Introduction
In today’s data-driven landscape, researchers often find themselves swimming in a sea of information. The real challenge? Making sense of it all. Fortunately, the University of Minnesota (UMN) stands as a guiding light for social researchers tackling the complexities of survey data analysis. With its rich resources and expert faculty, UMN equips you to turn raw survey data into meaningful insights.
At UMN, you won’t just learn how to crunch numbers. You’ll discover the art of transforming data into compelling narratives. From state-of-the-art statistical tools to proven methodologies, the university offers a treasure trove of options for analyzing survey data. Whether you’re a seasoned researcher or just starting out, the support available can be a game-changer in your quest for knowledge.
One of the standout resources at UMN is the University Survey & Assessment Services (USAS). They provide professional data analysis services tailored for various research needs. Imagine having experts at your fingertips, ready to help clarify those pesky data ambiguities!
But what about the software? UMN has you covered. With access to popular programs such as SPSS Statistics 27, SAS Software, and Microsoft Excel 2021, you can easily navigate through your data. Each software package is designed to meet different analytical needs, making it easier to tackle complex survey results.
Additionally, the Minnesota Student Survey (MSS) is a vital tool that highlights the experiences of students across the state. This comprehensive survey informs policy decisions in education and public health, proving the importance of well-analyzed data.
By embracing best practices in survey data analysis, you can enhance your research’s quality and relevance. This blog post will delve into essential techniques, the significance of statistical terminology, and effective reporting styles. So, fill your coffee cup and prepare to embark on a journey toward mastering survey data analysis at the University of Minnesota!
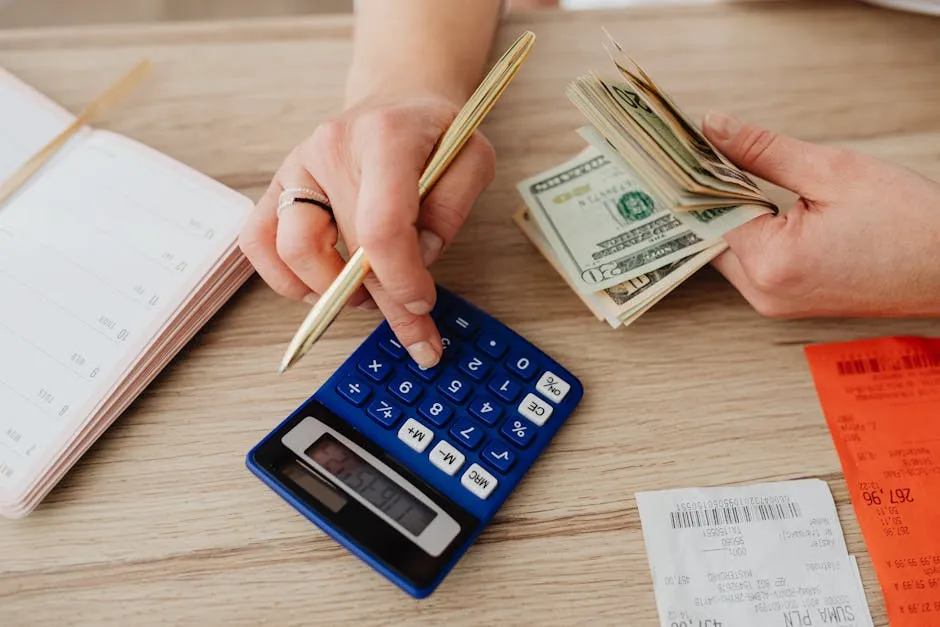
Summary
Understanding survey data is crucial for making informed decisions in social research. The University of Minnesota offers a wealth of resources, tools, and expertise for analyzing this data effectively. This article explores the best practices in survey data analysis, common statistical terms, popular software packages, and the intricacies of reporting findings. We will also touch on the significance of the Minnesota Student Survey (MSS) and how it informs public health and educational policies. With guidance from experienced faculty and access to cutting-edge software, researchers can navigate the complexities of data interpretation. Whether you’re a student, researcher, or educator, this comprehensive guide will spark your curiosity and provide practical insights to enhance your survey data analysis skills.
