Introduction
Ever heard the phrase, “most cats prefer naps over work”? Well, that’s a classic example of a statistical syllogism, and it’s more relevant to our daily lives than we might think! A statistical syllogism is a nifty little argument that allows us to draw conclusions about individuals based on group statistics. It’s that friendly reminder that just because most people in a group do something doesn’t mean everyone does.
In various fields like psychology, marketing, and philosophy, statistical syllogism plays an essential role. Imagine a marketer saying, “Most millennials prefer streaming services.” This kind of reasoning helps shape marketing strategies, allowing businesses to target demographics effectively. In psychology, it aids in understanding behaviors by linking individual actions to broader trends. Philosophically, it invites us to question the validity of generalizations and the strength of our conclusions.
So, what’s the typical structure of a statistical syllogism? It generally follows this pattern: if a certain proportion of a group has a specific attribute, and an individual belongs to that group, we can infer that the individual likely possesses that attribute too. Simple, right?
In this article, we’ll unpack this concept further. From the basics to its practical applications, we’ll provide a comprehensive guide to statistical syllogism. Get ready to sharpen your critical thinking skills and learn how to navigate statistics like a pro!
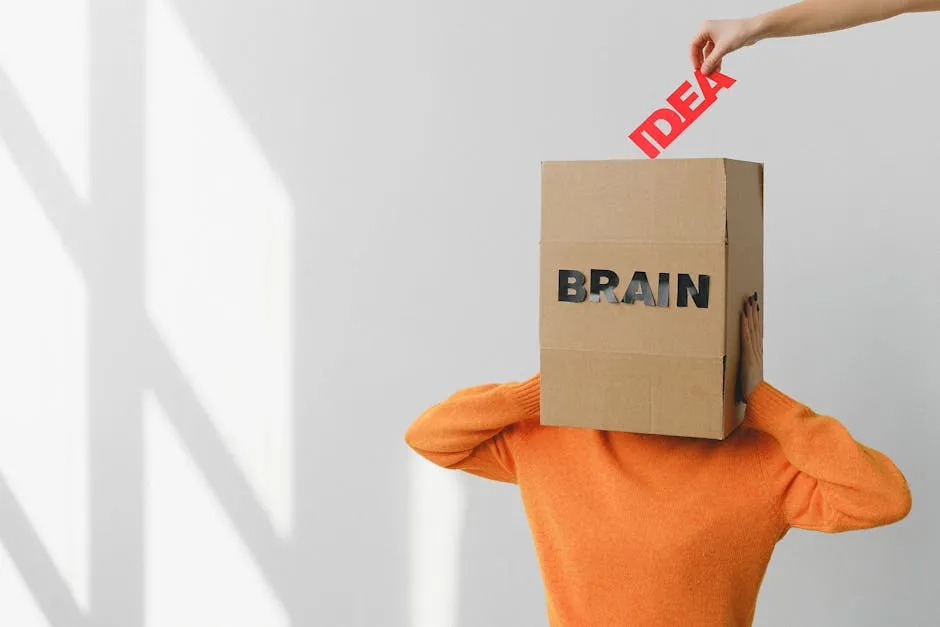
Part 1: The Basics of Statistical Syllogism
Definition and Structure
Statistical syllogism is a fascinating type of inductive reasoning that helps us draw conclusions about individuals based on generalizations about groups. Unlike deductive reasoning, which guarantees conclusions if the premises are true, statistical syllogism operates on probabilities. This means that while the premises may lead to a reasonable conclusion, they don’t guarantee its truth.
The general structure of a statistical syllogism can be represented as follows:
- P1: Proportion of group (X) having attribute (Y)
- P2: Individual (I) is part of group (X)
- C: Therefore, individual (I) likely has attribute (Y)
Let’s illustrate this with an example. Imagine we know that 90% of students enjoy pizza. If we then say, “Alex is a student,” we can conclude, “Therefore, Alex likely enjoys pizza.” Here, we’re relying on a statistical generalization to make an inference about an individual.
