Introduction
Statistical tables are like the ultimate cheat sheet for data lovers. They present complex data in a neat, organized way, allowing analysts to make sense of the numbers without losing their minds. Imagine trying to sift through hundreds of data points scattered across endless pages. Sounds like a nightmare, right? Enter statistical tables, the knights in shining armor of data analysis.
These tables are crucial in various fields, including economics, science, and social research. They help researchers, economists, and scientists summarize and interpret data efficiently. From t-tables used in hypothesis testing to ANOVA tables that help compare multiple groups, these tables are as diverse as they are useful.
In this article, you’ll learn all about statistical tables—what they are, the different types available, and how to read them like a pro. By the end, you’ll be ready to tackle any data-related challenge that comes your way, armed with the knowledge of statistical tables. So, buckle up and get ready for a fun ride through the world of numbers!
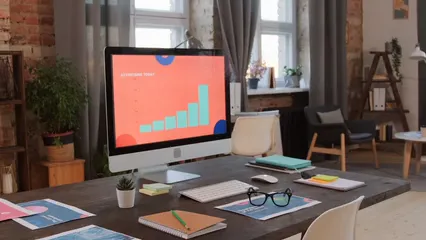
What are Statistical Tables?
Definition of Statistical Tables
Statistical tables are structured formats that display data in rows and columns. They serve to summarize vast amounts of information, transforming chaos into clarity. Think of them as the condensed version of a lengthy novel—providing essential plots without all the fluff.
These tables allow for quick reference and easy comparison of data. By grouping related numbers together, they enable users to spot trends and patterns that might otherwise go unnoticed. Whether you’re analyzing test scores or tracking economic indicators, statistical tables help you make sense of the information at hand.
Want to dive deeper into data analysis? Check out Data Analysis with R: A Hands-On Guide. This book can help you harness the power of R programming to analyze your data effectively.
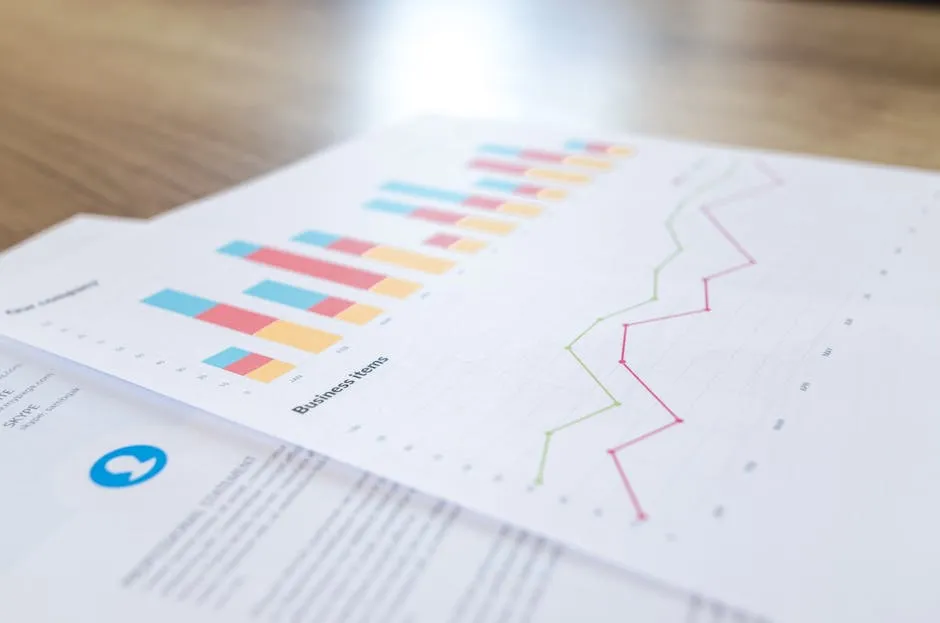
Types of Statistical Tables
Statistical tables come in various shapes and sizes, each designed for specific purposes. Here’s a quick overview of some common types:
- Descriptive Statistics Tables: These tables summarize key data points like mean, median, and mode. They give a snapshot of the data set, helping you understand its central tendencies. For more on this, check out our guide on descriptive statistics in manufacturing plant.
