Introduction
In the world of healthcare analytics, the devil is truly in the details, especially when interpreting data. Class boundaries are the unsung heroes that help healthcare professionals make sense of complex datasets. Imagine trying to classify patient data or treatment outcomes without clear intervals—chaos! This blog post will guide you through the top methods for calculating class boundaries, ensuring your statistical analyses are as precise as a surgeon’s scalpel.
To get started on your journey into the data-driven world of healthcare, consider picking up Statistics for Healthcare Professionals by David H. Kaye. This book is a fantastic resource that will help you navigate through the statistical methods crucial for healthcare analytics. It’s like having a trusted guide in a data jungle!
Understanding the methods for calculating class boundaries is essential for accurate data interpretation in healthcare analytics. top methods for calculating class boundaries
Class boundaries define the values that separate different classes in a frequency distribution. They allow us to create meaningful categories for analysis, transforming raw data into actionable insights. Without effective class boundaries, interpreting patient records or treatment efficacy becomes a Herculean task.
The importance of class boundaries cannot be overstated. They provide clarity in data representation and eliminate ambiguity. This becomes critical in healthcare, where decisions can significantly impact patient outcomes. For instance, when classifying cholesterol levels, clear boundaries help in determining risk categories, allowing for better clinical decisions.

If you’re looking to enhance your data visualization skills, check out Data Visualization: A Practical Introduction by Kieran Healy. This book will help you turn those numbers into engaging visuals, making your data presentations pop like a confetti cannon at a birthday party!
With advancements in healthcare technology and data collection, the amount of data available for analysis has skyrocketed. Consequently, the need for robust statistical methods to interpret this data has become increasingly vital. From understanding the basics to applying advanced techniques, we’ll explore various methods for calculating class boundaries.
Whether you’re a seasoned statistician or a healthcare professional venturing into data analysis, mastering these techniques will enhance your analytical toolkit. So, buckle up as we embark on this enlightening journey into the world of class boundaries! Prepare to equip yourself with the knowledge that will empower your statistical analyses and help improve healthcare outcomes.
Let’s dive into the top methods for calculating class boundaries, ensuring that your analyses are sharp, insightful, and ready to make a difference in healthcare analytics.
Understanding Class Boundaries
What Are Class Boundaries?
Class boundaries are the invisible lines that separate different classes in a frequency distribution. Imagine a classroom where every student is trying to make sense of their grades. Class boundaries help define the limits of what counts as an A, B, or C, ensuring there’s no confusion about where one grade ends and another begins.
In statistics, class boundaries are crucial for accurately representing data. They differ from class limits—class limits define the maximum and minimum values within a class, while class boundaries refine these limits to eliminate any overlap. For instance, if one class ends at 30 and the next starts at 31, the class boundary between them would be 30.5. This distinction allows for precise categorization, especially when dealing with continuous data where values can fall anywhere within the range.
Understanding class boundaries is particularly significant in healthcare analytics. Healthcare professionals rely on accurate data interpretation to make informed decisions. Whether classifying patient risk levels or analyzing treatment outcomes, class boundaries provide clear intervals. They ensure that healthcare data is not just numbers on a page, but a roadmap guiding practitioners toward better patient care.
