Introduction
Data visualization is crucial in statistics. It transforms raw data into visuals that everyone can understand. After all, a picture can often express what words cannot. In AP Statistics, students learn how to represent data visually, making it easier to grasp complex concepts.
AP Statistics provides students with essential tools for analyzing and interpreting data. The formula sheet is a vital resource that consolidates key formulas and concepts. It serves as a quick reference guide during studies and exams, helping students navigate through various statistical analyses.
This article aims to provide techniques for visualizing data using AP Statistics concepts and formulas. We’ll explore how to leverage the AP Statistics formula sheet effectively to create meaningful visualizations. By incorporating these techniques, students can enhance their understanding and communication of statistical data, making their analyses more impactful.
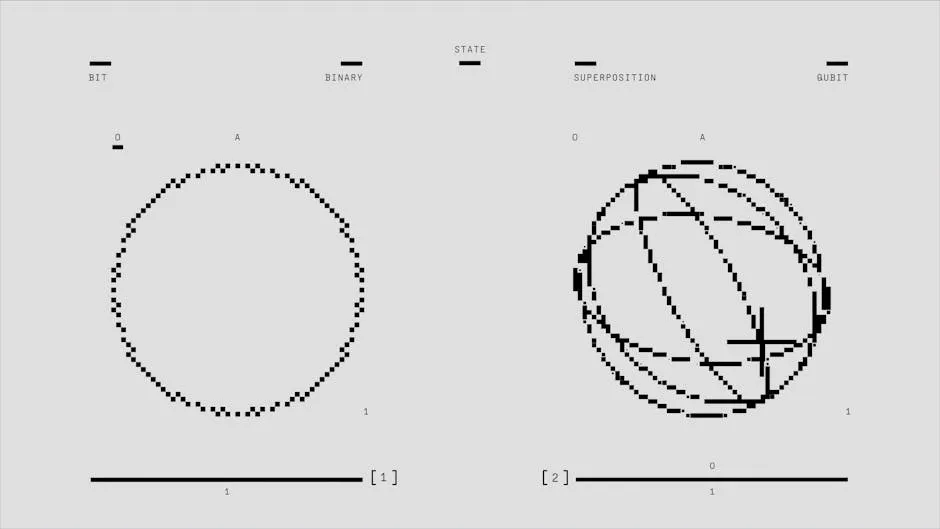
Understanding the AP Statistics Formula Sheet
What is the AP Statistics Formula Sheet?
The AP Statistics formula sheet is an essential tool for students preparing for the AP exam. It compiles critical formulas and tables from various units of the course. This sheet is designed to aid students during both multiple-choice and free-response sections of the exam.
On the formula sheet, students will find formulas for descriptive statistics, probability distributions, and inferential statistics. These components are crucial for analyzing data, interpreting results, and making informed decisions based on statistical evidence. Understanding the significance of each formula allows students to apply them confidently in different scenarios.
Moreover, the formula sheet includes probability tables for normal and t-distributions. Familiarity with these tables helps students quickly access necessary probabilities during the exam. Therefore, mastering the formula sheet is essential for success in AP Statistics. It not only aids in exam preparation but also fosters a deeper understanding of statistical concepts.
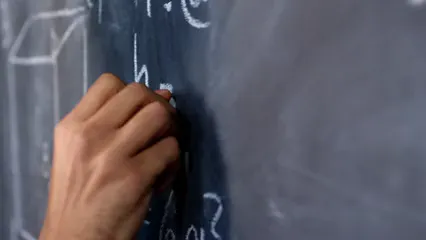
Key Sections of the Formula Sheet
Descriptive Statistics
Descriptive statistics are the foundation of data analysis. They summarize data sets with key measures. The formula sheet provides essential formulas for calculating the mean, median, mode, and standard deviation.
Mean: The average of a data set is calculated by summing all values and dividing by the number of observations. It’s a go-to measure for central tendency. For further insights on this concept, check out what does mean identically distributed in statistics.