- Inferential Statistics Tables: These include t-tables, z-tables, F-tables, and more. They help conduct hypothesis tests, estimate population parameters, and determine the significance of results. Imagine these as your trusty sidekicks when diving into inferential statistics!
For a better understanding, let’s visualize these tables:
- Descriptive Statistics Table: Picture a simple table displaying average scores of students in a class, alongside the highest and lowest scores. Easy to read and packed with information!
- t-Table: This table lists critical values of t based on degrees of freedom and significance levels. It’s a must-have for anyone conducting a t-test to determine if there’s a significant difference between two means.
- ANOVA Table: This table helps analyze the variance among group means. It displays sums of squares, degrees of freedom, and F-values, making it essential for researchers comparing multiple groups.
Statistical tables, in all their glory, provide a compact and efficient means of presenting data. They are indispensable tools for anyone working with numbers, making complex analyses digestible and actionable.
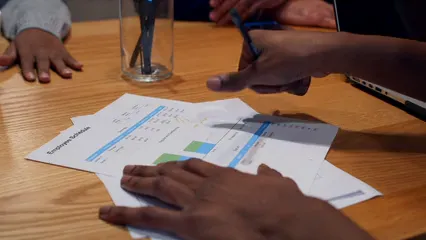
Importance of Statistical Tables in Data Analysis
Facilitating Comparison
Tables excel at making comparisons straightforward. Imagine tracking economic indicators like GDP or inflation rates over several years. A well-structured table allows you to see trends at a glance, rather than wading through pages of text.
For example, consider GDP growth rates across countries. A table can display the GDP of Country A, Country B, and Country C side by side for the last five years. Instantly, you spot which country is thriving and which is struggling.
Now, let’s consider inflation rates. A table showing monthly inflation rates for different years allows you to quickly identify patterns. Are prices rising faster in 2023 compared to 2022? With a table, these insights pop out like a neon sign, guiding your analysis.
Statistical tables make cross-group comparisons like comparing different demographic factors, such as income levels across age groups. They provide a snapshot that enhances understanding and drives deeper analysis.

Enhancing Clarity and Understanding
Complex data can be intimidating. Yet, statistical tables simplify this complexity. They break down information into rows and columns, making large datasets more approachable.
Take educational research as an example. A table summarizing test scores across various demographics can clarify how different groups perform. It highlights disparities in educational outcomes, making it easier to identify where intervention may be needed.
In healthcare, statistical tables can show patient outcomes based on treatment types. By comparing recovery rates, doctors can make informed choices about the best course of action for future patients.
When data is organized logically in a table, it enhances clarity. Readers can quickly grasp the information without feeling overwhelmed. This clarity fosters better discussions and decision-making.
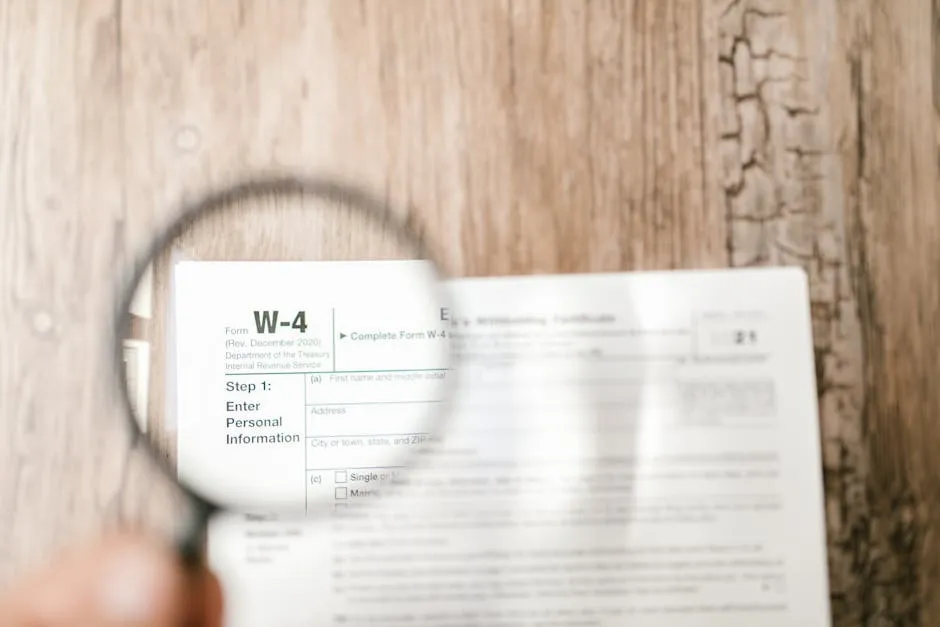
Supporting Decision-Making
Statistical tables don’t just provide insights; they drive important decisions. In business, executives often rely on tables to assess performance metrics. For instance, a table comparing quarterly sales figures can highlight trends that inform strategic pivots.
