Introduction
Elementary statistics is the study of collecting, analyzing, interpreting, and presenting data. Think of it as the GPS for navigating through the world of numbers. Whether you’re in business, healthcare, or government, statistics helps you make sense of the chaos.
In business, statistics informs decisions on market trends and customer satisfaction. In healthcare, it plays a crucial role in clinical trials and understanding disease patterns. Government agencies use statistical data for everything, from census surveys to policy-making.
The objective of this article is to provide a comprehensive overview of elementary statistics. We’ll break down its core concepts, importance, and applications in real life. So, grab a snack and get ready to tackle the fascinating world of numbers!
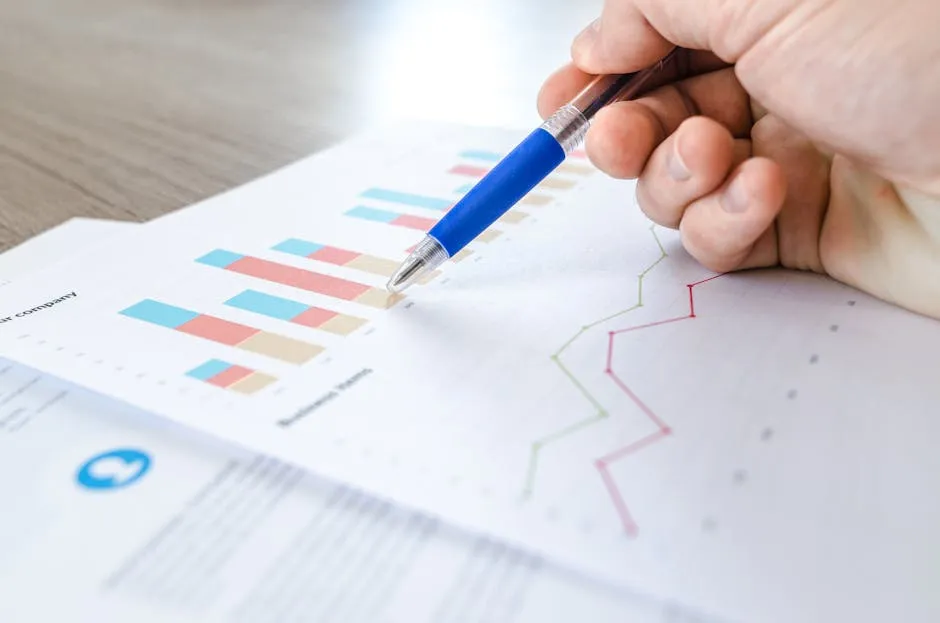
Understanding Elementary Statistics
Definition of Elementary Statistics
Elementary statistics serves as the foundation for more advanced statistical methods. It focuses on basic concepts like populations, samples, and data types. Imagine trying to bake a cake without knowing the difference between flour and sugar; that’s elementary statistics for you!
At its core, elementary statistics involves two main branches: descriptive and inferential statistics. Descriptive statistics summarizes data through measures like mean, median, and mode. On the other hand, inferential statistics helps us make predictions and generalizations about a population based on sample data.
The key difference between elementary and advanced statistics lies in their complexity. While elementary statistics focuses on foundational concepts, advanced statistics delves deeper into intricate analyses and theories. This distinction is essential for understanding when to apply basic techniques versus more complex methodologies.
Understanding these foundational concepts paves the way for more advanced exploration in fields like data science, economics, and psychology. So, whether you’re a student or a professional, grasping the essentials of elementary statistics is a crucial step in your analytical journey!

Importance of Statistics
Statistics plays a crucial role in decision-making across various fields. Think of it as the secret sauce that helps us make informed choices. Whether you’re running a business, conducting medical research, or even voting in an election, statistics is your go-to tool.