For a deeper understanding of statistical concepts, check out this summary statistics table.
Best Practices in Survey Data Analysis
Importance of Proper Data Analysis
Accurate data analysis is crucial in social research. It transforms raw data into meaningful insights, guiding critical decisions. Without proper analysis, researchers risk drawing incorrect conclusions, leading to misguided policy or program development.
Common challenges arise during data analysis. Data inaccuracies often stem from survey design flaws or respondent misunderstanding. For instance, poorly worded questions can lead to ambiguous responses. Additionally, inconsistent data entry can introduce errors. Even a small mistake can skew results, making it essential to prioritize accuracy throughout the analysis process.
Another hurdle is the overwhelming volume of data. As researchers, we can easily feel inundated by the sheer amount of information collected. Navigating through extensive data sets can lead to fatigue and oversight. Hence, developing a systematic approach is vital for effective data analysis.
By addressing these challenges, researchers can significantly enhance the quality of their findings. This, in turn, supports informed decision-making that benefits communities and advances social policies.

Steps in Analyzing Your Data
Data Review: Before analysis, cleaning and preparing data is essential. This involves checking for missing values, correcting entry errors, and ensuring consistency. A clean dataset sets the foundation for accurate analysis. Think of this step as polishing your diamond—you want it to shine bright without any flaws!
Software Tools: At the University of Minnesota, a variety of software options are available to assist you. Microsoft Excel is great for basic analysis and data organization. SAS offers a robust suite for advanced statistical analysis, while SPSS is user-friendly for those new to data analysis. Each tool serves a unique purpose, so choosing the right one can streamline your workflow.
Statistical Techniques: Familiarize yourself with the core statistical techniques. Descriptive statistics summarize data through measures like mean, median, and standard deviation. On the other hand, inferential statistics allow you to draw conclusions about a population based on a sample. Understanding these techniques is fundamental to effectively interpreting your data.
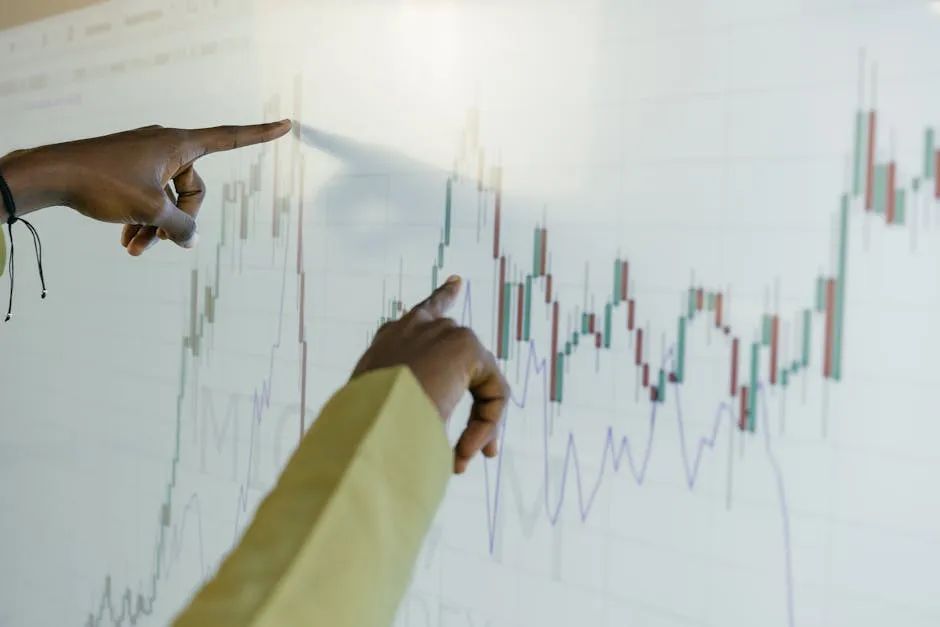
For a comprehensive look at statistical techniques, refer to this statistical techniques in business and economics.
