Introduction
Ratios in statistics are like the secret sauce of data analysis. They help us understand relationships between numbers, making them essential for informed decision-making. So, what exactly is a ratio in this realm? Simply put, a ratio compares two quantities, illustrating how much of one exists in relation to another. Imagine you’re at a party with friends. If there are 10 slices of pizza and 5 hungry friends, the ratio of pizza slices to friends is 10:5, or simplified, 2:1. This means each friend could have two slices if they shared equally. Understanding ratios is crucial because it allows analysts to make meaningful comparisons across different datasets, aiding in drawing conclusions and making predictions. Ratios find their utility in various contexts. Whether you’re analyzing financial metrics like profit margins, measuring performance indicators in business, or evaluating data scales in research, ratios play a pivotal role. They help clarify trends and relationships, enabling statisticians to extract insights from raw data. Throughout this article, we will dive into the critical aspects of ratios, including their definition, importance in data analysis, and the different types of ratios commonly used. By the end, you’ll appreciate how ratios can transform seemingly complex data into understandable and actionable insights. If you’re looking to deepen your knowledge, grab a copy of Statistics for Dummies—it’s a fantastic starting point!
Summary of Key Points
In the world of statistics, ratio data stands tall as a formidable type of quantitative data. It possesses unique characteristics that set it apart from other data types. One of the most notable traits is the true zero point, meaning that a value of zero indicates the absence of the variable being measured. This quality makes ratio data vital for accurate calculations, allowing for the use of all mathematical operations. Recognizing the characteristics of ratio data is important. Unlike nominal or ordinal data, which can’t express magnitude, ratio data can reveal how much more one value is than another. For instance, when measuring height or weight, zero signifies no height or weight, making these measurements meaningful. Real-world applications of ratio data are abundant. Think about your height, your income, or the number of hours you exercise weekly. Each of these examples illustrates how ratio data operates in everyday life. By understanding these applications, you can grasp the significance of using the right type of data for analysis. If you want to explore more about data modeling, check out The Data Warehouse Toolkit: The Definitive Guide to Dimensional Modeling. Moreover, ratio data is distinct from other data types. It stands on its own, with clear differences when compared to nominal, ordinal, and interval data. Being aware of these differences can enhance your analytical skills, enabling you to choose the appropriate data type for your research or business needs. As we progress through this article, we’ll unravel these concepts further. By the end, you’ll be equipped with the knowledge to effectively analyze ratio data, understand its implications, and apply it in various contexts. Get ready to unlock the full potential of ratios in statistics! And if you’re keen on brushing up your statistical knowledge, consider Principles of Statistics.
Understanding Ratio Data
Ratio data is the highest level of measurement in statistics. It’s like the VIP section of the data club! This type of data has a true zero point, allowing for meaningful calculations and comparisons. Think of it as the gold standard for quantitative data. Let’s break down what makes ratio data unique and how it compares to other data types.Characteristics of Ratio Data
One of the standout features of ratio data is its true zero point. This means that a value of zero indicates the complete absence of the variable being measured. For instance, if you measure weight, a weight of zero means there is no weight at all. In contrast, if you measure temperature in Celsius, zero doesn’t mean there’s no temperature—it’s just another point along the scale. Another characteristic is the equal intervals between values. This means the difference between, say, 10 and 20 kilograms is the same as the difference between 20 and 30 kilograms. In other words, the scale is consistent, allowing for reliable comparisons. Let’s not forget about negative values. Ratio data cannot contain negative numbers. You can’t have a negative height or weight! If someone claims to be -5 feet tall, they might need a reality check. Examples of ratio data include height, weight, speed, and duration. These measurements are not only quantifiable but also carry meaningful significance. And speaking of significance, if you’re looking for a practical guide to data science, The Art of Data Science is a must-read!