This reasoning form is incredibly useful in everyday life. We often use statistical syllogisms without even realizing it! For instance, if we hear that “most pets are dogs,” we automatically lean towards the idea that a friend’s pet might be a dog. However, remember that these conclusions are probabilistic. The premises could be true, and the conclusion could still be false, although that’s less likely.
If you’re looking to deepen your understanding of statistical reasoning, check out Statistical Reasoning: A Practical Guide to Critical Thinking. It’s a fantastic resource to help you navigate the complex world of statistics!
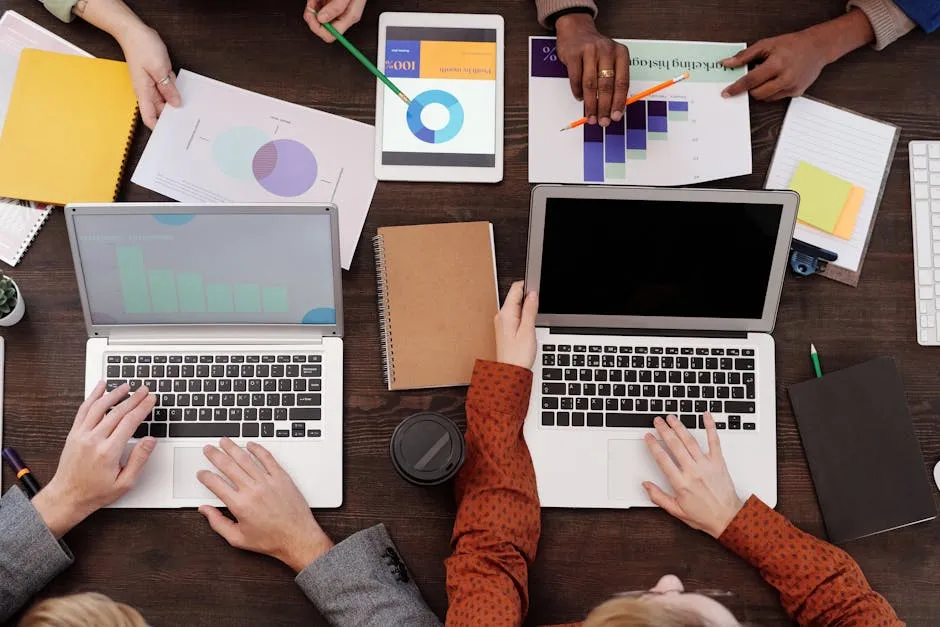
Inductive Nature
The inductive nature of statistical syllogisms is what makes them both intriguing and essential for critical reasoning. Unlike deductive arguments, which provide certainty, statistical syllogisms give us probabilities. This means that while we can make educated guesses, we must also be aware of the potential for error.
For example, if we know that 60% of people in a city prefer chocolate ice cream, and we meet someone from that city, we might think they likely prefer chocolate ice cream too. But hold your horses! Just because the majority favors chocolate doesn’t mean our new friend won’t be a die-hard vanilla fan.
When evaluating a statistical syllogism, it’s crucial to assess the strength of the argument based on the premises. A strong syllogism relies on accurate statistical data and a representative sample. If the data is skewed or the sample is too small, the conclusions drawn may lead us astray.
To further enhance your statistical knowledge, consider reading The Art of Statistics: Learning from Data. It’s a brilliant guide that will help you understand how to interpret data effectively!
In summary, statistical syllogisms allow us to make informed inferences based on group data. They are a valuable tool in our reasoning toolkit, helping us navigate the sea of information we encounter daily. The next time you hear a generalization, take a moment to consider how it might apply to individuals. The world of probabilities is waiting to be explored!
