Introduction
Post hoc statistical power analysis might sound like a fancy term that only statisticians care about. But hold onto your calculators! This concept plays a significant role in research and statistical analysis. Simply put, post hoc power measures the likelihood of detecting an effect from data that has already been collected. It’s like trying to guess the answer to a pop quiz after seeing the questions.
However, the use of post hoc power analysis is controversial. Some researchers believe it helps make sense of non-significant results, while others argue it’s misleading. Critics contend that it does little more than rehash the p-value, making it seem like researchers are grasping at straws to justify their findings. This article aims to clarify the nature of post hoc statistical power, its relevance, and the ongoing debates within the research community.
So, why should you care? Understanding post hoc power can help you interpret research more critically. It can also guide you in designing better studies. And who wouldn’t want to be the smartest cookie in the lab? Let’s break down what statistical power really means and why it matters in hypothesis testing and research conclusions.
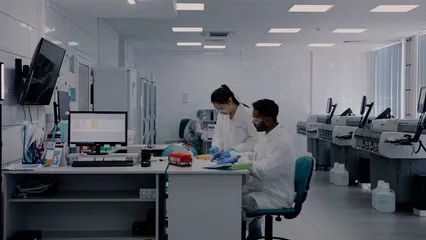
Understanding post hoc power can help you interpret research more critically. It can also guide you in designing better studies. hypothesis testing is a crucial aspect to consider.
1. What is Statistical Power?
Statistical power is a key player in hypothesis testing. It represents the probability that a statistical test will correctly reject a null hypothesis when it is, in fact, false. In simpler terms, imagine you’re at a carnival trying to win a stuffed bear. If you have a good strategy (high power), you’re more likely to succeed. But if your strategy stinks (low power), you’ll likely walk away empty-handed.
Power is crucial because it relates directly to two types of errors: Type I and Type II errors. A Type I error occurs when you mistakenly reject a true null hypothesis (think of it as declaring a bear is yours when it’s not). On the flip side, a Type II error is when you fail to reject a false null hypothesis (like not trying for that bear when you could have won). The ideal scenario is high power, which reduces the chances of these errors.
But how do we measure power? It depends on several factors, including effect size, sample size, significance level (alpha), and variability. That’s right—just like a recipe, if you mess with the ingredients, the results may turn out differently! Increasing the sample size usually boosts power, giving you a better shot at detecting true effects. To dive deeper into these concepts, consider picking up Statistical Power Analysis: A Practical Guide for Researchers. It’s a great resource for understanding the ins and outs of power in research.
Understanding statistical power is essential for researchers. It guides study design and ensures that studies are adequately powered to detect meaningful effects. With low power, even the most well-designed studies can yield inconclusive results. So, before you hit “publish,” ensure your study has the power to back up your claims.
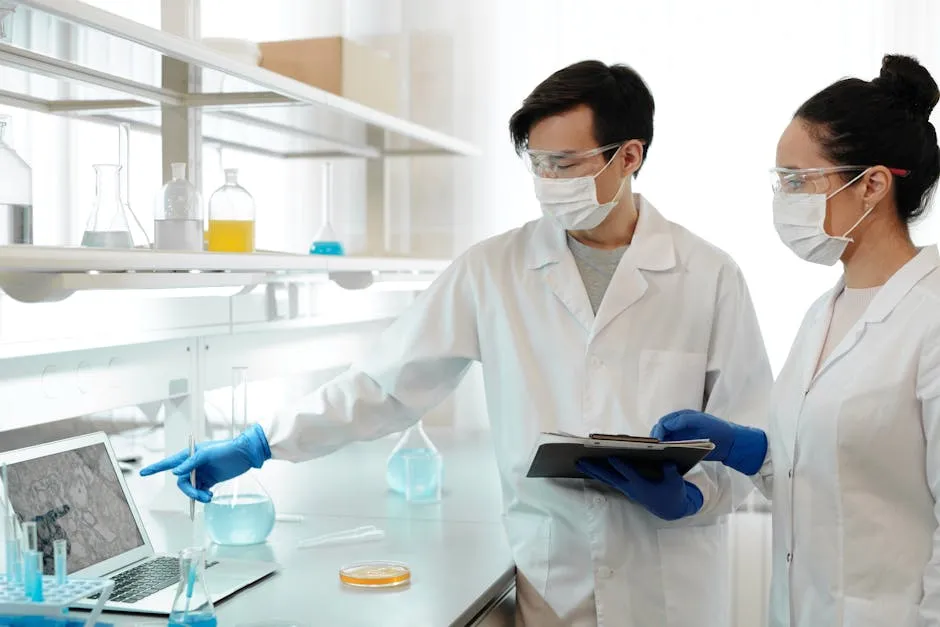
1.1 The Components of Statistical Power
When it comes to statistical power, several key components come into play. Think of them as the building blocks that determine whether your study can detect a meaningful effect.