Median: This value divides a sorted data set into two equal halves. If the dataset has an even number of observations, the median is the average of the two middle numbers. For a deeper understanding, refer to statistics Poland median salary 2024.
Mode: The mode represents the most frequently occurring value in a dataset. A dataset can be unimodal (one mode), bimodal (two modes), or multimodal (multiple modes).
Standard Deviation: This formula measures the variability of data points from the mean. A low standard deviation indicates that data points are close to the mean, while a high standard deviation indicates greater dispersion.
Understanding these concepts helps interpret data accurately and lays the groundwork for more advanced statistical techniques.
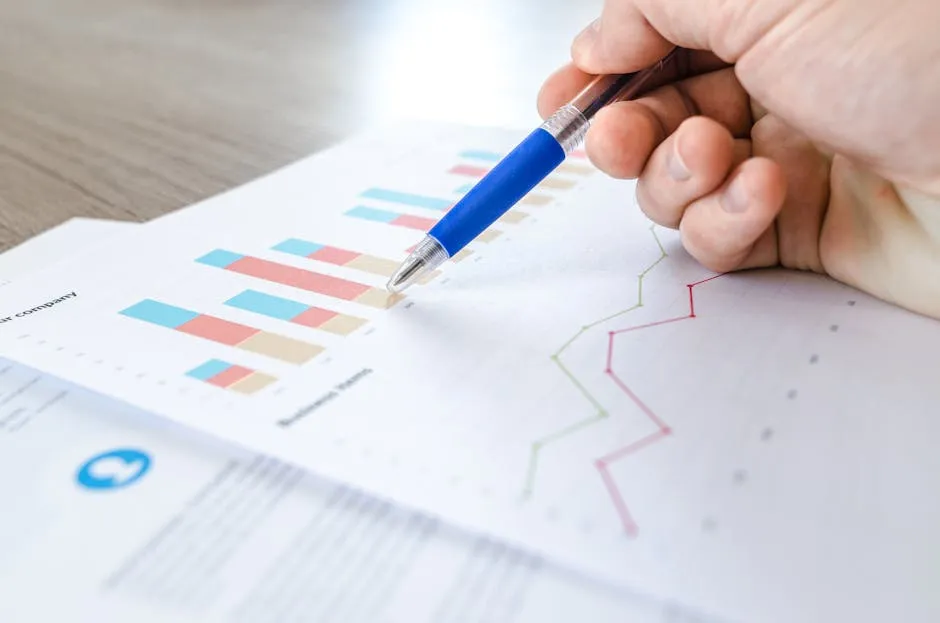
Probability and Distributions
Probability is a key element in statistics, allowing us to measure uncertainty. The formula sheet contains crucial formulas for various distributions.
Binomial Distribution: This distribution applies to scenarios with two outcomes, such as success or failure. The formula calculates the probability of getting exactly x successes in n trials.
Normal Distribution: This continuous probability distribution is vital for many statistical methods. It’s characterized by its bell-shaped curve, defined by the mean and standard deviation. The area under the curve represents probabilities.
The sheet also includes formulas for calculating the mean and standard deviation for both binomial and normal distributions. These tools are essential for making predictions and understanding variability.