Reporting Your Findings
Reporting findings is a critical step in the research process. A comprehensive report should include several key elements. Start with a title page and table of contents for easy navigation. Follow this with an executive summary that highlights the main findings. Don’t forget to provide context and detail your research methodology.
Different reporting styles cater to various audiences. For example, a transcription report is ideal for qualitative research, capturing participants’ verbal responses in detail. In contrast, a summary report is suited for quantitative data, presenting results with charts and tables. An executive report condenses information for quick insights, perfect for stakeholders who need the essentials without the fluff.
Ultimately, the style you choose should align with your audience’s needs. Whether it’s a lengthy academic paper or a concise executive summary, clarity and relevance are paramount. Remember, the goal is to communicate your findings effectively, ensuring they resonate with your readers.
Following these best practices will not only enhance the quality of your survey data analysis but also empower you to present your findings in a compelling and effective manner. With the right approach and tools, your research can make a significant impact in the field of social research.

Inferential Statistics
Inferential statistics is the branch of statistics that allows researchers to draw conclusions about a population based on sample data. Think of it as your trusty sidekick in the world of social research. This statistical approach helps in making predictions and generalizations, ensuring that the findings from a selected sample can be applied to a larger group.
For instance, consider a survey conducted among university students. By analyzing the responses from a sample, researchers can infer trends about all students at the university. If 70% of the surveyed students express satisfaction with their college experience, inferential statistics can suggest that a similar percentage likely feels the same across the broader student body.
Another classic example is predicting voting behavior based on poll data. If a survey shows that a significant portion of respondents favor a particular candidate, inferential statistics can help predict the overall election outcome. This process is crucial, especially when it comes to social research that informs policy decisions.