Consider a case study of a retail company assessing its product lines. A statistical table detailing sales performance over several quarters can reveal which products are underperforming. Armed with this knowledge, management can decide whether to discontinue a product or boost marketing efforts.
In policy-making, statistical tables offer a solid foundation for decisions. For instance, a government might analyze economic indicators through tables to determine if stimulus measures are needed during a recession. The data presented clearly aids policymakers in making timely, informed choices.
Statistical tables are not just tools; they are essential assets in the decision-making process. They ensure that every decision is backed by data, minimizing guesswork and maximizing outcomes.
In summary, statistical tables are vital for facilitating comparison, enhancing clarity, and supporting decision-making. Their role in data analysis cannot be overstated. They turn raw data into actionable insights, making them indispensable in various fields.
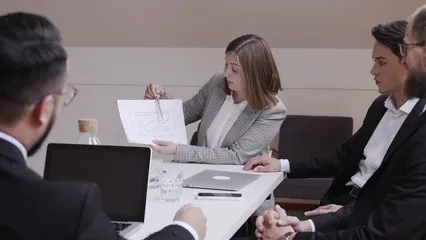
T-Table
A t-table is essential in hypothesis testing. It helps determine whether the means of two groups are statistically different. This table lists critical t-values based on degrees of freedom and significance levels. So, what does that mean for you? Simply put, it’s your go-to for deciding if your findings are statistically significant.
Let’s look at a practical example. Imagine you’re testing a new teaching method. You have two groups of students: one using the new method and another using traditional teaching. After the lessons, you measure their test scores.
To find out if the new method really made a difference, you calculate the t-value from your data. Then, consult the t-table to find the critical value based on your chosen significance level (let’s say 0.05) and degrees of freedom (calculated based on your sample sizes). If your t-value exceeds the critical value, congratulations! You can reject the null hypothesis, indicating a significant difference in means.
Understanding how to utilize a t-table is crucial for hypothesis testing. For more information, refer to our statistics hypothesis testing cheat sheet.
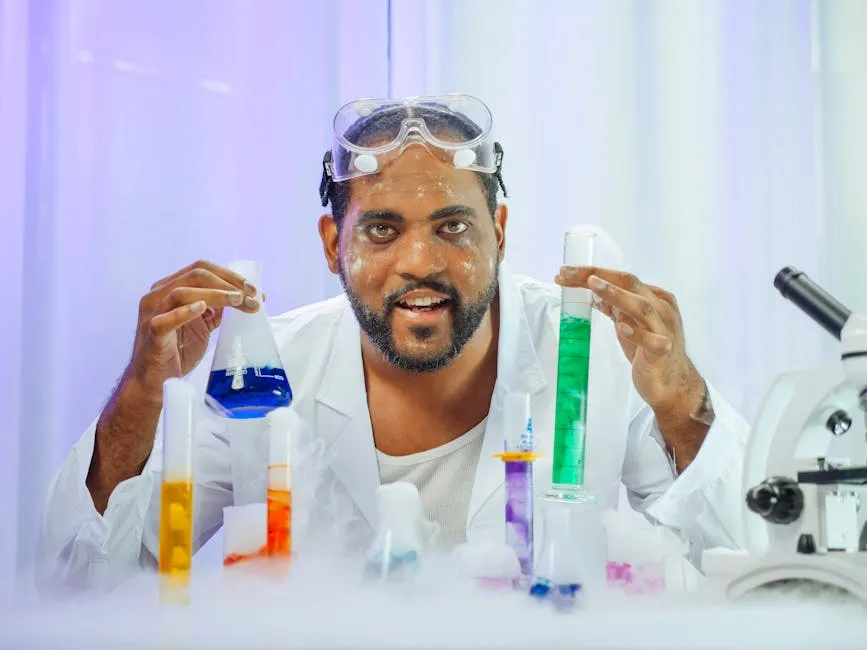
Z-Table
A z-table is crucial in standard normal distribution. It provides the probability of a z-score occurring under the standard normal curve. Why is this important? Well, it helps us understand how far a data point is from the mean, expressed in standard deviations.