Comparison with Other Data Types
To truly appreciate ratio data, it’s essential to compare it with other types of data: nominal, ordinal, and interval. Nominal Data is the most basic type. It consists of categories without any order or ranking. Think of it as labels. An example would be colors like red, blue, and green. You can’t say one color is greater than another; they just exist. Ordinal Data is a step up. It introduces order but doesn’t specify the distance between the values. For instance, a rating scale of 1 to 5 gives you an idea of preference but doesn’t quantify the difference between, say, a 3 and a 4. Interval Data has equal intervals but lacks a true zero. A classic example is temperature in Celsius. Zero degrees doesn’t mean there’s no heat; it’s just a point on the scale. Now, what sets ratio data apart? It allows for meaningful calculations, including ratios and comparisons. You can say that a person weighing 80 kg is twice as heavy as someone weighing 40 kg. However, with interval data, such statements would be meaningless. In summary, ratio data is a powerful tool for analysis. It combines all the benefits of nominal, ordinal, and interval data while providing that all-important true zero. When conducting research or statistical analysis, understanding these differences is key to selecting the right type of data for your needs. If you want to dive deeper into statistical methods, consider Statistical Methods for the Social Sciences.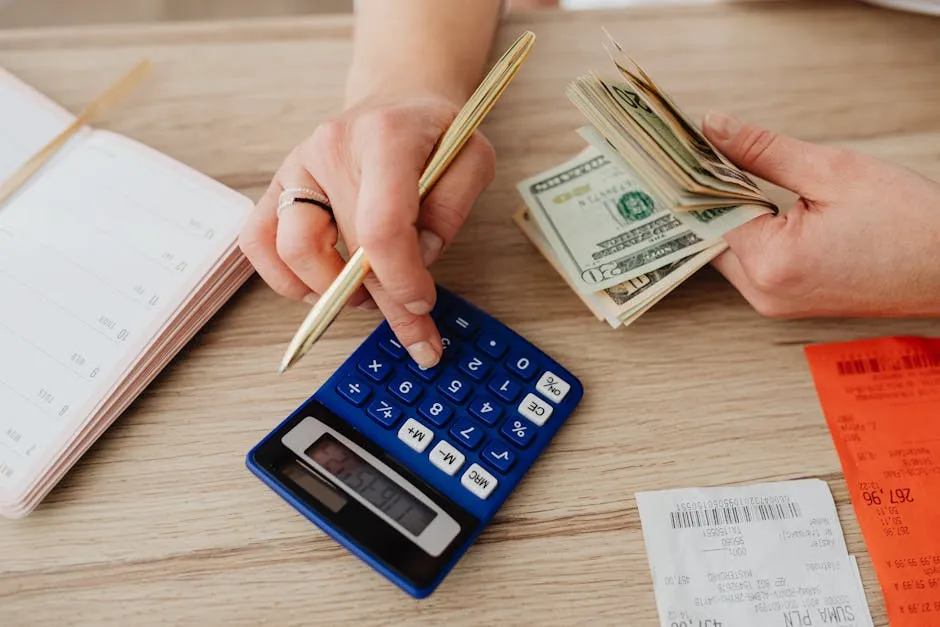
Techniques for Analyzing Ratio Data
Analyzing ratio data is crucial for deriving meaningful insights from quantitative measurements. This section will cover two primary methods: descriptive and inferential statistics, followed by effective data visualization techniques.Descriptive Statistics
Descriptive statistics summarize the central tendency and variability of ratio data. Here are the key components: 1. Mean, Median, Mode: – The mean is the average of all values. It provides a single representative value. – The median is the middle value when data is ordered. It’s useful for understanding the midpoint, especially when outliers skew the mean. For more insights on median calculations, check out this article on statistics poland median salary 2024.– The mode is the most frequently occurring value. While less common with continuous data, it can highlight peaks in datasets. 2. Measures of Variability: – Range: The difference between the maximum and minimum values. It offers a quick glance at the spread. For a deeper understanding of range in data, refer to this guide on orange county ca crime statistics.Understanding the median is vital for data analysis. Learn more about median statistics here.
– Standard Deviation: This statistic indicates how much individual data points deviate from the mean. A low standard deviation means data points are close to the mean, while a high standard deviation indicates more spread out data. – Variance: The square of the standard deviation, it quantifies the degree of variation in the data. These descriptive measures are essential for understanding your dataset before conducting more complex analyses. If you want to keep your data organized, consider using a Multi-Compartment Organizer to keep your workspace tidy!The range is essential for understanding data spread. Explore crime statistics for more insights.