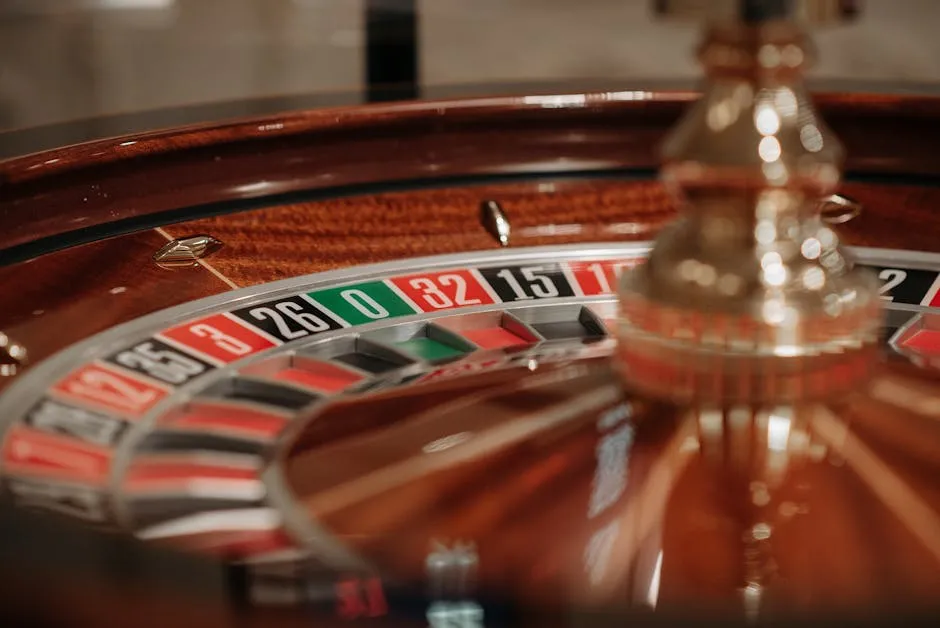
Inferential Statistics
Inferential statistics allow us to make conclusions about populations based on sample data. This section of the formula sheet covers key formulas for confidence intervals and hypothesis testing. For more insights, see the problem with inferential statistics.
Confidence Intervals: A confidence interval estimates a range within which a population parameter lies. The formula incorporates the sample statistic, critical value, and standard error.
Hypothesis Testing: This involves testing assumptions about population parameters. The test statistic helps determine if the null hypothesis can be rejected in favor of the alternative hypothesis.
These inferential statistics tools enable researchers to draw meaningful conclusions and assess the reliability of their findings, making them crucial for data-driven decision-making.
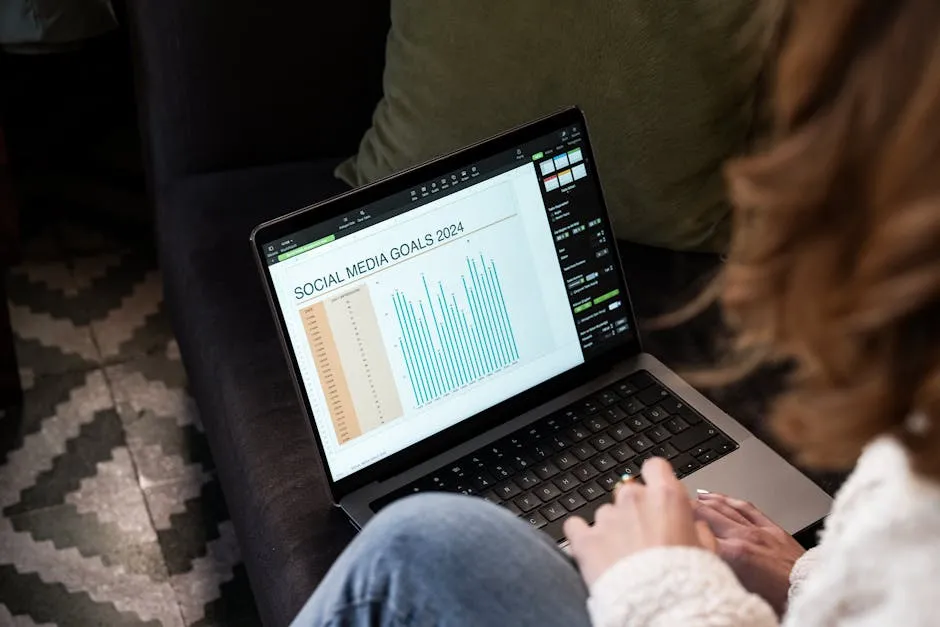
Common Visualization Techniques
Bar Charts
Bar charts are perfect for visualizing categorical data. They present data in rectangular bars, where the length of each bar corresponds to the category’s value. Use bar charts when you want to compare different categories easily. For example, if you survey friends about their favorite ice cream flavors, a bar chart can clearly show how many prefer chocolate, vanilla, or strawberry.