Consider you’re creating confidence intervals for a new product’s price. You’ve collected data, calculated the sample mean, and standard deviation. To find the confidence interval at 95%, you need the z-score, which is approximately 1.96 for this level.
Using the z-table, identify the z-value for 95% confidence. With this, you can calculate your confidence interval by applying the formula: mean ± (z-score * standard error). This reveals the price range in which you can expect the true mean to fall, helping in pricing strategies.
For those looking to master data analysis, consider picking up Python for Data Analysis. This book will help you leverage Python’s capabilities to analyze and visualize your data effectively.
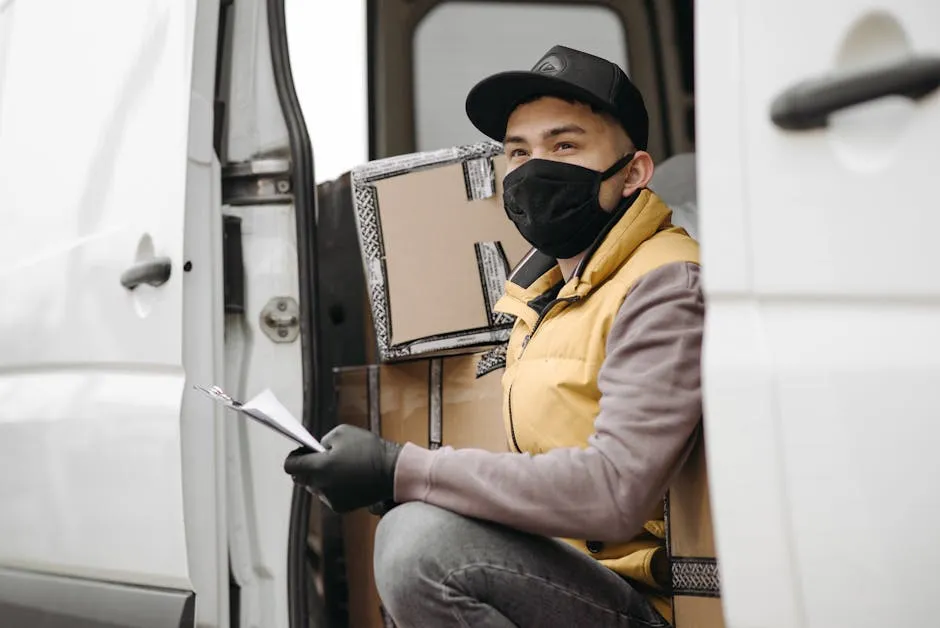
ANOVA Table
The ANOVA table plays a vital role in analysis of variance. It helps compare means across multiple groups, determining if at least one group differs significantly from the others. Why does this matter? It saves you from running multiple t-tests, which could inflate your chances of a Type I error.
Let’s say you’re evaluating the effectiveness of three different diets on weight loss. Your ANOVA table summarizes the variance within each diet group and between them. It shows sums of squares, degrees of freedom, mean squares, and the F-statistic.
If your F-statistic is significant, you conclude that at least one diet leads to different weight loss results. You can then perform post-hoc tests to pinpoint which diets differ. This approach provides a comprehensive understanding of your data.
For an insightful read on data science principles, consider Data Science for Business: What You Need to Know About Data Mining and Data-Analytic Thinking. This book provides a solid foundation for understanding how data analytics can drive business decisions.