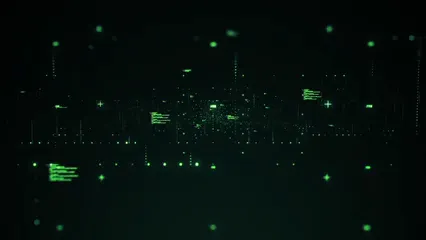
Inferential Statistics
Inferential statistics allow researchers to make conclusions about a population based on a sample. Here’s why these techniques matter: 1. Importance of Hypothesis Testing: – Hypothesis testing helps researchers determine if their findings are statistically significant. This process involves setting up a null hypothesis and an alternative hypothesis, then using data to support or reject these claims. For a comprehensive overview of hypothesis testing, check out our statistics hypothesis testing cheat sheet.2. Common Tests Applicable to Ratio Data: – T-tests: Used to compare the means of two groups. For instance, you might compare the average income of two different regions. – ANOVA (Analysis of Variance): This test is utilized when comparing the means of three or more groups. It helps determine if at least one group mean is statistically different from the others. – Regression Analysis: This technique explores the relationship between variables, helping predict outcomes based on independent variables. For example, it can be used to analyze how income influences spending habits. If you want to deepen your understanding of regression, consider checking out R for Data Science. These statistical tests provide a framework for making informed conclusions based on empirical data.Hypothesis testing is crucial for statistical analysis. Learn more about hypothesis testing here.
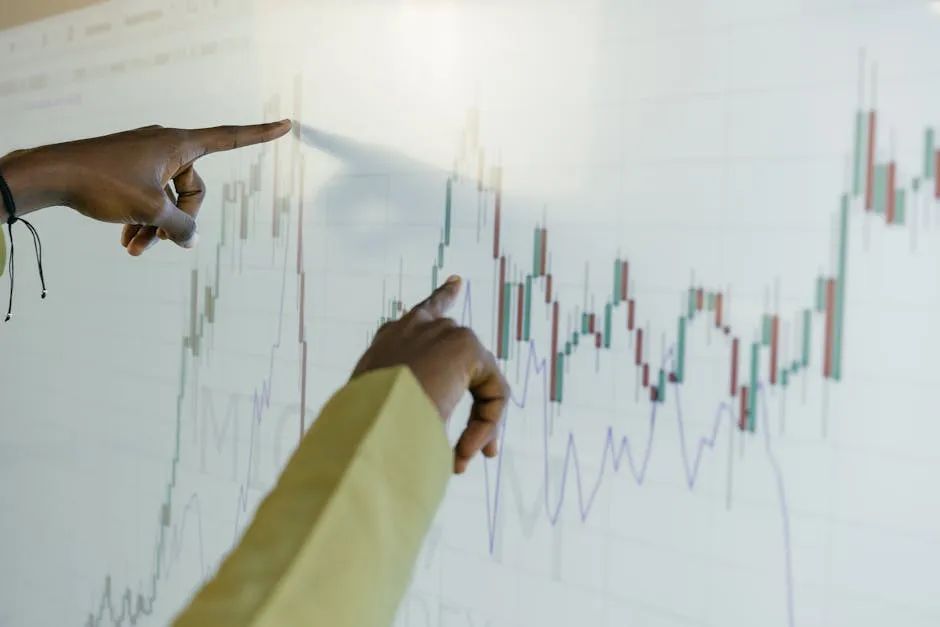
Visualizing Ratio Data
Effective data visualization is crucial for interpreting and communicating findings. Here’s how to visualize ratio data effectively: 1. Importance of Data Visualization: – Visualizations can simplify complex data, highlighting key trends and relationships that might not be immediately apparent in raw numbers. They allow for quick assessments and enhance the storytelling aspect of data analysis. 2. Types of Graphs Commonly Used with Ratio Data: – Histograms: These are useful for showing the frequency distribution of continuous data. They can reveal the shape of the data’s distribution (normal, skewed, etc.). – Scatterplots: Ideal for examining relationships between two continuous variables. They can indicate correlations and help identify outliers. – Box Plots: These provide a visual summary of data through their quartiles, showing the median, range, and potential outliers. Incorporating these visual tools not only aids in understanding but also enhances the communication of findings to various stakeholders. By using descriptive and inferential statistics alongside effective visualization techniques, you can unlock the full potential of your ratio data, leading to more informed decisions and insights. If you’re looking for a great way to visualize your data, check out the Graphing Calculator TI-84 Plus—it’s a classic tool for students and professionals alike!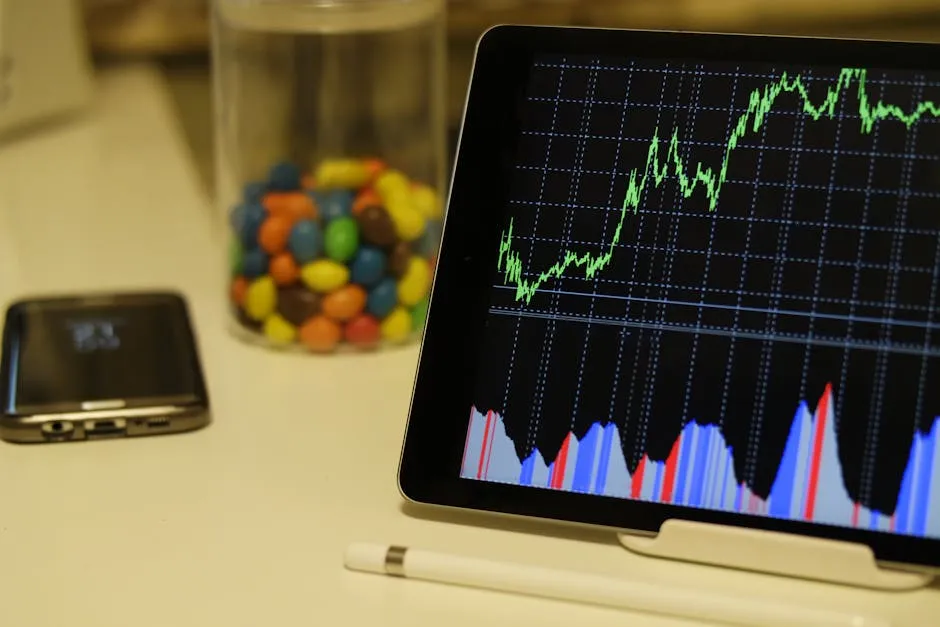
Frequently Asked Questions
What is the main difference between interval and ratio data?
Interval and ratio data share similarities, yet they are distinct. The key difference lies in the presence of a true zero. Ratio data has a zero point indicating the absence of a quantity. For example, a weight of zero means there is no weight. In contrast, interval data lacks this true zero. Take temperature: zero degrees Celsius doesn’t mean there’s no temperature; it’s just a point on the scale. Thus, you can perform meaningful calculations and comparisons with ratio data, such as saying one value is twice another, while with interval data, such ratios lose their significance.