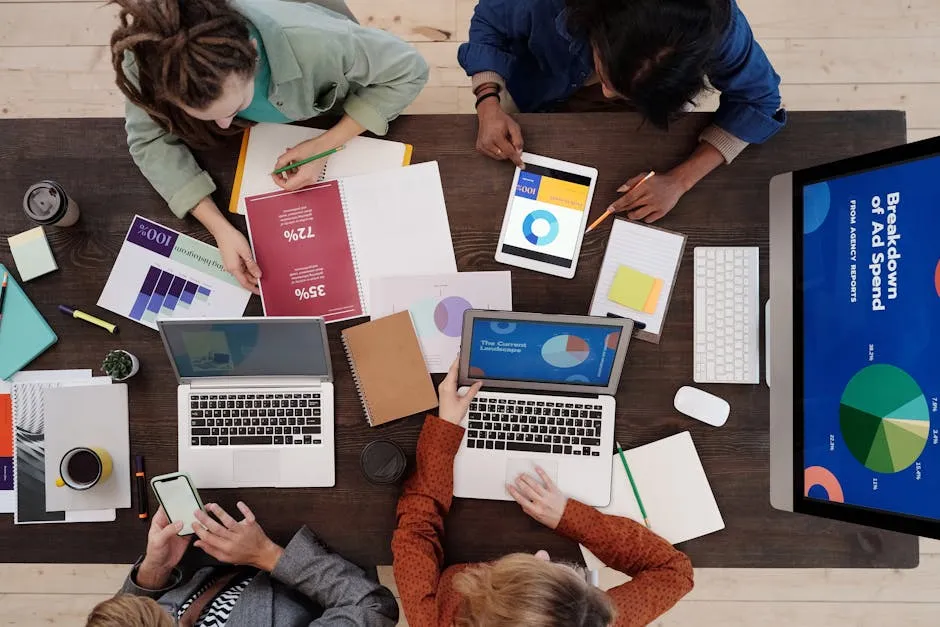
In the realm of continuous data, you might find yourself needing tools like a Blood Pressure Monitor. Keeping track of blood pressure readings can help you establish class boundaries effectively, ensuring you never miss a beat (pun intended!) when it comes to patient care.
Importance in Healthcare Analytics
Class boundaries play a pivotal role in data interpretation. In healthcare, the implications of decisions based on data can be life-altering. For instance, when analyzing patient cholesterol levels, class boundaries help categorize individuals as low, moderate, or high risk. This classification directly influences treatment plans and patient counseling, ultimately improving health outcomes.
Consider a case study involving a hospital’s patient monitoring system. By establishing clear class boundaries for various health metrics, staff can quickly identify patients needing immediate intervention. In one instance, a sudden spike in blood pressure readings led to timely medical responses, demonstrating how effective data categorization directly impacts patient safety.

If you’re a healthcare professional looking to upgrade your skills, take a look at Healthcare Analytics: From Data to Knowledge to Healthcare Improvement by Hui Yang. This book provides insights into transforming data into actionable healthcare strategies that can save lives!
Moreover, class boundaries facilitate clear communication of data insights among healthcare teams. When presenting statistical findings during meetings, well-defined class boundaries enable healthcare professionals to convey important information succinctly. Instead of getting lost in technical jargon, teams can focus on actionable insights, ensuring everyone is on the same page. This clarity can enhance collaboration and decision-making processes, ultimately benefiting patient care.
In summary, class boundaries are not merely statistical constructs; they are essential tools in healthcare analytics. They enhance data interpretation, support informed decision-making, and foster teamwork in clinical settings. By mastering the calculation and application of class boundaries, healthcare professionals can significantly improve their analytical capabilities, leading to better patient outcomes and more effective healthcare strategies.
Method 3: Adjusting for Continuous Data
Importance
Continuous data is the lifeblood of healthcare analytics. It requires special attention because it can take on any value within a range. If we treat this data like categorical data, we risk misinterpretation. For instance, consider blood pressure readings. A reading of 120.5 mmHg clearly differs from 120.8 mmHg. Classifying them inaccurately could lead to misguided treatment decisions. Thus, adjusting class boundaries for continuous data ensures precision and clarity, ultimately improving patient outcomes.
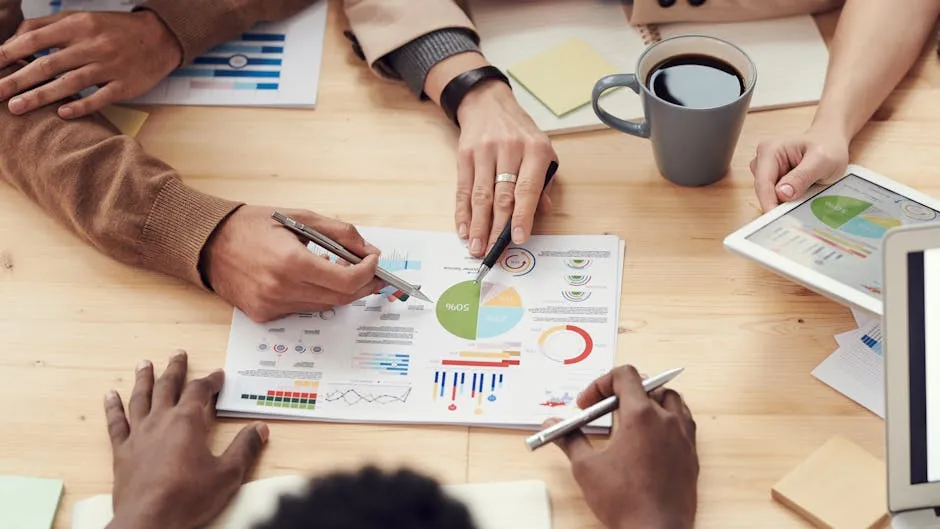
Steps to Adjust Boundaries
Let’s walk through the adjustment process. Suppose we have patient cholesterol levels measured in mg/dL. The challenge is to categorize these levels effectively.