In summary, inferential statistics plays a vital role in social research. It enables scholars to estimate population parameters, test hypotheses, and draw meaningful conclusions from sample data, ultimately guiding decision-making in various fields.
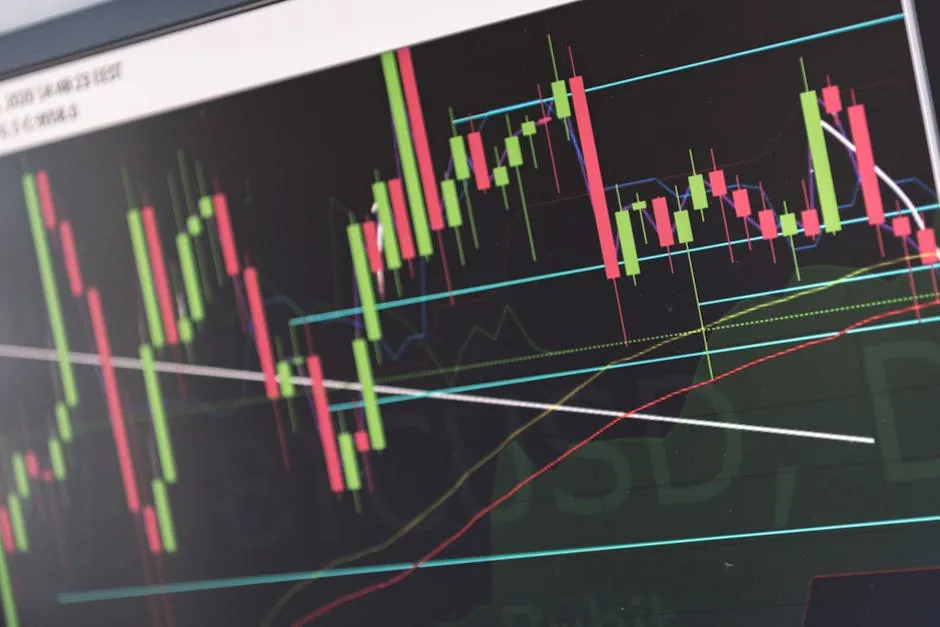
Popular Data Analysis Packages at UMN
Overview of Tools
When it comes to analyzing survey data at the University of Minnesota, several software packages stand out. Each tool has unique features that cater to different analytical needs. Here’s a snapshot of some of the most popular options:
Program | Key Features |
---|---|
Microsoft Excel | Basic data analysis, data organization, and visualization tools. |
SAS | Advanced statistical analysis, data manipulation, and complex modeling features. |
SPSS | User-friendly interface for statistical analysis, widely used in social science research. |
AMOS | Structural equation modeling and analysis of covariance structures. |
HLM | Specializes in analyzing nested data structures, such as students within schools. |
PRELIS / LISREL | Conducts structural equation modeling and related analyses. |
Mplus | Versatile tool for factor analysis, SEM, and latent variable modeling. |
Each software has its strengths and weaknesses. For example, while Excel is great for beginners, it may lack the advanced statistical capabilities offered by SAS or SPSS. SPSS, with its intuitive interface, is often favored by researchers who prefer a straightforward approach, but it might not handle large datasets as efficiently as SAS. Meanwhile, Mplus stands out for its flexibility in modeling complex relationships but may require a steeper learning curve.
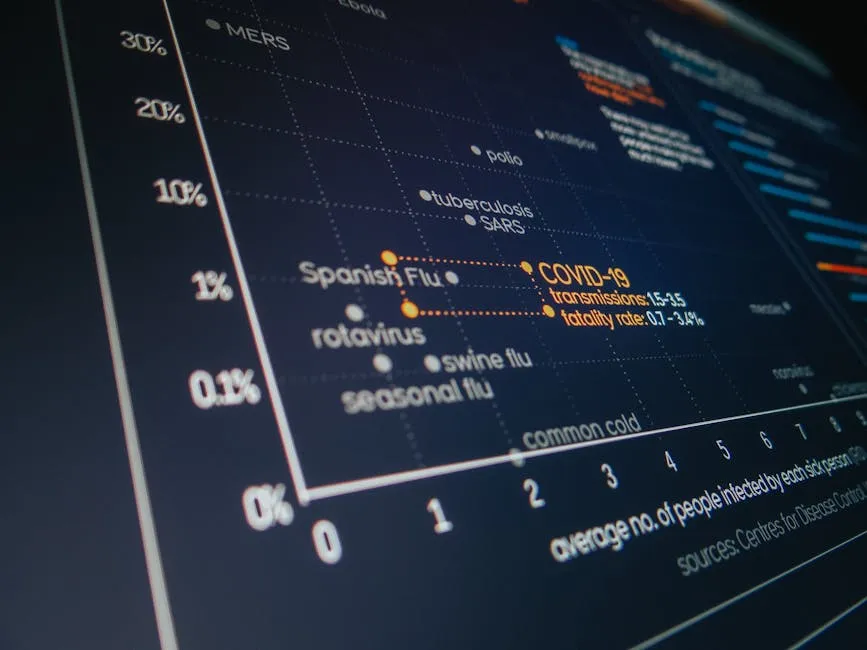
Case Studies
Numerous research projects at UMN have successfully employed these tools for data analysis. For instance, a recent study on student mental health utilized SPSS to analyze survey responses, revealing crucial insights about the factors affecting student well-being. Another project examined educational disparities using SAS, demonstrating the software’s capability in handling large datasets and complex statistical models. These case studies illustrate how the right tools can significantly impact the quality of research outcomes and policy recommendations.