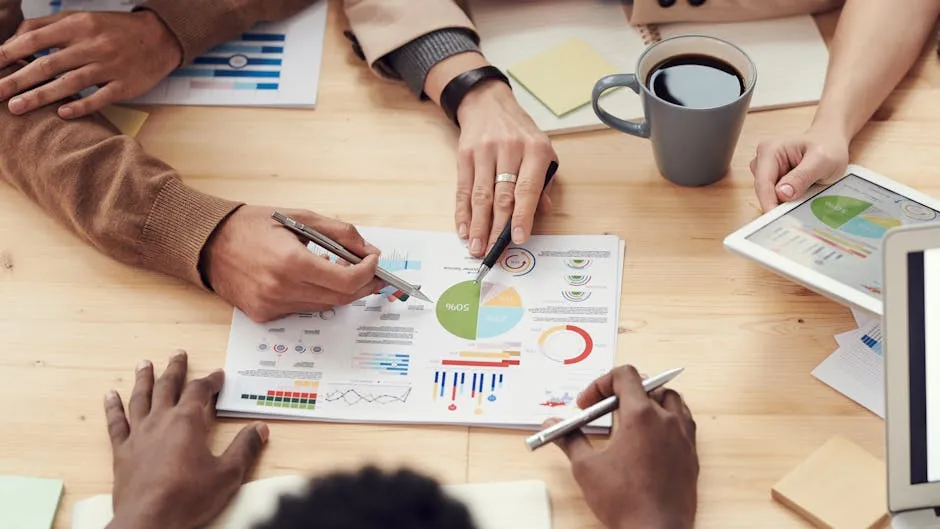
Chi-Square Table
The chi-square table is essential for analyzing categorical data. It helps determine if there’s a significant association between two categorical variables. Why should you care? It’s widely used in fields like marketing, where understanding consumer behavior is key.
For instance, imagine conducting market research to see if gender influences product preference. You gather data and create a contingency table comparing preferences across genders. Using the chi-square test, you calculate the chi-square statistic.
Now, consult the chi-square table to find the critical value based on your degrees of freedom. If your calculated statistic exceeds this critical value, you can confidently say there’s a significant association between gender and product preference. This insight can guide targeted marketing efforts and product development strategies.
For more insights on statistical concepts, grab a copy of Naked Statistics: Stripping the Dread from the Data. This book demystifies statistics and makes it accessible for everyone.
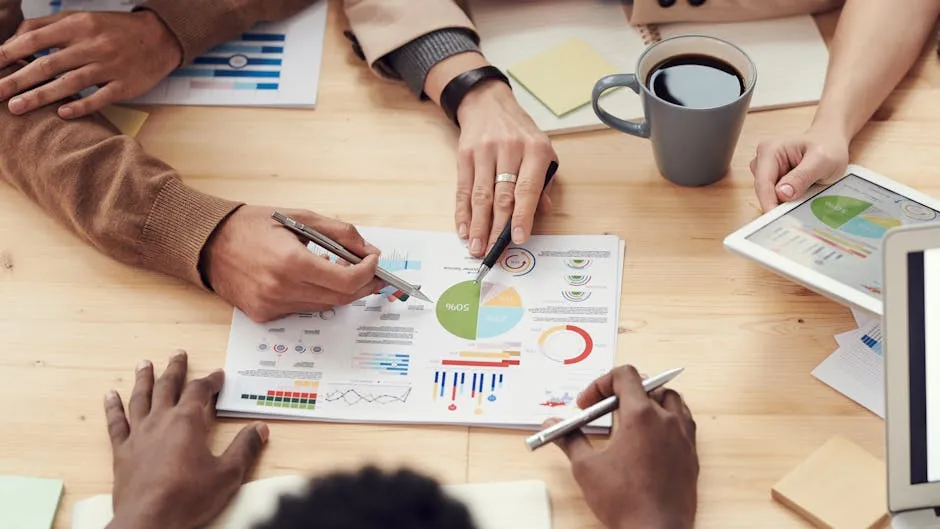
Understanding Table Structure
Statistical tables are the backbone of data representation. They typically consist of headers, rows, and columns. Headers label each column, providing context. Rows display individual data points, while columns organize these points into categories.