Can ratio data be expressed as a percentage?
Absolutely! Ratio data can be transformed into percentages to better convey information. For instance, if you have a group of 100 participants, and 25 of them prefer coffee over tea, you can say that 25% of the group prefers coffee. This conversion is especially helpful in surveys and research when you want to present data clearly. Just remember, while percentages offer insights, maintaining the context of the original ratio data is essential for accurate interpretation.
What are some common errors to avoid when working with ratio data?
When handling ratio data, a few pitfalls can trip you up. One common mistake is misinterpreting the true zero. Always ensure you recognize that zero indicates the absence of the quantity. Another error is treating ratio data like interval data. Remember, with ratio data, you can conduct all arithmetic operations, including multiplication and division. Lastly, avoid ignoring the context of the data. Presenting percentages without understanding the underlying ratios can lead to misleading conclusions.
How do I know when to use ratio data in my analysis?
Choosing to use ratio data hinges on the nature of your variables. If you need to measure quantities that have a true zero and equal intervals, ratio data is your go-to option. Think of measurements like height, weight, or income—these all fit the bill! If your analysis requires comparisons, calculations of ratios, or meaningful averages, then ratio data is essential. Always assess your research questions and the type of data you’re collecting. The right data type can dramatically enhance your analysis and conclusions.
Conclusion
Understanding ratio data is a cornerstone of effective statistical analysis. By recognizing its unique characteristics, you can apply this knowledge in various fields, from finance to healthcare. The importance of ratio data lies in its ability to provide clear, quantitative insights, allowing for accurate comparisons and meaningful conclusions. Remember, ratio data is the most comprehensive measurement scale. It enables you to perform all mathematical operations, including ratios and percentages, enhancing your analytical capabilities. Embrace statistical methods and tools that facilitate the analysis of ratio data. If you’re interested in a broader understanding of data analysis, consider The Basics of Data Literacy to level up your skills! As you delve deeper into the world of statistics, consider exploring related topics such as descriptive statistics or inferential techniques. The more you understand about data types, the better equipped you’ll be to tackle your research questions. Keep learning, and let the power of ratio data elevate your analytical skills to new heights! And of course, don’t forget to take breaks with a good book; you might enjoy The Power of Habit for some insightful reading! Please let us know what you think about our content by leaving a comment down below! Thank you for reading till here 🙂All images from Pexels