Imagine a survey where 10 friends respond with their favorite flavors:
Flavor | Count |
---|---|
Chocolate | 4 |
Vanilla | 3 |
Strawberry | 2 |
Mint | 1 |
This data can be visualized in a bar chart, making it easy to see that chocolate reigns supreme. And if you want to color your bar chart like a pro, consider using a Colorful Markers Set to make your visuals pop!
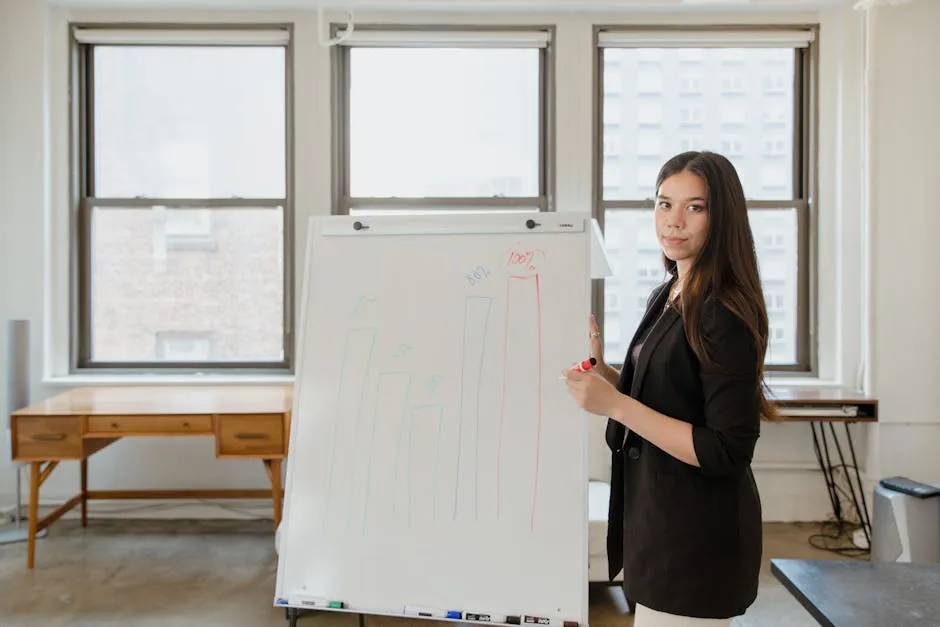
Histograms
Histograms are a go-to for displaying the distribution of quantitative data. They group data into bins or intervals, allowing you to see the frequency of data points in each bin. When you want to analyze the distribution of test scores, for instance, histograms can reveal how many students scored within certain ranges.
To create a histogram, follow these steps:
- Collect your data: Let’s say you have exam scores for 30 students.
- Define your bins: For example, 0-10, 11-20, 21-30, etc.
- Count the frequencies: Tally how many scores fall into each bin.
- Draw the axes: The horizontal axis represents the score intervals; the vertical axis represents frequency.
- Draw bars: Each bar represents the frequency of scores within a particular range.
A sample histogram might reveal that most students scored between 61 and 70, providing a clear visual of performance distribution. And to make those calculations easier, consider a Histogram Maker Tool that can simplify the process!
Box Plots
Box plots are fantastic for displaying the spread of data and identifying outliers. They show the median, quartiles, and potential outliers in a dataset. When you want to compare data distributions, box plots can be incredibly insightful.
Creating a box plot involves these steps:
- Gather your data: For example, scores from two different classes.
- Calculate the quartiles: Determine Q1 (25th percentile), Q2 (median), and Q3 (75th percentile).
- Identify outliers: Any data points beyond 1.5 times the interquartile range (IQR) from the quartiles should be marked.