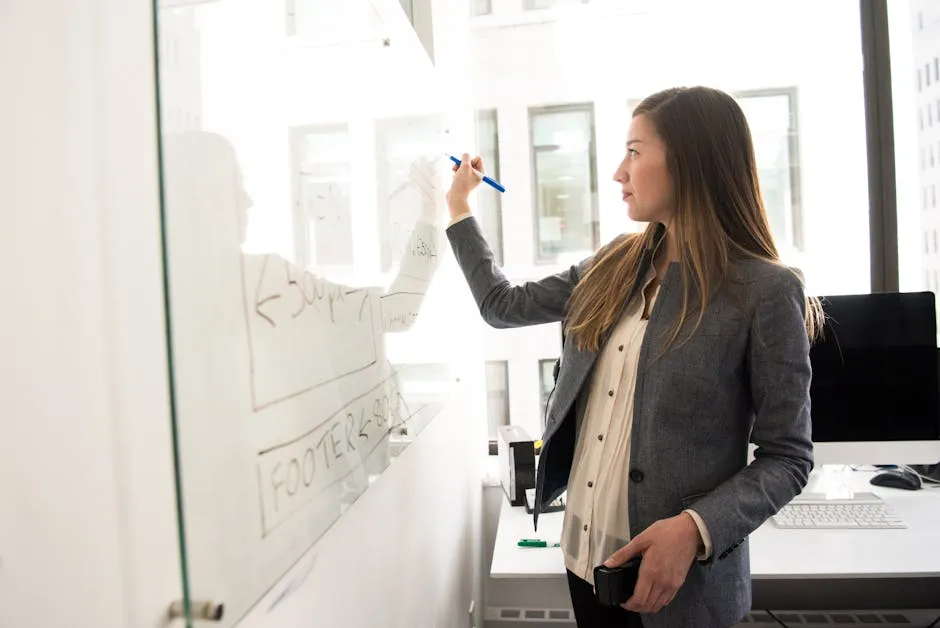
Qualifying Words and Their Impact
When it comes to statistical syllogisms, qualifying words are the unsung heroes—or villains, depending on how you look at it. Words like “most,” “frequently,” and “almost never” can significantly affect the strength of conclusions we draw. They can turn a mild suggestion into a bold statement or vice versa.
Let’s consider the classic example:
- “Most cats are aloof.”
If you were to say, “Fluffy is a cat; therefore, Fluffy is aloof,” you might feel pretty confident. But what if the premise was different?
- “Some cats are aloof.”
Now, saying Fluffy is aloof feels riskier, doesn’t it? The shift from “most” to “some” reduces the probability of Fluffy fitting the aloof stereotype. This little swap has a powerful impact on how we perceive the conclusion.
Another example could be:
- “Frequently, people who eat pizza are happy.”
If you say, “Chris eats pizza, so Chris is likely happy,” the word “frequently” softens the blow. Yes, there’s a correlation, but it doesn’t guarantee a happy ending for Chris.
But what if you tweak it to:
- “Always, people who eat pizza are happy.”
Now, we’re talking! The use of “always” dramatically strengthens the conclusion. Be careful, though. This could lead to some serious pizza-induced misconceptions!
In summary, the qualifying words we use can color our conclusions in striking ways. So, the next time you hear a statistic or make an inference, pay close attention to those qualifiers. They might just be the key to understanding the true strength of the argument!