Let’s visualize this. Imagine a table presenting a school’s test scores. The header might read “Student Name,” “Score,” and “Grade.” Below, each row features a student’s name, their corresponding score, and the grade achieved. This simple structure allows for easy comparisons and quick insights.
A well-structured table should have clear and concise headers. Rows should maintain uniformity, and columns should align neatly. Here’s an example of a well-structured table:
Student Name | Score | Grade |
---|---|---|
Alice | 90 | A |
Bob | 75 | B |
Charlie | 60 | C |
This format makes it easy to compare scores and grades at a glance. The visual clarity offered by well-structured tables is essential for effective data analysis.
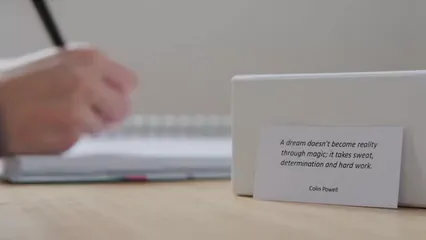
Steps to Interpret Data
Reading statistical tables doesn’t have to be daunting. Follow these simple steps to master data interpretation.
- Identify the Headers: Start by examining the headers. They tell you what each column represents. This context is crucial for understanding the data.
- Examine the Rows: Look at each row for individual data points. Each row represents a separate observation or grouping.
- Understand the Values: Pay attention to the values in the table. Are they averages, counts, percentages, or something else? Recognizing this will help you interpret the data accurately.
- Look for Patterns: Once you grasp the basics, start looking for trends. Are there any noticeable increases or decreases? Do certain categories perform better than others?
For interactive learning, here’s a simple exercise: Find a table that shows sales data for different products. Identify the headers, read the values, and determine which product had the highest sales. This practice will reinforce your understanding of how to read and interpret statistical tables effectively.
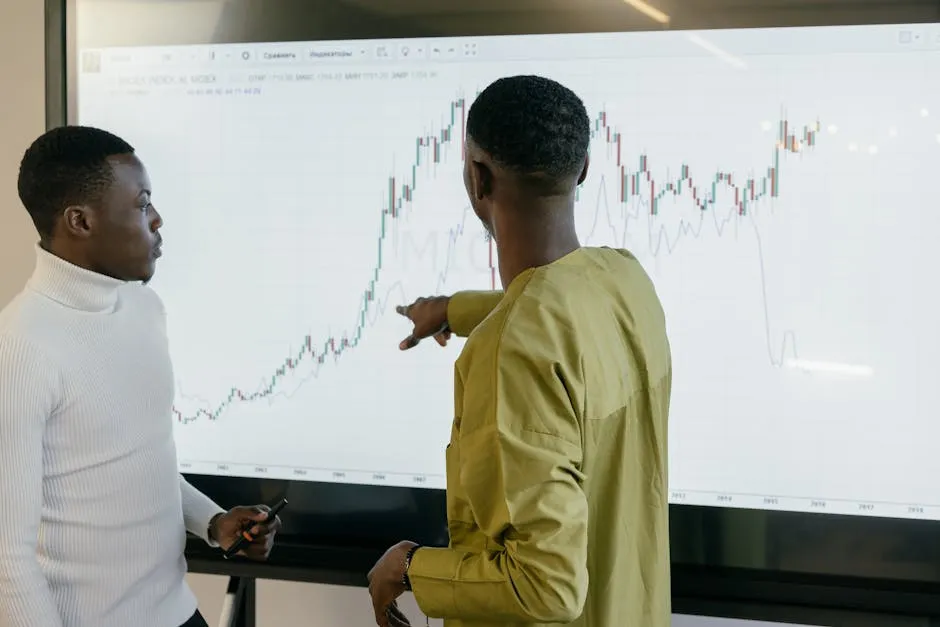
Common Mistakes to Avoid
Interpreting data from tables can trip up even seasoned analysts. Here are some common pitfalls and how to dodge them.
- Ignoring Headers: Failing to read the headers can lead to misunderstanding the data. Always check them first!