- Draw the box: Create a box from Q1 to Q3 with a line at the median (Q2).
- Add whiskers: Extend lines from the box to the minimum and maximum values that aren’t outliers.
For instance, if Class A has a median score of 75 and Class B has a median of 85, the box plot will visually represent these differences, helping you see which class performed better overall. And if you’re looking for an efficient way to create box plots, a Box Plot Maker Tool might just be your new best friend!
Scatter Plots
Scatter plots are the superheroes of data visualization. They show relationships between two quantitative variables, making it easy to spot trends and correlations. By plotting individual data points on a two-dimensional graph, you can determine how one variable influences another.
For example, imagine you’re analyzing the relationship between study hours and test scores. Each student’s study hours could be on the x-axis, while their test scores sit on the y-axis. If the points trend upward, congratulations! You’ve uncovered a positive correlation: more study hours lead to higher test scores. Conversely, a downward slope suggests that as one variable increases, the other decreases.
A clear scatter plot can turn a tangled mess of numbers into an insightful visual. For instance, consider a scatter plot illustrating the correlation between daily exercise in minutes and weight loss in pounds. The points might cluster together, forming a line that slopes downward, indicating that increased exercise often correlates with greater weight loss. Now that’s a visual that speaks volumes! And to help you visualize your data with clarity, a Scatter Plot Maker Tool can be invaluable!
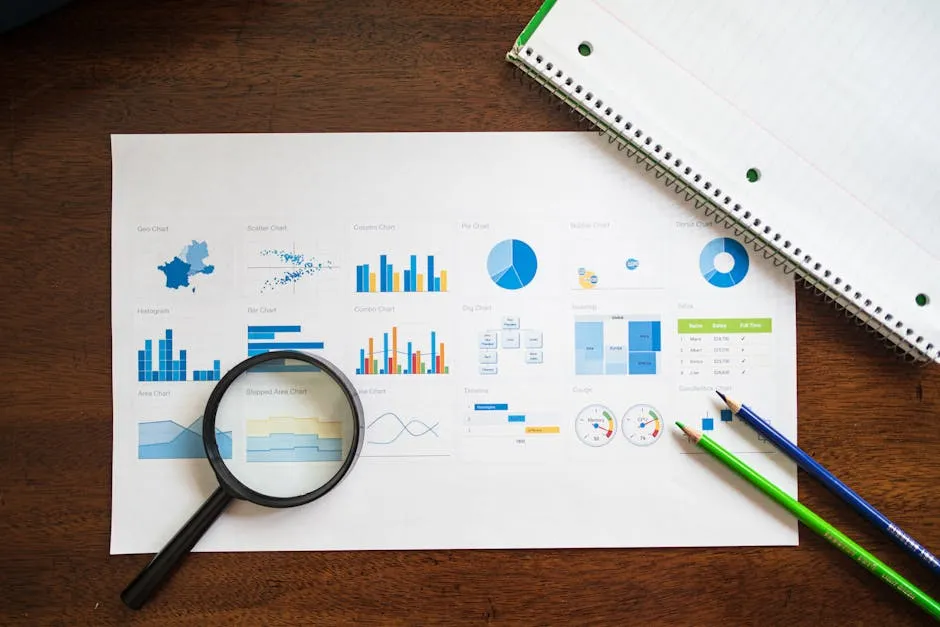
Advanced Visualization Techniques
Heatmaps
Heatmaps are a fantastic way to visualize complex data relationships. They transform quantitative data into a color-coded matrix, allowing you to quickly assess patterns and correlations across multiple variables. Each cell in the heatmap represents a value, and color intensity indicates how high or low that value is.
For example, imagine analyzing a dataset that records student performance across various subjects. A heatmap can show how well students scored in each subject, with darker colors representing higher scores. This method provides an instant snapshot of performance gaps and strengths in different subjects, enabling educators to tailor their teaching strategies effectively. And if you want to create your own heatmaps, a Data Visualization Toolkit Book is a great resource!