In business, statistics helps companies analyze market trends and customer preferences. For instance, a retail chain might examine sales data to determine which products are flying off the shelves. This analysis allows them to stock up on popular items and plan promotions effectively. Without statistics, they might end up with a warehouse full of items no one wants.
Healthcare is another area where statistics shines. Medical researchers use statistical methods to assess the effectiveness of new treatments. For example, during clinical trials, they compare the health outcomes of patients receiving a new drug versus those on a placebo. This helps determine if the new treatment is genuinely beneficial or just a case of wishful thinking.
Education also utilizes statistics to improve student learning. Schools analyze test scores to identify areas where students struggle. This data-driven approach allows educators to tailor their teaching methods and provide the necessary support. Imagine teachers using statistics to pinpoint exactly where students are falling behind—talk about a game plan!
For those looking to deepen their understanding of statistics, consider picking up a copy of Elementary Statistics. This textbook offers a thorough foundation that can help you grasp essential concepts and apply them to real-world scenarios.
Elections are a classic example of statistics in action. Polls use statistical sampling to predict election outcomes. By surveying a small, representative group of voters, pollsters can estimate how the entire population might vote. This can sway campaign strategies and inform voters about potential outcomes.
In summary, statistics is the backbone of informed decision-making. From business to health and education, its applications are extensive and impactful. Embracing statistical methods can lead to better outcomes, whether in a boardroom, a hospital, or a classroom. So next time you come across a statistic, remember—it’s not just numbers; it’s a powerful tool for understanding and improving our world.
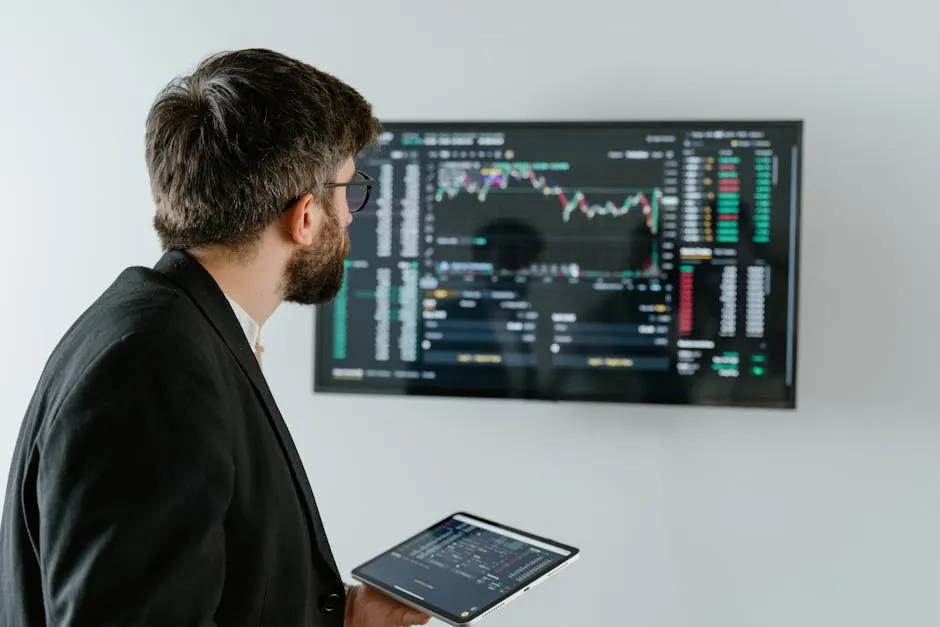
Population vs. Sample
In statistics, understanding the difference between a population and a sample is crucial. A population refers to the entire group of individuals or items that we are interested in studying. Imagine trying to count every single jellybean in a jar; that jar represents your population. On the other hand, a sample is a smaller subset of that population. Instead of counting every jellybean, you might grab a handful to get an idea of the overall mix.