- Overlooking Footnotes: Tables often contain notes explaining specific data points or calculations. Ignoring these can lead to misinterpretation.
- Assuming Uniformity: Not all rows or columns represent the same type of data. Make sure you understand the context before jumping to conclusions.
- Rushing the Analysis: Take your time. Data interpretation is a skill that requires patience and careful thought.
To ensure accurate data interpretation, keep these tips in mind:
- Double-check the units of measurement.
- Look for visual cues, like highlights or bolding, that indicate significant data.
- Practice with various tables to build familiarity and confidence.
By avoiding these mistakes and following best practices, you’ll improve your ability to read and interpret statistical tables.
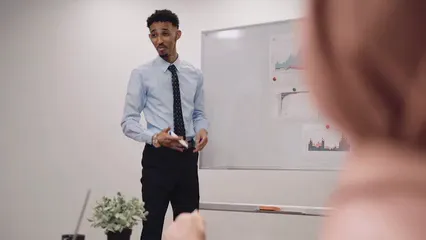
Best Practices in Table Design
Creating effective statistical tables is akin to crafting a good recipe. You need the right ingredients: clarity, simplicity, and relevance. Let’s break these down.
Clarity: A table should communicate data clearly. Use concise titles and clear headings. Avoid jargon unless necessary. For example, instead of “Mean Sales per Annum,” opt for “Average Yearly Sales.” This small change can make a massive difference.
Simplicity: Keep it straightforward. Too much information can overwhelm readers. Focus on key data points. A well-structured table with fewer rows and columns often delivers a better impact than a cluttered one.
Relevance: Only include data that serves your purpose. Irrelevant information can confuse readers. For instance, if you’re showcasing sales data, don’t clutter it with unrelated metrics like customer demographics.
Good vs. Bad Table Designs:
- Good Table: Imagine a table comparing monthly sales figures. It has clear headers, limited colors, and uses bold for totals. This design lets the data shine.
Month | Sales ($) | Growth (%) |
---|---|---|
January | 10,000 | – |
February | 12,000 | 20% |
March | 15,000 | 25% |
– Bad Table: Now picture the opposite. A table packed with unnecessary metrics, bright colors everywhere, and unclear headings. It’s a visual headache that makes readers want to run away.
Month | Sales ($) | Customer Feedback | Returns | New Customers | Growth % |
---|---|---|---|---|---|
January | 10,000 | Good | 50 | 200 | – |
February | 12,000 | Excellent | 30 | 300 | 20% |
March | 15,000 | Fair | 25 | 250 | 25% |
Which one would you prefer to read? Exactly!
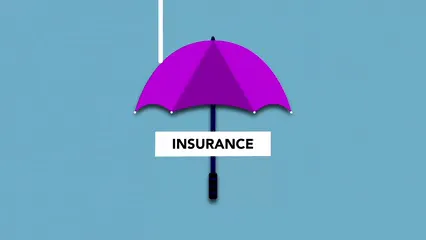
Software Tools for Creating Tables
When it comes to creating statistical tables, several software tools can make your life easier. Here’s a quick overview of some popular options:
- Excel: This classic tool is user-friendly and widely accessible. It allows for easy data manipulation and table creation. Want to master Excel? Check out Excel 2021 for Dummies to unlock its full potential!
- R: A favorite among statisticians, R offers powerful packages for customized table creation and complex analyses.
- SPSS: This software is perfect for social sciences. It simplifies data analysis and provides intuitive table generation features.
Let’s focus on Excel for a brief tutorial. Here’s how to create a simple statistical table:
- Open Excel: Start a new workbook.
- Input Data: Enter your data into rows and columns. Make sure to label each column appropriately.
- Select Data: Highlight the data you want to include in your table.
- Insert Table: Go to the “Insert” menu and click “Table.” Ensure “My table has headers” is checked.
- Format Table: Use the “Table Design” tab to choose styles and colors that enhance readability.
- Add Totals: If needed, check the box for “Total Row” to add a summary at the bottom.
Voila! You’ve created a sleek statistical table. Excel’s features allow for quick modifications, making it a handy tool for data presentation.