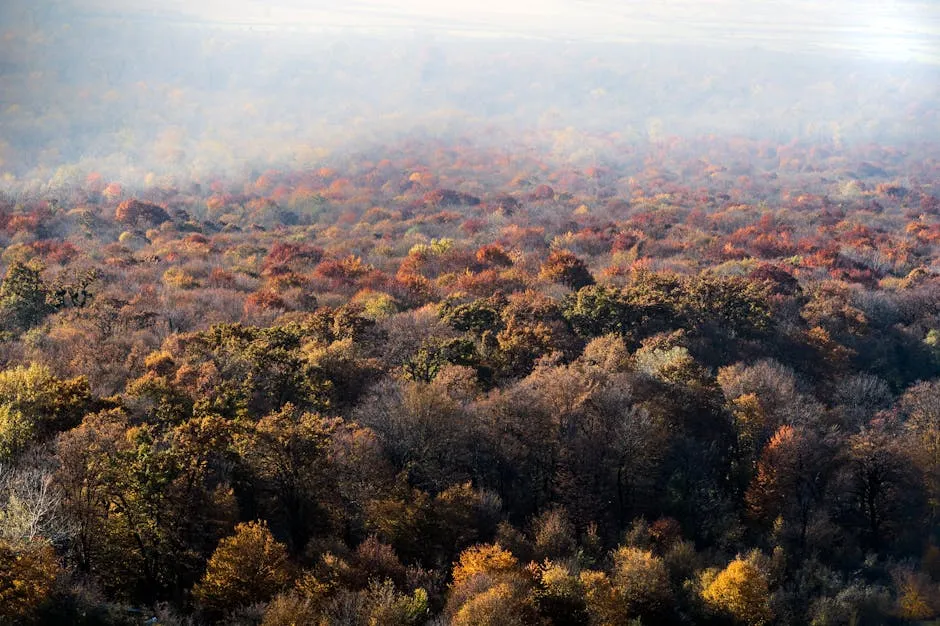
Density Plots
Density plots serve as a sleek alternative to histograms for revealing distribution shapes. Instead of using bars, density plots use smooth curves to illustrate the data’s distribution. They help visualize the probability density of a variable over a continuous range, making it easier to see where data points cluster.
When to use density plots? If you want to compare distributions between groups, density plots shine. For instance, comparing the test scores of two different classes can be easily achieved with a density plot. Instead of two separate histograms, a single plot overlays both distributions. You can clearly see which class performed better, examining the peaks and spread of scores without the clutter of overlapping bars.
In essence, these advanced techniques—scatter plots, heatmaps, and density plots—are essential tools for any AP Statistics enthusiast. They bring data to life, making complex relationships understandable and visually appealing. So, grab your data and start visualizing! And to stay organized while you do, consider a Student Planner for Organizing Study Time to manage your schedule!
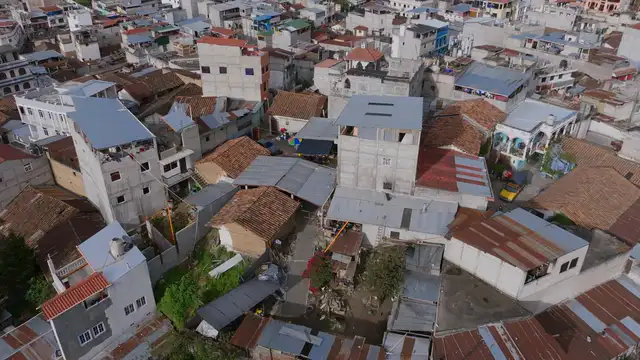
Case Studies and Examples
Real-world examples of visualizing data using AP Statistics techniques can significantly enhance comprehension. Let’s look at a few case studies that illustrate how students can apply the discussed visualization methods.
