Introduction
Average Variance Extracted (AVE) is vital in validating measurement models. It helps researchers assess convergent validity, ensuring that items reflect the intended construct. AVE plays a key role in structural equation modeling (SEM), providing insights into the quality of latent variables.
If you’re diving into the world of research and need a solid foundation, check out The Researcher’s Toolkit: A Guide to Measurement and Evaluation. This book is like having a Swiss Army knife for your research needs—everything you need to measure and evaluate with precision.
Summary and Overview
Average Variance Extracted (AVE) measures the quality of latent constructs by evaluating how much variance in observed variables is accounted for by the construct. A higher AVE indicates better convergent validity and reliability. Typically, an AVE value above 0.5 is considered acceptable, suggesting that more than half of the variance is explained by the construct. In this article, we will cover AVE’s definition, its relationship with convergent validity and reliability, calculation methods, thresholds, and best practices for researchers.
Need a practical guide to statistics that simplifies data analysis? Statistics for Research: A Practical Guide to Data Analysis is your answer! It demystifies the statistical methods you need, making your research journey smoother and more enjoyable.
Understanding Average Variance Extracted
What is Average Variance Extracted?
Average Variance Extracted (AVE) quantifies how much variance in a set of indicators is captured by a latent variable. The formula for calculating AVE is:
AVE = (Σ λi2) / (Σ λi2 + Σ Var(ei))
Here, λi represents the factor loadings of the indicators, and Var(ei) reflects the error variance. AVE is significant in research methodology because it helps ensure that the latent construct adequately explains the indicators. A higher AVE value indicates a stronger relationship between the construct and its indicators, enhancing the overall validity of the measurement model. As researchers, we should always strive for an AVE greater than 0.5 to confirm robustness in our constructs.
If you’re looking to deepen your understanding of structural equation modeling, consider picking up Introduction to Structural Equation Modeling. It’s a fantastic resource that will guide you through the complexities of SEM with ease and clarity.
The Role of AVE in Convergent Validity
Convergent validity is essential for ensuring that a measurement model accurately captures what it intends to measure. Average Variance Extracted (AVE) serves as a key metric in this assessment. It indicates how much variance in indicators is accounted for by a latent construct.
When evaluating measurement models, researchers often compare AVE values across different studies. A common benchmark is an AVE value above 0.5, suggesting that the latent variable explains more than half of the variance in its indicators. For example, a study on perceived quality in education found AVE values ranging from 0.512 to 0.745 for various constructs. These findings reinforce the importance of achieving sufficient AVE for robust convergent validity.
AVE also relates closely to other validity measures, such as construct reliability. High AVE values usually correlate with strong construct reliability, creating a well-rounded framework for assessing measurement quality.
How well does your research design address convergent validity? Consider exploring more about reliability growth analysis RGA to refine your approach.
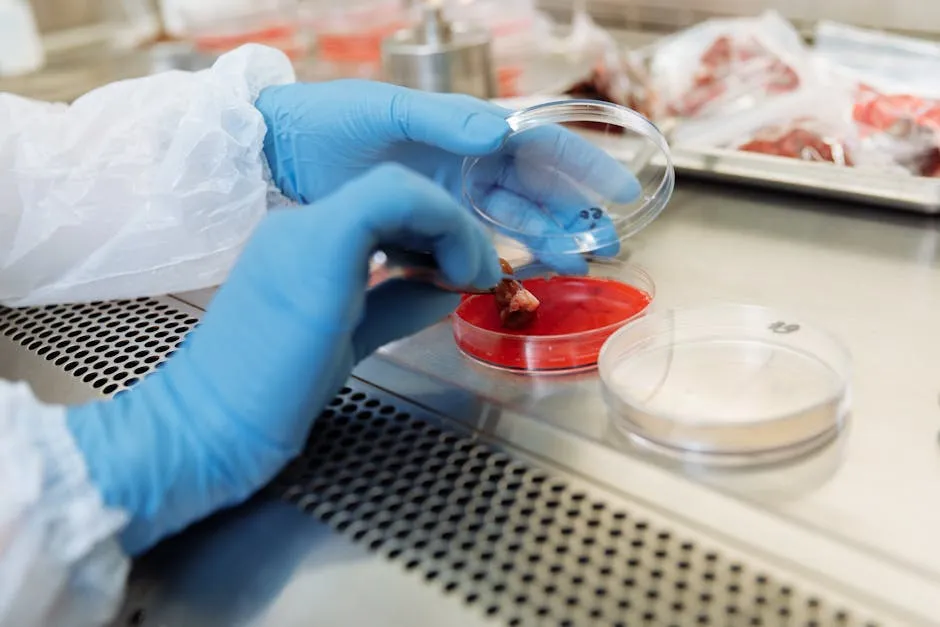
AVE and Its Thresholds
Acceptable Values for AVE
Average Variance Extracted (AVE) values are crucial for assessing the quality of measurement models. Generally, an AVE value of 0.5 or higher is considered acceptable. This threshold indicates that over half of the variance in the observed variables is accounted for by the latent construct. For example, in a study measuring perceived quality in education, constructs showed AVE values ranging from 0.512 to 0.745. Here, both values confirm adequate measurement quality.