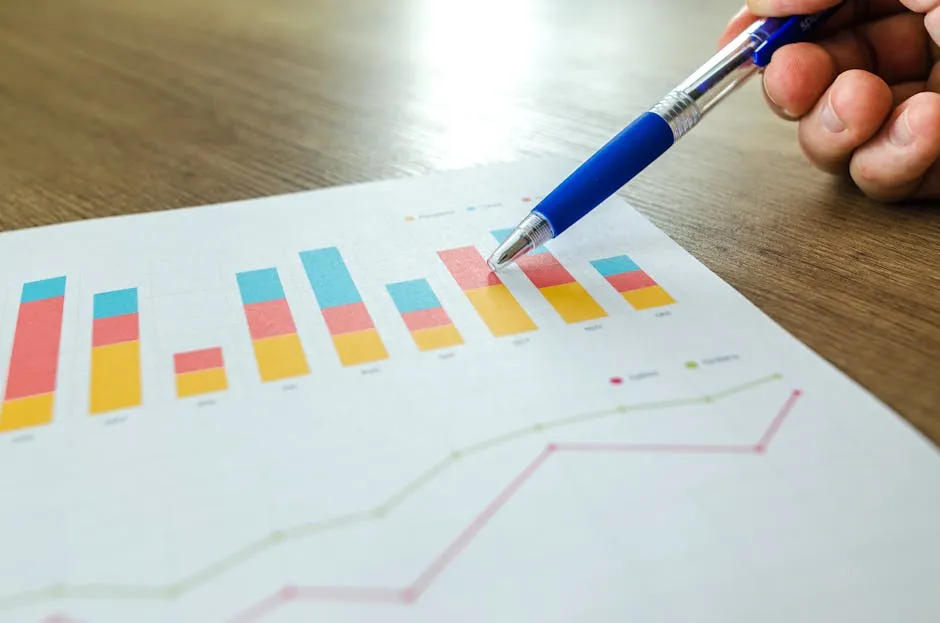
The Minnesota Student Survey (MSS) and Its Impact
Overview of the MSS
The Minnesota Student Survey (MSS) is a cornerstone of youth research in the state. Launched in 1989, it stands as one of the longest-running youth surveys in the nation. Conducted every three years, the MSS gathers anonymous responses from students across grades five, eight, nine, and eleven.
This survey aims to provide insights into students’ experiences, behaviors, and attitudes. Key areas of focus include health behaviors, educational experiences, and social issues. By analyzing trends over time, researchers can identify shifts in youth behavior and inform public policy.
With its comprehensive approach, the MSS plays a crucial role in shaping educational and health policies in Minnesota. The data collected helps stakeholders understand the challenges faced by students, ranging from mental health issues to substance use. By addressing these concerns, policymakers can create targeted interventions that support student well-being.
In summary, the Minnesota Student Survey not only serves as a vital research tool but also significantly impacts public health and education policy. Its findings help ensure that the voices of Minnesota’s youth are heard and considered in decision-making processes, ultimately contributing to a healthier and more supportive environment for students across the state.

Analysis of MSS Data
Researchers looking to access and analyze Minnesota Student Survey (MSS) data have several pathways. First, they can visit the Minnesota Department of Education’s website. There, they’ll find a treasure trove of resources, including downloadable PDF tables and interactive online reports. These reports offer insights into the experiences of Minnesota students across various grades.
Additionally, the MSS data includes specific details about health behaviors and educational experiences, making it a goldmine for public health and education policy analysis. To access school-level results or individual-level data files, researchers can complete the MSS Data Request Form. This allows for deeper statistical analysis tailored to specific research questions.
Understanding the implications of MSS findings is crucial for shaping public health and education policy. For instance, the data highlights trends in youth behaviors, such as substance use or mental health issues. By analyzing these trends, policymakers can craft targeted interventions that address the unique challenges faced by students.
Moreover, the MSS findings can guide resource allocation in schools. For example, if data reveals a spike in mental health concerns among high school students, schools can implement counseling programs or workshops. This proactive approach ensures that resources are directed where they’re needed most.
In summary, the MSS is more than just a survey; it’s a key tool for informed decision-making in education and health sectors. By leveraging this data, researchers and policymakers can enhance student well-being and create a more supportive learning environment.