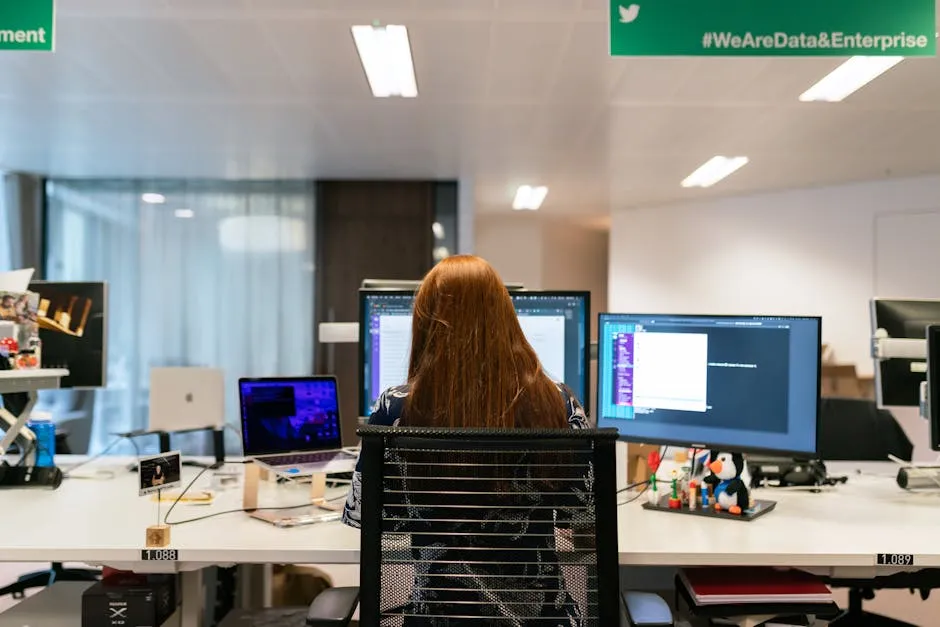
Conclusion
Statistical tables are more than just grids of numbers. They are powerful tools that transform raw data into meaningful insights. Throughout this article, we’ve discussed the importance of clarity and design in table creation. We explored how effective tables can facilitate comparison, enhance understanding, and support informed decision-making.
Remember, a well-designed statistical table should be clear and concise. It serves as a snapshot of your data, making it accessible to your audience. Whether you’re analyzing test scores or tracking economic trends, mastering the art of table design is crucial.
As you dive into your own research or work, don’t shy away from creating and utilizing statistical tables. They will not only aid your analyses but also enhance your presentations. In our data-driven world, the ability to present information effectively is invaluable. So, roll up your sleeves, embrace the power of statistical tables, and let your data tell its story.
FAQs
What is a statistical table?
A statistical table is a structured format that organizes data into rows and columns. It summarizes information, making it easier to interpret and compare. These tables are crucial for presenting complex data clearly and concisely.
How do I use a t-table?
To use a t-table, first determine your degrees of freedom based on your sample size. Next, select a significance level, typically 0.05. Finally, find the critical t-value in the t-table corresponding to your degrees of freedom and significance level. Compare this value to your calculated t-value to decide whether to reject the null hypothesis.
What types of data analysis require statistical tables?
Statistical tables are used across various fields, including economics, healthcare, marketing, and education. They are essential for summarizing data sets, presenting results of experiments, and facilitating comparisons in studies.
Can I create statistical tables in Excel?
Absolutely! Excel is user-friendly and allows you to create, format, and manipulate statistical tables easily. Simply input your data, select it, and use the “Insert Table” function to create a clear and organized table.
Where can I find examples of statistical tables?
You can find examples of statistical tables in research papers, government reports, and educational resources. Websites like the Reserve Bank of Australia and various academic journals often showcase well-designed statistical tables.
How do I interpret p-values in statistical tables?
P-values indicate the probability of observing your data given that the null hypothesis is true. A p-value below your chosen significance level (e.g., 0.05) suggests that the null hypothesis can be rejected, indicating a statistically significant result. Always consider the context of your analysis when interpreting p-values.
Please let us know what you think about our content by leaving a comment down below!
Thank you for reading till here 🙂
All images from Pexels