Conversely, an AVE below 0.5 suggests that the items explain more error variance than actual variance. This is problematic for research validity. For instance, if a construct yields an AVE of 0.45, it raises concerns about its reliability. Researchers should ensure their constructs meet or exceed the 0.5 threshold to maintain statistical significance and robust findings.
Have you encountered AVE issues in your studies? Share your experiences in the comments!
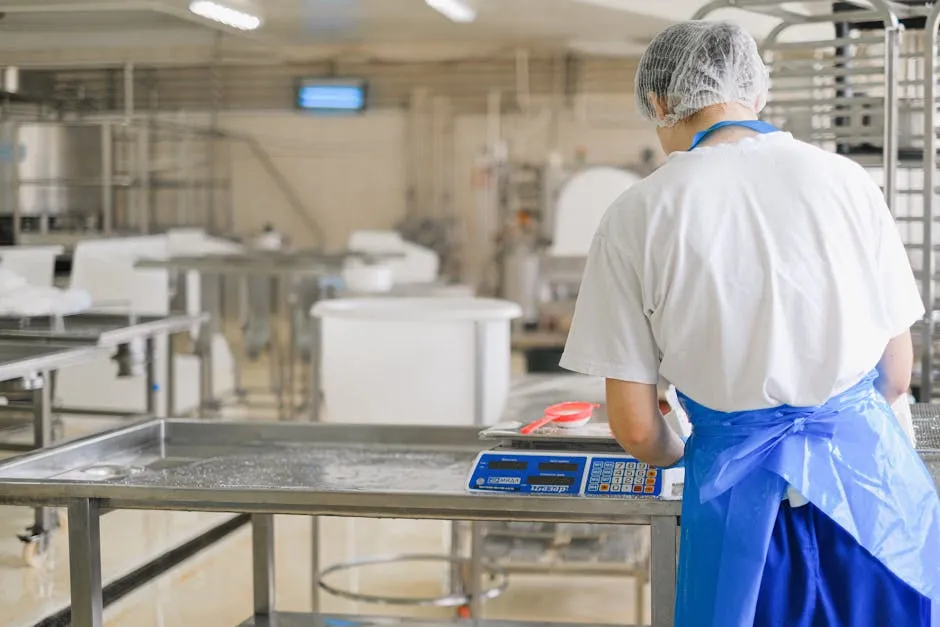
Relationship Between AVE and Other Metrics
Comparison with Cronbach’s Alpha
Average Variance Extracted (AVE) and Cronbach’s Alpha are both vital in evaluating measurement quality. While AVE focuses on convergent validity, Cronbach’s Alpha assesses internal consistency. High AVE values indicate that a latent construct explains a significant amount of variance in its indicators. Ideally, an AVE of 0.5 or greater aligns with a Cronbach’s Alpha of 0.7 or higher, signaling a reliable measurement scale.
However, they serve different purposes. Use AVE when validating the construct’s ability to explain variance. Meanwhile, rely on Cronbach’s Alpha to confirm that items consistently measure the same construct. For example, a study might report an AVE of 0.68 alongside a Cronbach’s Alpha of 0.85, showcasing both strong convergent validity and internal consistency.
Have you applied these metrics in your research? Share your experiences with reliability testing below!
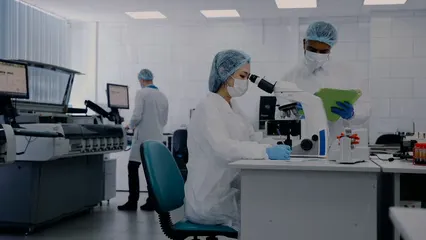
Impact of Low AVE Values
What Happens When AVE is Below 0.5?
When Average Variance Extracted (AVE) falls below 0.5, it raises red flags for research findings. This indicates that the measurement model explains more error than true variance. Consequently, the construct’s validity may be compromised. For example, a study on customer satisfaction reported an AVE of 0.45. This low value suggests that the items used to measure satisfaction may not accurately reflect the underlying construct.
To improve AVE in future studies, researchers can take several steps. First, reassess the indicators used in the measurement model. Are they truly representative of the construct? Second, consider increasing the number of indicators. Having more items can provide a more comprehensive view of the construct. Finally, examine the factor loadings closely. Items with low loadings should be revised or removed to enhance the overall model.
For those interested in improving their data analysis skills, I highly recommend Discovering Statistics Using IBM SPSS Statistics. It’s a delightful read that makes statistics fun and accessible!
Have you checked your studies for AVE issues? It’s crucial to ensure your research findings are robust and valid.