First up is effect size. This refers to the magnitude of the difference you’re trying to measure. A larger effect size usually translates to higher power, as it’s easier to detect a substantial difference than a tiny one. If you want to learn more about effect sizes, check out The Elements of Statistical Learning by Trevor Hastie. It’s a foundational text that dives into statistical models and their applications.
Next, we have sample size. Larger sample sizes generally lead to higher power. Picture this: the more darts you throw at the board, the better your chances of hitting a bullseye. So, if you want to boost your chances, consider recruiting more participants.
The significance level, often set at 0.05, is another critical factor. This alpha level determines the threshold for rejecting the null hypothesis. If you lower it, you reduce the risk of Type I errors but may inadvertently decrease power. It’s a classic balancing act!
Lastly, variability—or standard deviation—matters too. High variability in your data can obscure true effects, reducing power. Think of it like trying to find that one shiny penny in a pile of gravel. The more scattered the gravel, the harder it is to spot the penny!
By understanding these components, researchers can optimize their study designs. High statistical power enhances the chances of detecting true effects, leading to more reliable conclusions. Remember, in the world of research, power is not just a buzzword; it’s a crucial element in making your findings meaningful!
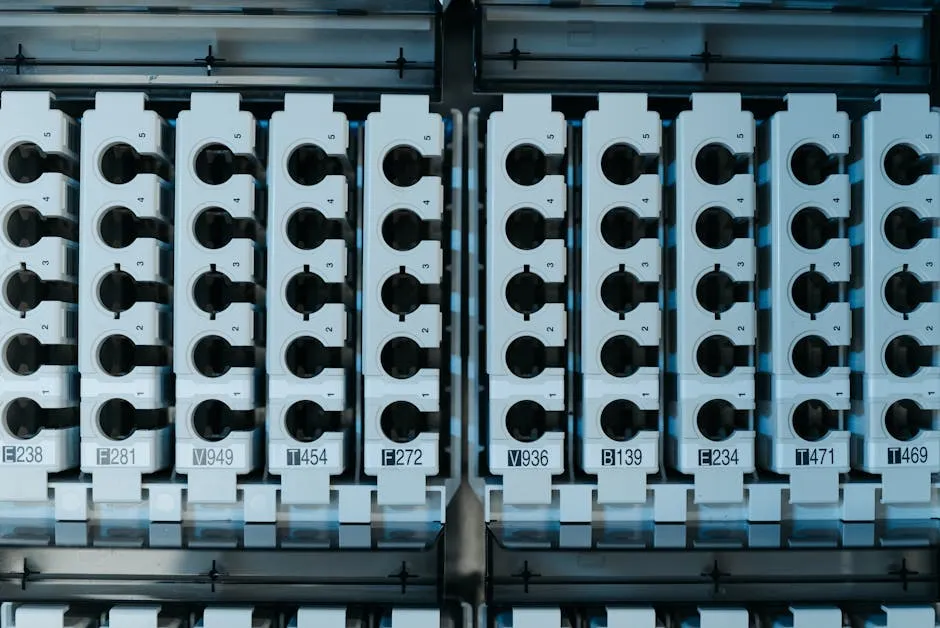
1.2 Importance of Prospective Power Analysis
When planning a study, prospective power analysis is a superhero in disguise. It swoops in to save the day by helping researchers determine the right sample size needed to detect an effect. Think of it as a crystal ball that reveals how likely a study is to yield significant results based on specific parameters. For a deeper understanding, you might want to check out The Art of Statistics: Learning from Data by David Spiegelhalter. It’s a great resource to help you navigate the complexities of data.