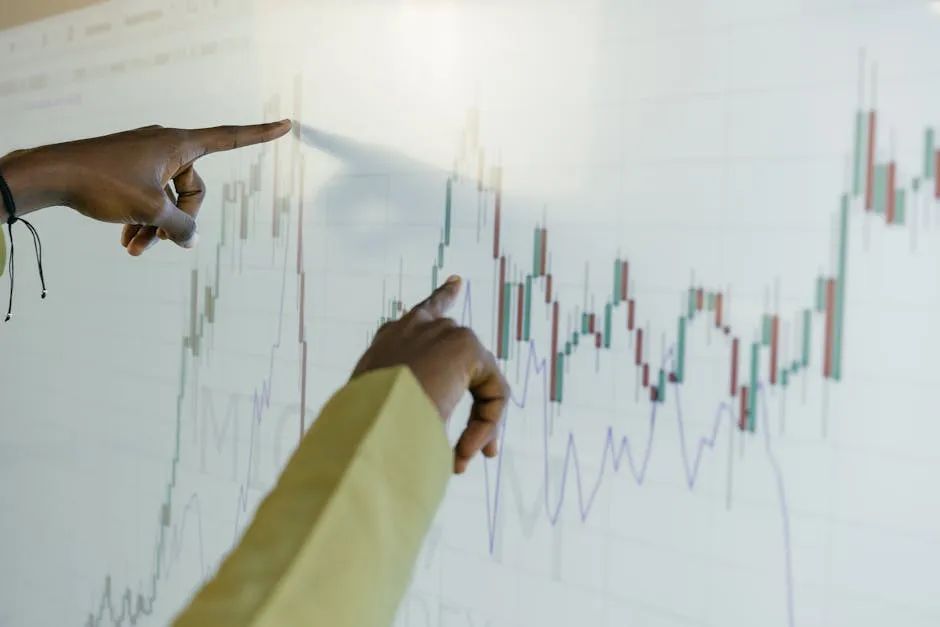
Common Fallacies in Statistical Syllogisms
Statistical syllogisms can be powerful reasoning tools. However, they come with some common pitfalls. Let’s explore a few of these fallacies.
Accident
The accident fallacy occurs when a generalization is misapplied to an exception. Imagine a scenario where “most cats dislike water.” If someone asserts, “Whiskers is a cat; therefore, he hates water,” they’ve fallen into the accident trap. While the generalization may hold for the majority, it doesn’t apply to every cat. Whiskers might just be that rare feline who enjoys a refreshing splash!
Converse Accident
Next up is the converse accident fallacy. This one happens when a conclusion is drawn too broadly from a specific case. For example, “My neighbor, who is a teacher, is very generous.” If someone concludes, “All teachers are generous,” they are generalizing from that one example. Sure, your neighbor might be a gem, but not every teacher will shower you with kindness!
Faulty Generalization
Lastly, we have the faulty generalization fallacy. This occurs when conclusions are based on inadequate evidence. Suppose a person claims, “I met two doctors, and they were both grumpy. Therefore, all doctors must be grumpy.” That’s a shaky conclusion, based on a sample size so small that it barely scratches the surface of the diverse world of doctors.
In summary, while statistical syllogisms can help us make inferences, we must tread carefully. Misapplying generalizations, overgeneralizing from specific cases, or drawing conclusions from weak evidence can all lead us astray. Recognizing these fallacies will sharpen our reasoning skills and help us make sound judgments. So, keep your thinking cap on and question those assumptions!
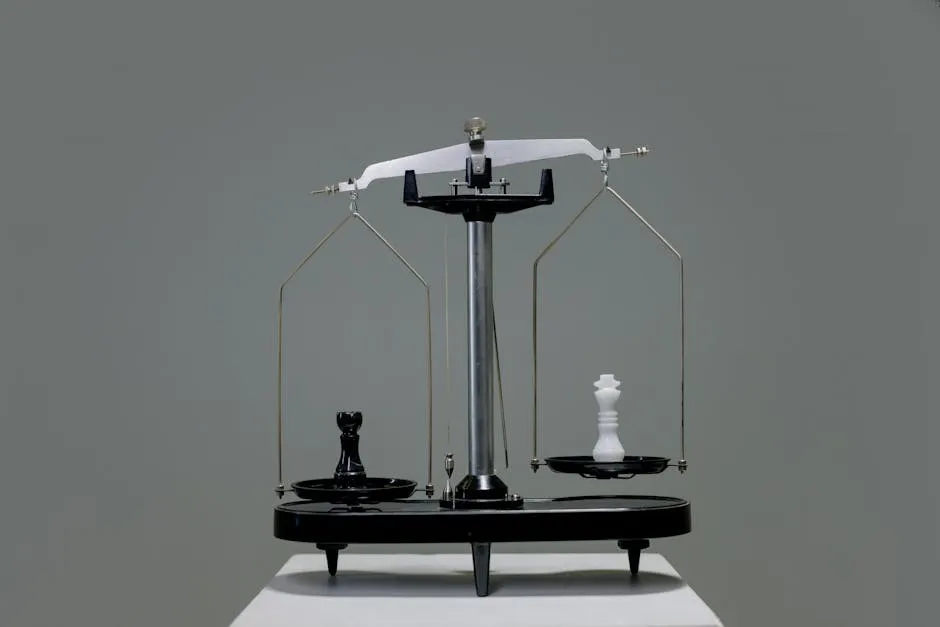
Statistical Syllogism vs. Other Forms of Reasoning
Statistical syllogism often gets tangled with other reasoning forms, like deductive reasoning and enumerative induction. Let’s untangle this web!