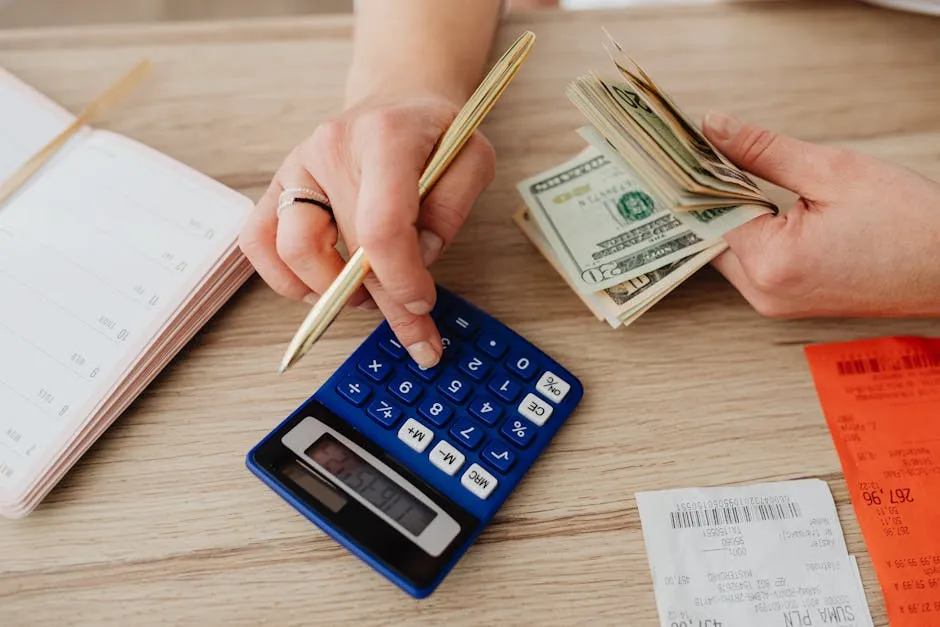
Best Practices for Using AVE
Recommendations for Researchers
When calculating and interpreting AVE, following best practices is essential. Start by ensuring that all indicators relate closely to the intended construct. Each item’s factor loading should ideally exceed 0.5. This indicates a strong relationship with the latent variable. Additionally, aim for an AVE greater than 0.5 to affirm convergent validity.
Common mistakes include overlooking the importance of item selection. Always review whether each item accurately captures the construct. Avoid using too few indicators, as this can skew results. A checklist can help ensure thorough assessments of AVE. Consider including factors like appropriate sample size, correct factor analyses, and clear documentation of findings.
For a handy resource, download our checklist for AVE best practices. This tool will guide you in maintaining research integrity and improving measurement practices. Together, we can enhance the quality of research outputs!
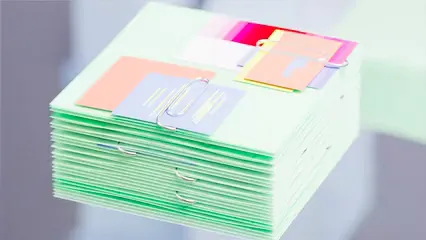
Speaking of resources, if you want to explore multivariate statistical analysis, grab a copy of Applied Multivariate Statistical Analysis. It’s a great addition to your research library!
Conclusion
In summary, Average Variance Extracted (AVE) is crucial for validating measurement models. It helps ensure that constructs accurately represent the underlying variables. Aiming for an AVE above 0.5 confirms strong convergent validity. This metric is vital for enhancing the overall rigor and credibility of your research findings. By applying what you’ve learned about AVE, you can improve the quality of your own research. Remember, a solid understanding of AVE can significantly impact the integrity of your work and your conclusions. Don’t hesitate to incorporate this knowledge into your future studies!
Before we wrap up, if you find yourself in need of a reliable data analysis software, consider R for Data Science: Import, Tidy, Transform, Visualize, and Model Data. It’s a fantastic tool that will help streamline your data analysis process!
FAQs
What is Average Variance Extracted (AVE) and why is it important?
Average Variance Extracted (AVE) is a measure used to assess convergent validity in research. It indicates how much variance in observed variables is accounted for by a latent construct. A high AVE value suggests that the construct effectively represents its indicators, enhancing the validity of the measurement model.
How is AVE calculated?
To calculate AVE, use the formula: AVE = (Σ λi2) / (Σ λi2 + Σ Var(ei)) Here, λi represents factor loadings of the indicators, and Var(ei) reflects error variance. Collect the necessary data from your analysis to apply this formula.
What does a low AVE value indicate?
A low AVE value, typically below 0.5, suggests that the items in a measurement model explain more error variance than actual construct variance. This can compromise the validity and reliability of your research findings, indicating the need for model improvement.
Can AVE be used alongside Cronbach’s Alpha?
Yes, AVE can be used alongside Cronbach’s Alpha. While AVE assesses convergent validity, Cronbach’s Alpha evaluates internal consistency. Together, they provide a comprehensive picture of measurement quality, ensuring that constructs are both reliable and valid.
What are the best practices for ensuring adequate AVE in research?
To ensure adequate AVE, focus on several best practices: – Select strong indicators that accurately reflect the construct. – Aim for an AVE greater than 0.5. – Reassess low-loading items and consider adding more indicators. – Conduct thorough exploratory and confirmatory factor analyses to validate your model.
Please let us know what you think about our content by leaving a comment down below!
Thank you for reading till here 🙂
All images from Pexels