The importance of this analysis cannot be overstated. First, it ensures that studies are adequately powered. A well-powered study reduces the risk of Type II errors, which occur when researchers fail to reject a false null hypothesis. Imagine this scenario: you’ve developed a new drug, but your study is too small to detect its effectiveness. You might walk away thinking the drug doesn’t work, when in reality, it could have cured the common cold!
Prospective power analysis also plays a crucial role in determining sample sizes. Researchers can estimate how many participants are needed based on the anticipated effect size, significance level, and variability within the data. Without this analysis, studies might end up with too few participants, leading to inconclusive results. Nobody wants to end up like the kid who threw a birthday party but only invited two friends!
This analysis fosters better research practices and helps allocate resources efficiently. By ensuring the correct sample size, researchers can avoid wasting time and money on studies that are unlikely to yield meaningful results. It’s like going grocery shopping with a list instead of wandering around the store aimlessly—much more efficient!
Moreover, prospective power analysis enhances the credibility of research findings. Journals and reviewers often expect evidence of this analysis before publication. It demonstrates that researchers have thoughtfully designed their studies to maximize their chances of finding real effects. In a world where research reproducibility is under scrutiny, this is a step in the right direction.
Lastly, conducting prospective power analysis encourages a deeper understanding of the data and the underlying assumptions. It leads researchers to consider how different factors like effect size and variability could impact their results. This reflection can lead to more robust research questions and better study designs.
In summary, prospective power analysis is a vital tool in the research toolbox. It not only helps in determining appropriate sample sizes but also strengthens the overall quality and credibility of research. With this analysis in hand, researchers can confidently stride into their studies, knowing they have set themselves up for success.

2.2 The Statistical Relationship Between p-values and Post Hoc Power
When researchers conduct a statistical test, they often find themselves staring at a p-value, wondering what it all means. Enter post hoc power analysis, which claims to shed light on the power of a test after the data has been collected. However, the relationship between p-values and post hoc power is more about correlation than revelation. As Hoenig and Heisey (2001) brilliantly pointed out, post hoc power is a monotone function of the p-value, meaning it’s like trying to measure the temperature of soup by merely tasting a spoonful—it doesn’t give you anything more than what you already know.
Let’s break it down. A low p-value (less than 0.05) suggests a significant result, and voilà, your post hoc power calculation will likely show high power. Conversely, a high p-value hints at no significant effect, leading to a low post hoc power result. In essence, post hoc power is essentially a rehash of the p-value, leading many statisticians to question its utility. After all, if you’re merely confirming what you already suspected, why bother?
Imagine this scenario: you conduct an experiment and get a p-value of 0.07. You then perform a post hoc power analysis, which reveals a power of about 40%. You might think, “Aha! If only I had a larger sample size, I could’ve detected that elusive effect!” But hold your horses! That power calculation does not magically validate your findings. It simply mirrors the inadequacy of your p-value. Instead of providing clarity, it muddies the waters further.
Several studies have looked into this relationship. For instance, a paper by Zhang et al. (2019) examined the concept of post hoc power and found it lacking in providing any meaningful insights. They emphasized that power analysis is primarily a tool for prospective studies, not a retrospective tool to defend non-significant results. So, if you think post hoc power will bail you out of a tight spot, think again.
The take-home message? Don’t let post hoc power analysis distract you from the real story told by your p-value. It’s the classic case of “once bitten, twice shy.” Instead of focusing on post hoc power, consider using confidence intervals or Bayesian Data Analysis by Andrew Gelman for a more informative approach. They provide a fuller picture of your data, including estimated effect sizes and uncertainty, rather than just a binary “yes or no” outcome.