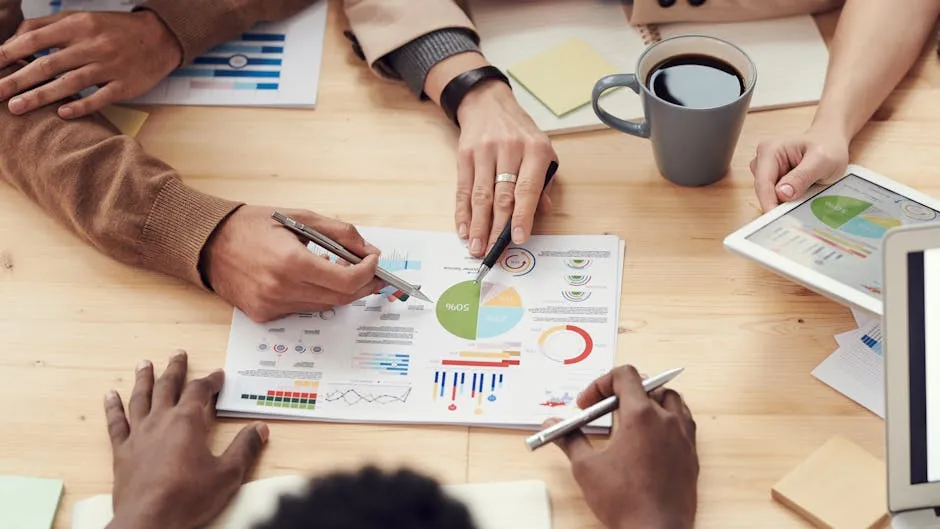
Conclusion
As we conclude our exploration of the University of Minnesota’s resources for analyzing survey data in social research, it’s clear that the right tools and knowledge can significantly enhance the quality of your findings. By familiarizing yourself with best practices, commonly used statistical terms, and the various software packages available, you can confidently engage in data analysis that informs important social issues.
Whether you’re conducting research for academic purposes or influencing policy decisions, the skills and insights gained from this guide will empower you to make data-driven decisions that resonate within your field. For example, mastering software like SPSS or SAS can streamline your analysis process. You’ll not only analyze data but also visualize it effectively, making your findings more impactful.
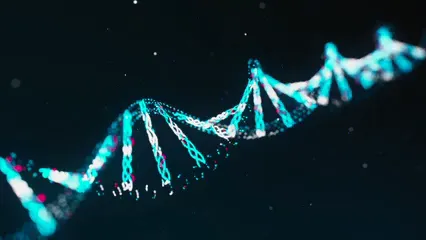
For troubleshooting software like SPSS, you can refer to this macos ibm spss statistics fix 28.1.1.
Moreover, understanding statistical techniques is crucial. Descriptive statistics help summarize your data, while inferential statistics allow you to draw broader conclusions. Knowing when to apply each technique can elevate your research.