Sampling methods play a significant role in statistics. They help researchers draw conclusions about a population without needing to analyze every single member. Think of it as a taste test—sampling the chocolate from a box to predict the overall quality. However, not all samples are created equal. A representative sample accurately reflects the population’s characteristics, ensuring that the insights gained are valid. If your sample is biased, like only tasting jellybeans from the top of the jar, your conclusions might be off.
When selecting a sample, various methods can be employed—random sampling, stratified sampling, and systematic sampling, to name a few. Random sampling gives everyone in the population an equal chance of being included, whereas stratified sampling divides the population into distinct groups before sampling. This ensures that specific subgroups are represented, much like ensuring all flavors of jellybeans are sampled.
To dive deeper into sampling methods and their implications, the book Statistics for Dummies is a fantastic resource. It breaks down complex concepts into digestible pieces, making it perfect for beginners.
In summary, understanding the distinction between populations and samples is foundational in statistics. It emphasizes the importance of choosing the right sampling methods to gather accurate data. A well-chosen sample can save time and resources while providing meaningful insights that reflect the larger population.

Variables
In the realm of statistics, a variable is a characteristic that can take on different values among individuals or items. Variables can be categorized into two main types: dependent and independent.
Dependent variables are those that rely on other factors. For instance, if we examine how study hours impact test scores, the test scores are the dependent variable. They depend on how much time students invest in studying. In contrast, independent variables are the factors that stand alone and can be manipulated. In our previous example, the number of study hours is the independent variable.
Let’s clarify these concepts with a few examples. Suppose a researcher is studying the effect of different fertilizers on plant growth. Here, the plant height is a dependent variable, as it changes based on the fertilizer used, the independent variable. Another example can be found in a fitness study, where the amount of exercise (independent variable) influences weight loss (dependent variable).
Understanding these types of variables is essential for conducting statistical analyses. They help researchers identify relationships between factors and predict outcomes. By clearly defining which variables are dependent and independent, statisticians can create effective models and draw meaningful conclusions from their data. For those interested in advanced modeling, The Elements of Statistical Learning is a must-read.
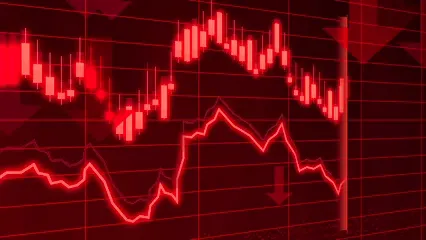
Central Tendency
Measures of Central Tendency
Central tendency is all about finding the “average” in a dataset. It helps us summarize a collection of numbers with a single value that represents the entire group. The three main measures of central tendency are mean, median, and mode.
Mean is what most people think of as the average. To find it, simply add all the numbers together and divide by the count of those numbers. For example, if you have the set {2, 4, 6, 8, 10}, the mean would be (2+4+6+8+10) / 5 = 6. Simple, right? However, the mean can be swayed by extremely high or low values, known as outliers. For further insights, you can explore what does mean identically distributed in statistics.
Understanding the concept of mean is fundamental in statistics. what does mean identically distributed in statistics provides a deeper insight into this measure.
Next up is the median, which represents the middle value when all numbers are arranged in order. If our set is {1, 3, 3, 6, 7, 8, 9}, the median is 6, as it separates the higher half from the lower half. If there’s an even number of values, average the two middle numbers. The median is particularly useful when dealing with skewed data, as it isn’t affected by outliers. For insights into median trends, you can refer to statistics poland median salary 2024.
The median is an important measure that helps in understanding data distributions. Check out statistics poland median salary 2024 for more detailed insights.
Lastly, we have the mode—the number that appears most frequently in a dataset. In the set {1, 2, 2, 3, 4}, the mode is 2 because it shows up twice. There can be more than one mode in a dataset, or none at all if all values are unique. For a deeper understanding of mode and related statistics, you may consult statsmodels residuals statistics.
The mode is a vital concept in statistics, especially in categorical data analysis. For more information, refer to statsmodels residuals statistics.