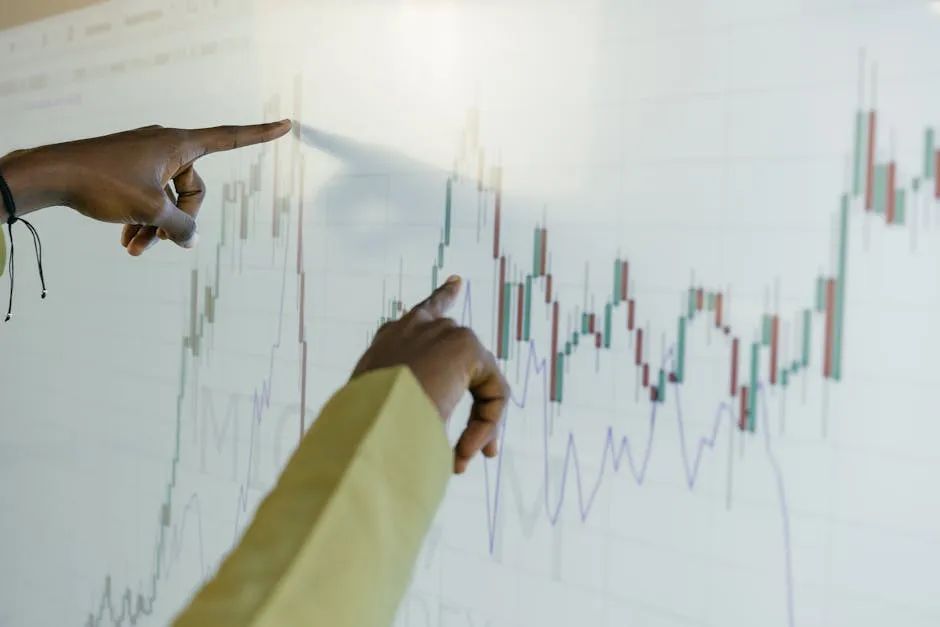
In conclusion, the relationship between p-values and post hoc power is undeniably critical but also somewhat misleading. The two are intertwined in a dance that offers little new information. Researchers should acknowledge this limitation and focus on more robust statistical methods that provide a clearer understanding of their findings. After all, it’s not just about having the right numbers; it’s about making sense of them in the grander scheme of research.
3.2 The “Type S” and “Type M” Errors
In the world of statistics, there’s more than just numbers and p-values. Enter the fascinating realm of Type S (sign) and Type M (magnitude) errors, two concepts that add a bit of spice to post hoc power analysis. These errors shine a spotlight on how we interpret our results, specifically focusing on the signs and sizes of effects we think we detect.
Let’s start with Type S errors. This error occurs when researchers mistakenly reverse the direction of an effect. Imagine you conduct an experiment to see if a new fertilizer boosts plant growth. The results show a significant decrease in growth! If you conclude that the fertilizer is harmful instead of beneficial, you’ve fallen into a Type S error. You’ve got the sign wrong, and now your garden might be suffering because of it.
Why does this happen? Often, it’s due to low statistical power. When a study lacks sufficient power, it can generate results that mislead researchers about the effect’s direction. This confusion can lead to erroneous conclusions and misguided recommendations. In fields where decisions hinge on these findings—like medicine or public policy—Type S errors can have significant implications.
Now, let’s move to Type M errors. Here, the focus shifts to the magnitude of the effect. Imagine you find a statistically significant increase in plant growth with that new fertilizer. Fantastic, right? But what if that increase is a mere 0.1 cm? That’s where the Type M error sneaks in, as it highlights the risk of overestimating the effect size. Researchers can mistakenly believe they have a substantial effect when, in reality, it’s practically negligible.
Type M errors often arise from small sample sizes and inflated p-values. When studies are underpowered, the estimated effect sizes can be exaggerated. This phenomenon is especially prevalent in post hoc power analyses, where researchers look at observed effects after the fact. The results can look impressive, but they often misrepresent the true nature of the effect.
So, how can researchers guard against these errors? First, understanding the limitations of post hoc power analysis is essential. It’s crucial to approach findings with a critical eye and consider the potential for Type S and Type M errors. Researchers should also prioritize prospective power analysis during study design to ensure that studies are adequately powered from the start. This planning can help avoid the pitfalls of misinterpreting results and provide a clearer picture of the effects being studied.
In summary, Type S and Type M errors serve as vital reminders for researchers. They underscore the need for careful interpretation of statistical results and the importance of robust study designs. Avoiding these errors helps ensure that conclusions drawn from research are not just statistically significant but also meaningful in real-world applications. After all, the ultimate goal is to use statistics not just to crunch numbers but to make informed decisions that positively impact our understanding of the world.