One compelling example involves analyzing the impact of study habits on student performance. Suppose a teacher collects data on hours studied versus exam scores from a class of 30 students. By using scatter plots, the teacher can visually represent the relationship between study hours and scores. Each point on the scatter plot corresponds to a student, showing how increased study time tends to correlate with higher exam scores. This visual representation makes it easy for students to grasp the positive correlation without wading through complex numbers.
Another example could be a survey on students’ favorite extracurricular activities. Imagine the data reveals preferences for sports, music, art, and theater. A bar chart can effectively display this categorical data, allowing students to see which activities are most popular at a glance. Each bar’s height represents the number of students who favor each activity, transforming dry numerical data into an engaging visual format. And to keep track of all these activities, a Educational Board Games related to Math can make learning fun!
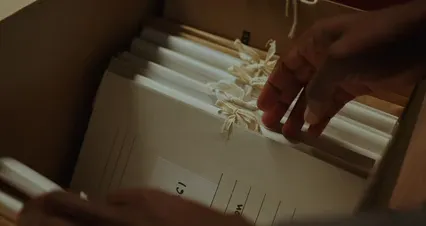
In addition, consider a study on the distribution of heights in a school. By collecting height data and using box plots, students can visually compare the median heights and identify any outliers. This method provides a clear summary of the data’s central tendency and variability, making it easy to see how heights differ among students.
Students can practice these techniques by utilizing sample data sets available online. By applying bar charts, scatter plots, and box plots to various datasets, they can reinforce their understanding of AP Statistics concepts. Visualizing data not only aids comprehension but also enhances communication of statistical findings. And while you’re at it, don’t forget to grab a Graph Paper Notebook to sketch out your ideas!
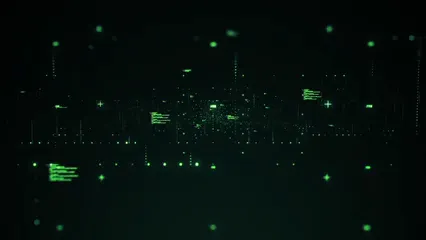
Using Software and Tools
When it comes to creating statistical visualizations, several powerful tools are at your disposal. R, Python, and Excel are among the most widely used software for generating high-quality graphics. For an introduction to using Python for statistical learning, refer to an introduction to statistical learning with Python book length.
Let’s focus on R, specifically the ggplot2 package, which is popular for its versatility and user-friendly syntax. To create a scatter plot using ggplot2, you can follow these simple steps:
- Install and load ggplot2: Make sure you have the package installed. You can do this by running
install.packages("ggplot2")
. Then, load it usinglibrary(ggplot2)
. - Prepare your data: Create a data frame with your variables. For example:
data <- data.frame(
study_hours = c(1, 2, 3, 4, 5),
exam_scores = c(68, 75, 80, 85, 90)
)
- Plot the data: Use the ggplot function to create your scatter plot.
ggplot(data, aes(x = study_hours, y = exam_scores)) +
geom_point() +
labs(title = "Study Hours vs. Exam Scores",
x = "Hours Studied",
y = "Exam Score")
- Customize your plot: You can enhance the visualization with colors, labels, and themes. For example:
ggplot(data, aes(x = study_hours, y = exam_scores)) +
geom_point(color = "blue", size = 3) +
geom_smooth(method = "lm", se = FALSE, color = "red") +
labs(title = "Study Hours vs. Exam Scores",
x = "Hours Studied",
y = "Exam Score") +
theme_minimal()
By following these steps, students can create appealing scatter plots that effectively communicate relationships in their data. Other tools like Python’s Matplotlib and Excel can follow similar principles, allowing students to visualize data effectively. And while you’re customizing your visuals, consider using Graphing Calculator (TI-84 Plus) for quick calculations!