Choosing the right measure of central tendency depends on the data at hand. The mean is great for normally distributed data without outliers, while the median shines in skewed distributions. The mode is handy for categorical data where we want to know the most common category. Understanding these measures equips you with the tools to summarize and interpret data effectively!
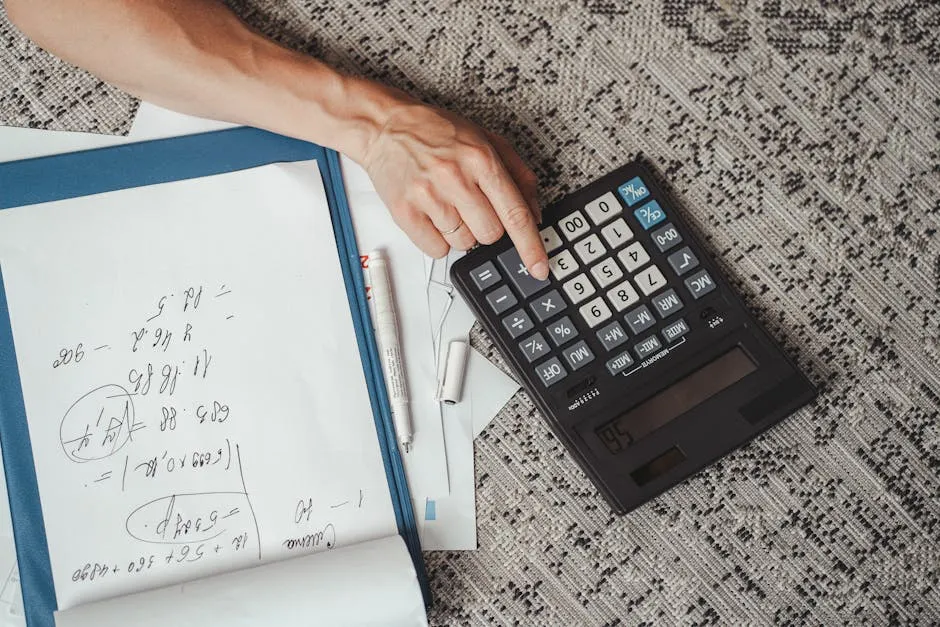
Variability
Understanding variability is crucial in statistics. It shows how much data points differ from each other. Imagine a group of friends who all love to play basketball. If one friend scores 5 points, another scores 15, and a third scores a whopping 30, you can see they have different performance levels. That’s variability in action!
Measures of Variability
Three key concepts help us measure variability: range, variance, and standard deviation. Each serves a unique purpose in understanding data spread.
Range is the simplest of the three. It represents the difference between the highest and lowest values in a dataset. For instance, if the scores of our basketball friends are 5, 15, and 30, the range is 30 – 5 = 25. A larger range indicates more variability among the scores, while a smaller range suggests they’re closer together.
Next up is variance. It takes the average of the squared differences from the mean. This means we first find the mean score, subtract it from each score to find the differences, square those differences, and finally average them. Variance gives us a sense of how far the data points spread from the mean. If our basketball scores were all around the same number, the variance would be low. Conversely, a wide spread would result in a high variance.
Last but not least is standard deviation. It’s the square root of the variance and provides insight into the average distance of each data point from the mean. A lower standard deviation means the data points are close to the mean, representing less variability. A higher standard deviation indicates data points are spread out, showing more variability.
Why do these measures matter? They help us understand the consistency of the data. In business, for example, knowing the variability in sales figures can guide inventory decisions. If sales are highly variable, a business might want to keep extra stock on hand to meet unexpected demand.
In healthcare, variability can inform treatment effectiveness. If a new medication has a low standard deviation in patient responses, it suggests consistent results—an encouraging sign for doctors and patients alike.
To gain a deeper understanding of variability, consider checking out Naked Statistics: Stripping the Dread from the Data. This book makes complex concepts accessible and enjoyable!