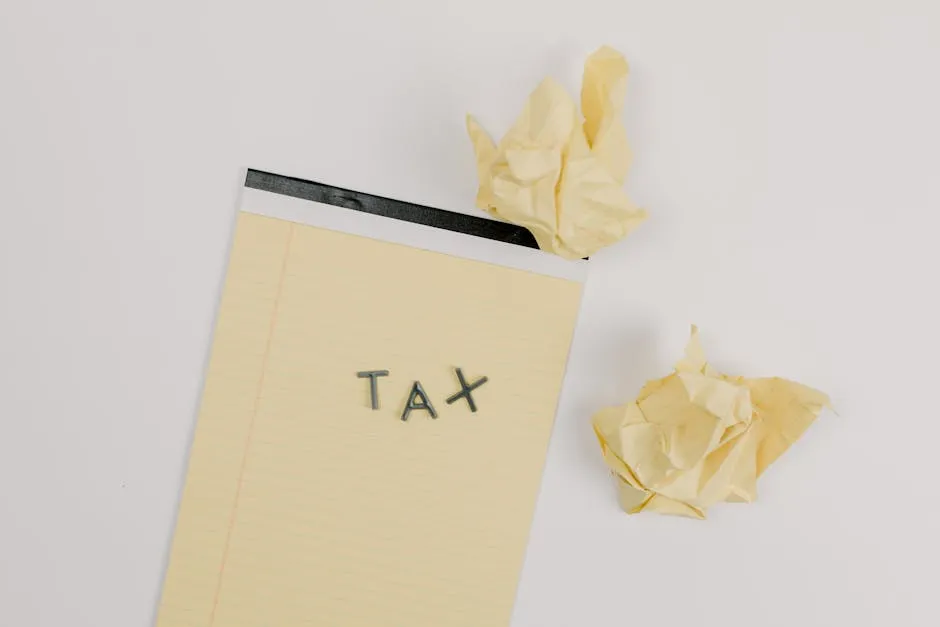
4.2 Bayesian Statistical Approaches
Bayesian statistics offers a refreshing alternative for evaluating evidence and power in research. Unlike traditional methods, Bayesian approaches incorporate prior information and beliefs about parameters. They allow researchers to update their beliefs as new data becomes available. This flexibility can lead to more informative and nuanced conclusions.
In the Bayesian framework, the focus shifts from p-values to probability distributions. Instead of simply determining whether an effect exists, researchers can estimate the likelihood of various outcomes. This aspect is particularly valuable when researchers face non-significant results. Instead of shrugging off the findings, Bayesian analysis provides a way to quantify uncertainty around the effect size. For an excellent introduction to Bayesian analysis, consider reading An Introduction to Bayesian Analysis: Theory and Methods by Jayanta K. Ghosh. It provides a solid foundation for anyone interested in this statistical approach.
One practical application is using Bayesian models to estimate the posterior distribution of a parameter of interest. This distribution combines prior beliefs with evidence from the data. For example, if a researcher suspects that a new medication might reduce symptoms of a disease, they can start with a prior belief about the effect size. After conducting the study, they update this belief based on the observed data. The result is a more comprehensive understanding of the medication’s efficacy.
Bayesian methods also facilitate decision-making under uncertainty. By providing a range of possible effect sizes with corresponding probabilities, researchers can make informed choices about future studies or interventions. This contrasts sharply with the binary decisions often driven by p-values, where results can hinge on arbitrary thresholds.
Moreover, Bayesian analyses can help address some limitations associated with post hoc power analyses. Instead of merely computing power based on observed data, Bayesian models incorporate the uncertainty inherent in the data collection process. This capability can lead to more robust conclusions about the likelihood of detecting true effects.
In summary, Bayesian statistical approaches present a powerful alternative for assessing evidence and power. They enrich the research process by allowing for the incorporation of prior knowledge and providing a clearer picture of uncertainty. Researchers can move beyond the constraints of p-values and post hoc power, engaging in a more meaningful dialogue about their findings. By embracing Bayesian methods, the scientific community can enhance the rigor and relevance of research outcomes, paving the way for better-informed decision-making.

5. Practical Implications for Researchers
Understanding the implications of statistical power is crucial for researchers. The concept of power, especially in relation to post hoc analyses, has significant consequences for how findings are communicated. Relying on post hoc power analysis can be problematic, leading to misinterpretations and confusion. Instead, researchers should focus on effective ways to present their results and acknowledge limitations.