Deductive reasoning is the superhero of logic. It guarantees conclusions if the premises are true. For example, if we know that “All birds can fly” and “A penguin is a bird,” we conclude that “A penguin can fly.” Spoiler alert: that’s not true! This reasoning locks you into certainty, but with a risk of false premises.
On the flip side, enumerative induction is a bit more relaxed. It observes patterns and projects them onto unobserved cases. For instance, if we observe that “Most swans are white,” we might conclude that “The next swan we see will be white.” It’s less rigid than deductive reasoning because it allows for some wiggle room.
Now, let’s compare them with statistical syllogism. This reasoning form is more probabilistic. It uses group statistics to infer something about an individual. For instance, “70% of students enjoy math; Alex is a student; thus, Alex likely enjoys math.” Here, the conclusion isn’t guaranteed but is based on statistical likelihood.
The key differences lie in structure and strength. Deductive reasoning leads to definitive conclusions. Enumerative induction offers probable outcomes based on observations. Statistical syllogism provides a blend, relying on statistical proportions to make a best guess about individuals.
In applications, deductive reasoning shines in mathematics and formal logic. Enumerative induction is handy in scientific studies. Meanwhile, statistical syllogism finds its home in everyday decision-making, marketing strategies, and social sciences, reflecting our reliance on group data to make personal judgments. For more insights on effective data analysis, check out tips for effective data analysis in economics and statistics.
Understanding the nuances of data analysis can enhance your reasoning skills, especially when applying statistical syllogisms. tips for effective data analysis in economics and statistics
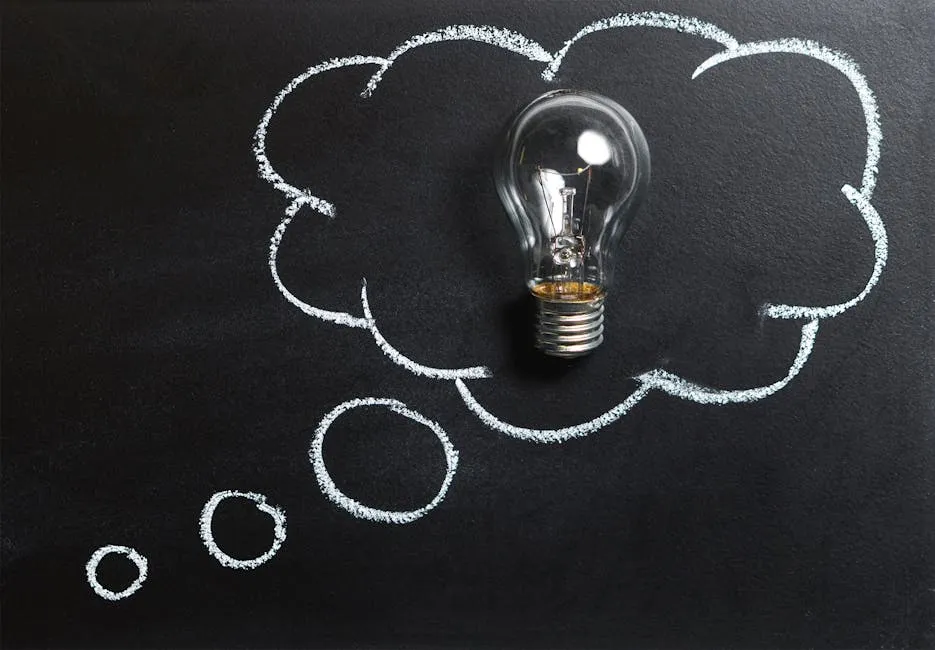
Critical Thinking and Statistical Syllogism
Understanding statistical syllogism is a game-changer for your critical thinking skills. It encourages us to question the validity of claims based on group data. That’s a big deal in a world overflowing with statistics!