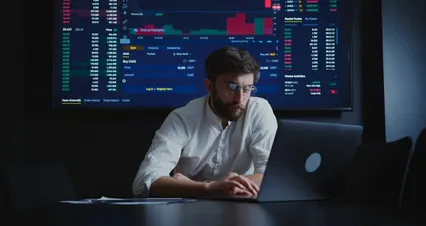
Conclusion
Visualizing data in AP Statistics is not just an academic exercise; it is an essential skill that enhances understanding and communication of statistical concepts. Through techniques like scatter plots, bar charts, and box plots, students can transform complex data into accessible visuals.
Mastering these visualization techniques is vital for success on the AP exam. By regularly practicing with the AP Statistics formula sheet, students can familiarize themselves with the formulas and concepts critical for analysis. The ability to visualize data effectively can significantly improve comprehension of statistical relationships and trends.
Encouraging students to practice these skills will not only prepare them for their exams but also equip them with tools they can utilize beyond the classroom. Understanding how to visualize data will undoubtedly enhance their performance in AP Statistics and other analytical disciplines. And don’t forget to keep hydrated while studying with a Water Bottle for Staying Hydrated While Studying!
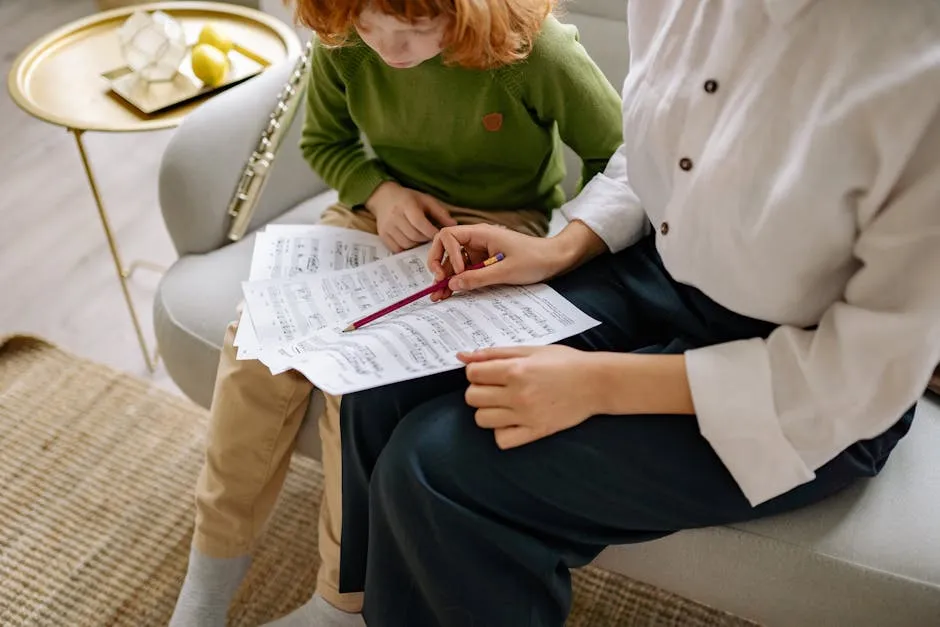
FAQs
What is the best way to prepare for the AP Statistics exam?
To excel in AP Statistics, students should focus on understanding the formula sheet and practicing visualization techniques. Reviewing past exam questions and engaging in group study sessions can also be beneficial. Make flashcards for key terms and concepts, and take practice tests under timed conditions to build confidence.
How can I improve my data visualization skills?
Improving data visualization skills requires practice and exploration of various resources. Online courses, textbooks, and tutorials for software like R and Python can offer valuable insights. Websites like Coursera and Khan Academy provide excellent courses on data analysis and visualization techniques.
Are there any common mistakes to avoid in data visualization?
Yes! Common pitfalls include creating misleading graphs, using inappropriate scales, and failing to label axes clearly. Always ensure that your visualizations accurately represent the data and provide context. Avoid cluttering visuals with unnecessary information, and focus on clarity to effectively communicate your message.
Please let us know what you think about our content by leaving a comment down below!
Thank you for reading till here 🙂
All images from Pexels