First, it’s important to avoid the temptation to calculate post hoc power solely to justify non-significant findings. This approach can create a false sense of validity. Instead, researchers should emphasize the effect sizes and confidence intervals in their reports. These statistics provide a richer context for understanding the results, allowing readers to grasp not just whether an effect exists, but how substantial that effect might be. For more insights into this, you can refer to Practical Statistics for Data Scientists by Peter Bruce. It’s a key read for anyone navigating the world of data.
When researchers encounter non-significant results, they should consider discussing the implications of their findings within the framework of uncertainty. Rather than claiming a lack of effect, they can highlight the possibility of an effect while acknowledging limitations such as a small sample size or high variability. This approach fosters transparency and encourages a more nuanced interpretation of the data.
Furthermore, when designing future studies, researchers should prioritize prospective power analysis. This proactive approach aids in determining sample sizes necessary for detecting meaningful effects. By doing so, researchers can avoid the pitfalls associated with underpowered studies, which often lead to inconclusive results. It’s akin to preparing adequately for a big presentation rather than scrambling at the last minute.
Communicating findings effectively requires clarity and precision. Researchers should consider their audience and tailor their messaging accordingly. Lay audiences may benefit from simplified explanations, while fellow researchers might appreciate more detailed statistical discussions. Regardless of the audience, the goal should be to convey findings in an accessible and meaningful manner.

In conclusion, researchers must recognize the practical implications of statistical power. By moving away from post hoc power analysis and emphasizing effect sizes and confidence intervals, they can provide clearer insights into their findings. Prioritizing prospective power analysis during study design enhances the quality of research, ultimately leading to more reliable and interpretable outcomes. Embracing these practices fosters a culture of transparency and rigor within the scientific community.
Conclusion
To wrap things up, let’s recap some key takeaways about post hoc statistical power and its implications. First, prospective power analysis is essential for effective study design. It helps researchers determine the sample sizes needed to detect meaningful effects before data collection begins. This proactive approach reduces the risk of inconclusive results and enhances the quality of research.
On the flip side, post hoc power analysis comes with significant pitfalls. It is often criticized for being logically flawed and practically misleading. Calculating power after the fact does little to clarify findings, especially when results are non-significant. Instead of providing new insights, post hoc power merely reiterates what the p-value already conveys.
As an alternative, researchers should consider using confidence intervals and The Data Warehouse Toolkit: The Definitive Guide to Dimensional Modeling by Ralph Kimball. These approaches offer more informative insights into data, allowing for a richer understanding of effect sizes and uncertainties. By shifting focus from p-values and post hoc power to these alternatives, researchers can enhance the clarity and relevance of their findings.
Ultimately, the goal is to foster a culture of rigorous and transparent research. By prioritizing prospective power analysis and communicating findings effectively, researchers can contribute to a more informed scientific community. In the end, it’s not just about crunching numbers; it’s about making sense of them and using that understanding to drive meaningful advancements in knowledge.
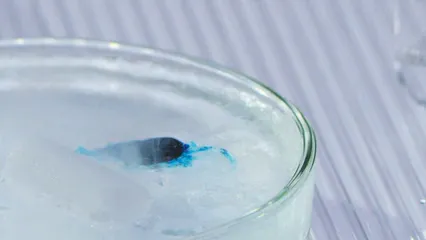
FAQs
Why is post hoc power analysis often criticized?
Post hoc power analysis is often criticized because it is logically flawed. It typically provides no new information beyond what is already indicated by the p-value. High p-values yield low post hoc power, while low p-values correspond to high power. Thus, it often leads to misleading interpretations of study results, especially when researchers try to justify non-significant findings.
What should researchers do if they obtain non-significant results?
When faced with non-significant results, researchers should focus on discussing the implications of their findings within the context of uncertainty. Instead of claiming there is no effect, they can explore the possibility of an effect while acknowledging limitations such as sample size or variability. Emphasizing effect sizes and confidence intervals can provide a more nuanced understanding of the results.
Can post hoc power analysis ever be useful?
While post hoc power analysis is generally discouraged, it may serve a purpose in specific contexts, such as discussions about the adequacy of a study’s sample size when planning future research. However, it should not be used to justify non-significant findings. Instead, researchers should focus on alternative methods like confidence intervals and Bayesian approaches for a more informative analysis of their data.
Please let us know what you think about our content by leaving a comment down below!
Thank you for reading till here 🙂
All images from Pexels