To critically assess statistical claims, follow these nifty tips:
- Check the Source: Always examine where the data comes from. Is it a reputable source, or are we just trusting a random blog? (I mean, who knows what they might say about pizza preferences!)
- Look for Sample Size: A small sample might lead to weak conclusions. If someone claims, “Everyone I know loves pineapple on pizza,” it might not represent pizza lovers everywhere. Their friends could just be a quirky bunch!
- Examine the Context: Don’t take statistics at face value. Consider how the data was collected and if it truly reflects the population. If the survey asked people at a pizza convention, the results might be skewed.
- Question Qualifiers: Pay attention to words like “most” and “some.” They can change the entire meaning of a claim. A statement like “Some cats are aloof” doesn’t hold the same weight as “Most cats are aloof.” Keep those distinctions in mind!
- Challenge Assumptions: Just because a claim has statistical backing doesn’t mean it’s correct. Always be curious and question the underlying assumptions.
By honing these skills, you’ll become a statistical sleuth in everyday life. You’ll be better equipped to sift through the sea of information and arrive at informed conclusions.
For those interested in diving deeper into statistical inference, I highly recommend Statistical Inference by George Casella. It’s an essential read for anyone looking to enhance their understanding of statistics!
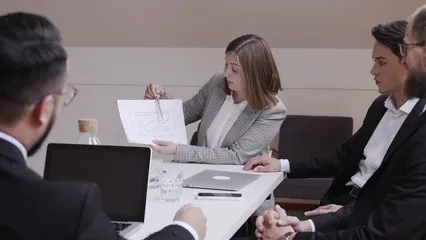
Conclusion
In this whirlwind journey through statistical syllogism, we’ve uncovered its nuances and significance. We explored how this reasoning form differs from deductive reasoning and enumerative induction. Each has its own playground, but statistical syllogism stands out by allowing us to make educated guesses based on group data.
We learned that critical thinking is essential when assessing statistical claims. With the right tools, we can navigate the statistical sea like pros! Remember the importance of checking sources, examining sample sizes, and questioning assumptions.
Understanding statistical syllogisms is vital for making informed decisions. In a world filled with data, we can’t simply accept every statistic thrown our way. We must analyze, question, and critically evaluate the information. This ensures we don’t fall into the trap of faulty reasoning.
To deepen your understanding, you might want to check out The Elements of Statistical Learning: Data Mining, Inference, and Prediction. It provides valuable insights into statistical learning and its applications!
So, the next time you hear a statistic, remember: it’s not just numbers; it’s a call to think critically and make sound judgments. Embrace your inner detective, and let statistical syllogism be your trusty sidekick on the quest for truth!

FAQs
What is a statistical syllogism?
A statistical syllogism is an inductive reasoning form. It lets us draw conclusions about an individual based on statistical generalizations about a group. For example, if we know that “most cats are aloof,” we can infer that a specific cat, like Fluffy, is likely aloof too. This reasoning structure relies on probabilities, not certainties. So, while it’s a handy tool, it doesn’t guarantee that our conclusion is correct.
