how” but also the “why” behind statistical methods. This mentorship can bridge the gap between theoretical knowledge and practical application.

Emphasizing Descriptive Statistics
Descriptive statistics deserve more attention! While inferential statistics often steal the show, descriptive stats provide essential groundwork. They summarize and present data in a digestible format, offering clarity before jumping into complex analyses.
Take a survey of students’ favorite pizza toppings. Descriptive statistics can show that 40% prefer pepperoni, while 30% go for vegetarian. This simple breakdown provides immediate insights without the complications of inferential methods.
Moreover, descriptive statistics can highlight trends over time. For example, tracking annual sales data visually can show peaks and troughs, making it easier to spot patterns. This kind of clarity helps researchers and decision-makers grasp the situation without getting lost in the numbers.
By focusing on descriptive statistics, we can build a strong foundation for further analysis. It’s like mastering the scales before playing a complicated piano piece.
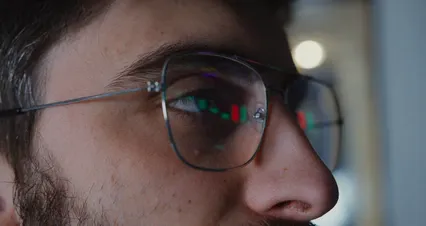
If you’re interested in digging deeper into statistical methods, consider “Statistical Inference” by George Casella and Roger L. Berger. This book offers a detailed look into the foundations of statistical theory!
Encouraging Transparency and Rigor
Transparency is key in research. When researchers share their methodologies and data sources, it fosters trust. Think of it like a recipe: if you know the ingredients and steps, you can replicate the dish.
Encouraging researchers to disclose their methods is essential for progress. It helps others understand the context of the results and allows for better evaluation. Moreover, when findings can be replicated, the scientific community strengthens its credibility.
To promote transparency, journals should implement stricter guidelines. Requiring detailed methodology sections will ensure that researchers provide sufficient information. This practice not only aids in replication but also enhances the overall quality of research.
In summary, transparency and rigor in statistical reporting can lead to better understanding and trust in research findings. It’s a win-win situation for everyone involved in the scientific process.
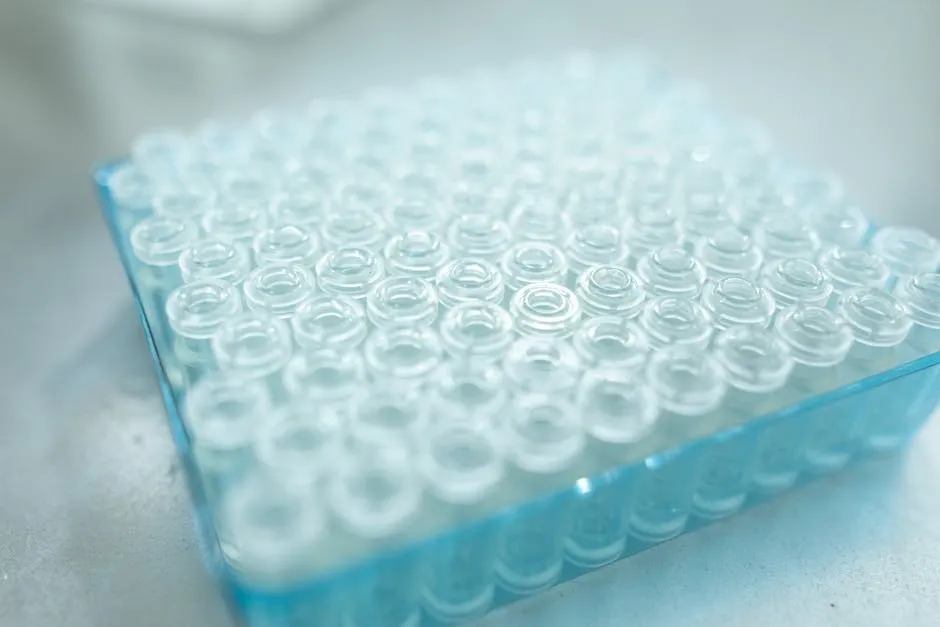
Please let us know what you think about our content by leaving a comment down below!
Thank you for reading till here 🙂
All images from Pexels