- Identify Class Limits: Start with your data, say 200-239 mg/dL for borderline high and 240-300 mg/dL for high cholesterol.
- Calculate Differences: The upper limit of the first class (239) and the lower limit of the next class (240) show a difference of 1 mg/dL.
- Find Midpoint: Divide this difference by 2, giving us 0.5.
- Adjust Class Boundaries: Now, subtract 0.5 from the lower limit of the first class and add it to the upper limit of the second class. So, the new boundaries become:
- For the first class: Lower boundary = 199.5 mg/dL, Upper boundary = 239.5 mg/dL.
- For the second class: Lower boundary = 239.5 mg/dL, Upper boundary = 300.5 mg/dL.
This adjustment ensures that there’s no overlap between classes, allowing for precise categorization of patients based on their cholesterol levels.
Common Errors
While adjusting class boundaries, several pitfalls can arise. One common error is failing to account for decimal values. If you neglect to adjust for a continuous scale, you may end up with boundaries that misrepresent the data. For example, rounding off too early can lead to significant data loss.
Another issue is using overly broad classes. If the classes are too wide, important variations within the data might be obscured. This is particularly detrimental in healthcare, where small differences can lead to entirely different treatment protocols. To avoid these pitfalls, always ensure that your boundaries reflect the true nature of the data.
With these adjustments, healthcare professionals can elevate their data interpretation skills, leading to more informed decisions that benefit patient care.
Challenges in Calculating Class Boundaries
Statistics can sometimes feel like a game of Jenga—one wrong move, and the whole thing can come crashing down! This is especially true when calculating class boundaries in healthcare analytics. Class boundaries are crucial for ensuring accurate data interpretation. However, they come with their own set of challenges. Let’s take a closer look.
Common Pitfalls
Misinterpretation of data often leads to headaches. When healthcare professionals misread class limits, they risk drawing incorrect conclusions. For example, if a class limit is set too broadly, it can obscure meaningful differences in patient outcomes. This confusion can lead to misguided treatment protocols, which is the last thing we want!
Overlapping boundaries are another sneaky problem. Imagine two classes where the upper limit of one overlaps with the lower limit of another. This can create chaos in data analysis. For instance, if one class is 30-40 and the next is 40-50, we’re left scratching our heads about where the number 40 belongs. This overlap can distort results and mislead clinicians, ultimately affecting patient care.
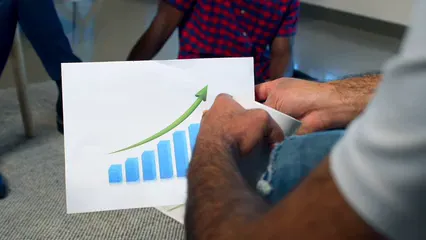
Want to monitor your patients’ health more effectively? Consider investing in a Fitbit Charge 5. This nifty gadget not only tracks physical activity but also monitors heart rate, sleep patterns, and even stress levels. It’s like having a personal health coach right on your wrist!
Solutions
Avoiding these pitfalls requires a proactive approach. First, ensure a clear understanding of class limits. A good rule of thumb is to double-check your intervals. Using precise decimal adjustments can help eliminate overlap. For instance, if your first class ends at 30, set the next class to start at 30.1, eliminating confusion.
Another effective strategy is to use visual aids, like histograms. These can help you see the distribution of your data at a glance. If classes overlap, it will be glaringly obvious! Regularly revisiting your data analysis process is also beneficial. Take a moment to reflect on the boundaries you’ve set. Are they still relevant? Taking time to reassess can help catch errors before they lead to misinterpretation.