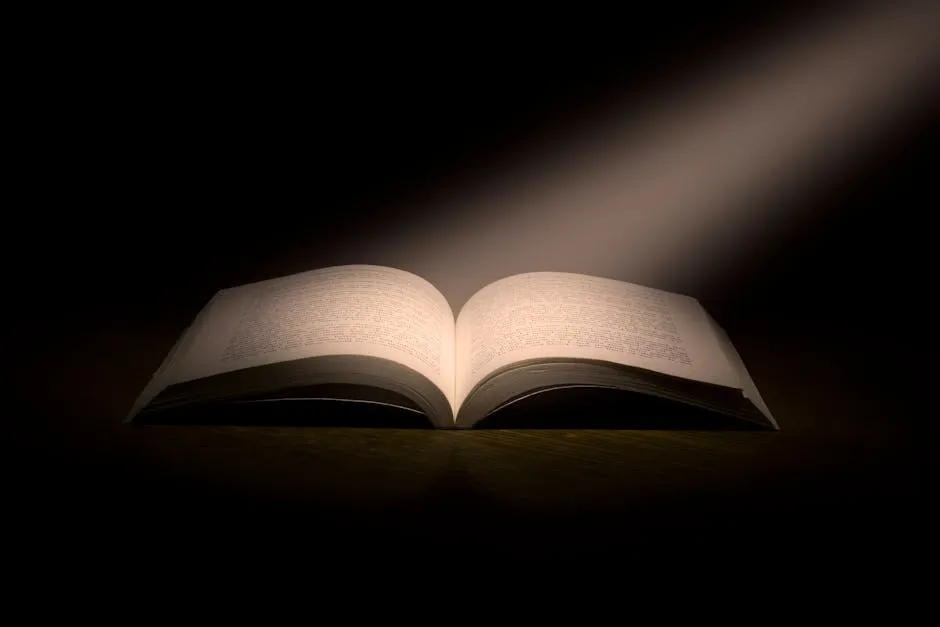
To learn about the challenges of inferential statistics, refer to this the problem with inferential statistics.
Incorporating insights from the Minnesota Student Survey (MSS) further amplifies your impact. This survey offers a snapshot of student experiences, which can inform crucial policy decisions. By analyzing MSS data, you can identify trends and advocate for changes that improve student health and educational outcomes.
Remember, research is about more than numbers. It’s about storytelling—crafting narratives that highlight the realities of social issues. Engaging effectively with data ensures that your research speaks volumes.
In short, the tools and knowledge available at the University of Minnesota empower researchers to tackle pressing social challenges. Embrace these resources, and you’ll be well on your way to making a meaningful difference in your field. So, keep learning, stay curious, and let your data tell its story!
FAQs
What statistical software is recommended for beginners?
If you’re just starting your journey in data analysis, user-friendly software can make a world of difference. Microsoft Excel is a fantastic option for beginners. It’s widely accessible and allows for basic data organization and analysis. You can create charts and tables with a few clicks. Plus, who doesn’t love those colorful spreadsheets? Another great choice is SPSS (Statistical Package for the Social Sciences). This software is specifically designed for statistical analysis and is incredibly intuitive. SPSS provides a menu-driven interface, making it easier for newbies to navigate without getting lost in technical jargon. Think of it as training wheels for your data analysis bike!
How can I ensure the accuracy of my survey data?
Ensuring the accuracy of your survey data is crucial. First, clean your data! Check for missing values, outliers, and entry errors. It’s like tidying up your closet before a big event—nobody wants to pull out a shirt with a stain! Next, implement validation techniques. Consider using logical checks to confirm that responses make sense. For instance, if someone claims to be 300 years old, it’s time for a double-check! Finally, conducting a pilot test with a small group can help identify potential issues before you launch your survey. Remember, a good foundation leads to solid results.
Where can I find additional resources for learning about statistics?
The University of Minnesota offers a wealth of resources for students eager to learn statistics. Start with online courses through platforms like Coursera or edX. Many universities partner with these platforms to provide quality content. Additionally, UMN hosts workshops throughout the year focused on statistical methods and software tools. Check out the University Survey & Assessment Services (USAS) for specialized training sessions. You can also explore their library’s digital resources for books and articles on statistics—think of it as an all-you-can-read buffet for your brain!
Can I access past Minnesota Student Survey data?
Absolutely! If you’re looking to analyze past Minnesota Student Survey (MSS) data, you’re in luck. The Minnesota Department of Education provides access to results from previous survey years. Simply visit their website to find interactive reports and downloadable tables. For more detailed analysis, you can request individual-level data files. Just fill out the MSS Data Request Form and User Agreement. It’s a straightforward process, so you can get back to crunching those numbers in no time! This access allows researchers to examine trends over the years, providing invaluable insights into student experiences across Minnesota.
Please let us know what you think about our content by leaving a comment down below!
Thank you for reading till here 🙂
All images from Pexels