How do statistical syllogisms differ from deductive reasoning?
The main difference lies in certainty. Deductive reasoning guarantees conclusions if the premises are true. For instance, if “All birds can fly” and “A penguin is a bird,” then we can conclude that “A penguin can fly.” This is not true, but the structure here is solid. In contrast, statistical syllogisms operate on probabilities. They suggest that conclusions are likely but not certain. For example, “Most students like pizza; Alex is a student; therefore, Alex likely likes pizza.” Here, Alex’s pizza preference isn’t guaranteed.
What are some examples of statistical syllogism in everyday life?
Let’s sprinkle some relatable examples into the mix! 1. **Weather Forecasts**: “Most days in July are sunny. Today is July 15. Therefore, today is likely sunny.” While this reasoning is common, it doesn’t mean every July day is a sun-soaked delight! 2. **Shopping Habits**: “Most adults prefer online shopping. Lisa is an adult. So, Lisa likely prefers online shopping.” This could be true, but Lisa might just love the thrill of in-person shopping! 3. **Diet Choices**: “Most people who eat vegetables are healthy. John eats vegetables. Therefore, John is likely healthy.” While John’s veggie love is commendable, he might still indulge in a cupcake or two! 4. **Pet Preferences**: “Most people with pets have dogs. Jamie has a pet. Therefore, Jamie likely has a dog.” However, Jamie could be the proud parent of a sassy cat! These examples show how statistical syllogisms pop up in our daily conversations and thoughts. They’re a part of our reasoning toolkit, helping us make sense of the world around us.
What should I consider when evaluating a statistical syllogism?
Evaluating statistical syllogisms requires a keen eye for detail. Here are some practical tips: 1. **Evaluate the Premises**: Assess the generalization in the first premise. Is it accurate? Check for sample size and representation. A sample of two people doesn’t cut it! 2. **Context Matters**: Ensure the premises are relevant to the conclusion. If the group has specific traits, the individual should fit that mold as well. For example, “Most cats are playful; Mittens is a cat; therefore, Mittens is playful.” But what if Mittens is a couch potato? 3. **Consider the Qualifiers**: Words like “most” and “some” can drastically change conclusions. “Most students study hard” feels more robust than “Some students study hard.” Be wary of how qualifiers sway the argument. 4. **Question the Statistics**: Are they up-to-date? Are they sourced from a reliable location? Statistics can be twisted to tell a compelling story, so do your homework! 5. **Be Mindful of Bias**: Recognize any potential biases in the premises. If the data is skewed, the conclusions might lead you astray. By applying these tips, you’ll sharpen your critical thinking skills and evaluate arguments like a true detective!
Can statistical syllogisms lead to misconceptions?
Absolutely! While statistical syllogisms can be beneficial, they can also lead to some head-scratching misconceptions. Here are a few pitfalls to avoid: 1. **Overgeneralization**: Just because “most” people behave a certain way doesn’t mean everyone does. If “most students enjoy math,” it doesn’t mean every student will be a math whiz. So, let’s keep those assumptions in check! 2. **Ignoring Exceptions**: Every rule has exceptions. If “most dogs are friendly,” that doesn’t mean every dog will greet you with a wagging tail. Some might be guarding their turf! 3. **Misleading Averages**: Statistics can be slippery! “The average income in a city is $50,000” could be skewed by a few high earners. This means that most people might earn much less. Be cautious of how averages shape perceptions. 4. **Confirmation Bias**: It’s easy to latch onto statistics that support our beliefs while disregarding those that don’t. If you believe “all teenagers are glued to their phones,” you might overlook the few who prefer reading books. To avoid these misconceptions, stay curious and question generalizations. Remember, just because a conclusion appears likely doesn’t mean it’s true. Embrace the complexity of life, and keep your reasoning sharp!
Please let us know what you think about our content by leaving a comment down below!
Thank you for reading till here 🙂
All images from Pexels