In summary, navigating the challenges of calculating class boundaries is essential for accurate data analysis in healthcare. By remaining vigilant and using visual tools, healthcare professionals can avoid common pitfalls and improve their data interpretation skills. After all, making informed decisions based on solid data can lead to better patient outcomes.
Conclusion
In conclusion, understanding and applying the correct methods for calculating class boundaries is crucial for healthcare analytics. They not only facilitate accurate data interpretation but also enhance decision-making processes that can lead to improved patient outcomes. By mastering these techniques, healthcare professionals can ensure that their analyses are precise and actionable, ultimately driving better health strategies.
Class boundaries are more than just numbers; they are the framework that supports data integrity and clarity. When these boundaries are correctly established, they help categorize data into meaningful segments. This is particularly important in healthcare, where every detail can significantly impact a patient’s treatment and care.
As you continue to explore the intricacies of statistical analysis, remember that class boundaries are your allies in navigating the complexities of healthcare data. They help transform raw numbers into actionable insights that can help providers make informed decisions. By ensuring that class boundaries are well-defined, healthcare professionals can enhance their ability to communicate findings effectively, collaborate with their teams, and drive improved patient outcomes.
In a field where precision is paramount, understanding how to calculate and apply class boundaries will elevate your data analysis skills. So, let’s embrace the power of well-defined class boundaries and strive for excellence in healthcare analytics!
FAQs
What exactly are class boundaries, and why are they important?
Class boundaries are the critical lines that separate different classes in a frequency distribution. Think of them as the invisible fences that define the limits for data categorization. This distinction is crucial in statistics, particularly in healthcare analytics, where accurate data interpretation can directly impact patient outcomes. For instance, when recording cholesterol levels, class boundaries ensure that patients are classified correctly into categories like low, moderate, or high risk. By clearly defining these intervals, healthcare professionals can make informed decisions that enhance patient care.
How do you calculate class boundaries for continuous data?
Calculating class boundaries for continuous data involves a few straightforward steps. Let’s illustrate this with an example: 1. Identify Class Limits: Suppose we have two classes: 30-40 and 40-50. 2. Calculate the Difference: The upper limit of the first class (40) and the lower limit of the second class (40) yield a difference of 0. 3. Find the Midpoint: Divide this difference by 2. In this case, 0/2 = 0. 4. Adjust Class Boundaries: For the first class: Lower boundary = 29.5, Upper boundary = 40.5. For the second class: Lower boundary = 40.5, Upper boundary = 49.5. This adjustment ensures that there’s clear separation between classes, particularly important for continuous healthcare data like blood pressure or glucose levels.
What challenges might I face when calculating class boundaries?
Calculating class boundaries can sometimes feel like trying to solve a Rubik’s Cube blindfolded. One common challenge is misinterpreting data, which can lead to incorrect class limits. If classes overlap, it can create confusion. For example, if one class is defined as 30-40 and the next as 40-50, where does the number 40 go? It’s crucial to clearly define boundaries to avoid this overlap. Another challenge is handling continuous data accurately. If you round values too early, important distinctions might be lost. To tackle these issues, always verify that class limits are precise and utilize visual aids like histograms to identify overlaps at a glance.
Can class boundaries be applied to categorical data?
Class boundaries primarily cater to continuous data, but that doesn’t mean categorical data is left out in the cold! While categorical data doesn’t require the same boundary adjustments, understanding the limits is still useful. Class boundaries can help define categories in instances like patient demographics or treatment types. However, since categories are distinct and non-overlapping, the concept of boundaries is less critical here. Just remember, while boundaries are vital for continuous data, they serve a different purpose for categorical data.
What tools can assist in calculating class boundaries?
Healthcare analysts have a plethora of tools at their disposal! Software like Excel or R can facilitate class boundary calculations with built-in functions. For those who enjoy a more visual approach, data visualization tools like Tableau or Power BI can help create histograms, making class boundaries easier to identify. Additionally, online calculators designed for statistical analysis can simplify the process. By leveraging these resources, healthcare professionals can ensure their analyses are accurate and insightful, ultimately improving patient care decisions.
Please let us know what you think about our content by leaving a comment down below!
Thank you for reading till here 🙂
All images from Pexels