In summary, measures of variability—range, variance, and standard deviation—are vital tools in statistics. They help us grasp how spread out our data is, guiding decisions in various fields. Whether you’re analyzing sports statistics or evaluating healthcare outcomes, understanding variability is key to making informed choices.
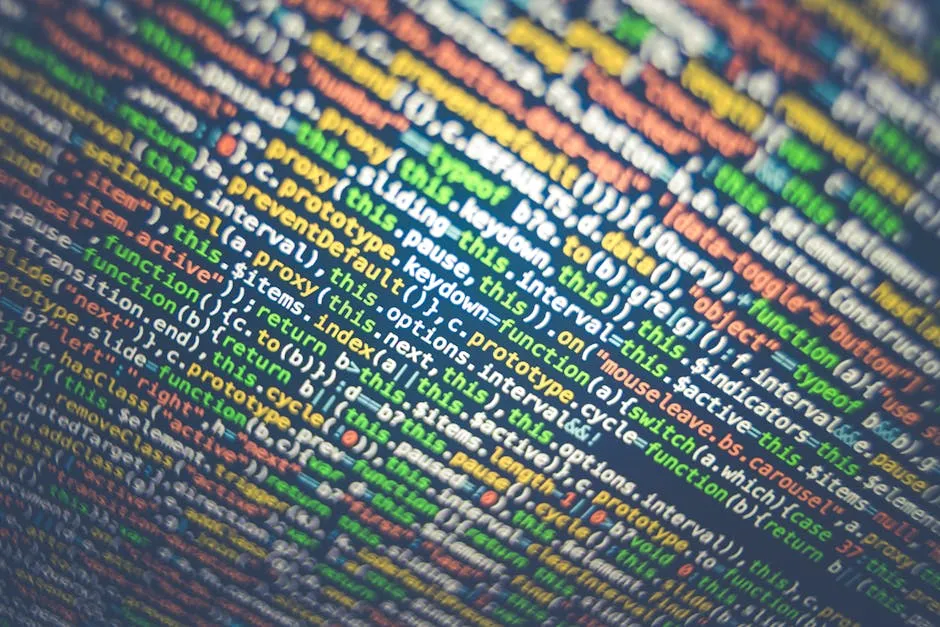
Statistical Methods
Descriptive Statistics
Descriptive statistics is all about summarizing and presenting data in a way that’s easy to understand. Think of it as the highlight reel of a game—giving you the best parts without the full play-by-play. It includes several essential methods and techniques that help us make sense of data.
The primary purpose of descriptive statistics is to provide a clear overview of the dataset. This involves calculating measures of central tendency like the mean, median, and mode. The mean is the average, the median is the middle value when data is sorted, and the mode is the most frequently occurring value. These measures help us identify general trends in the data. For example, if we want to know the average score of a class, we can easily compute that using the mean.
Visual aids play a significant role in descriptive statistics. Graphs, charts, and tables help us present data visually, making it more digestible. Bar charts can show how many students received each grade, while pie charts can illustrate the percentage of students who passed versus failed. These visuals not only engage the audience but also highlight patterns that raw numbers may obscure.
Common descriptive techniques include frequency distributions, which categorize data points to show how often each value occurs. For instance, if we have the ages of a group of people, a frequency distribution can reveal how many fall into specific age ranges. This can be particularly useful in surveys to understand demographics.
Another important method is the box plot. This graphical representation shows the median, quartiles, and potential outliers in the data. Box plots are excellent for comparing distributions across different groups. Think of it as a quick way to see if one group consistently outperforms another.
In summary, descriptive statistics serves as a powerful tool for summarizing and presenting data. By utilizing measures of central tendency and various visual aids, we can effectively communicate insights and trends. Whether in business, education, or any field that relies on data, mastering descriptive statistics is essential for informed decision-making.
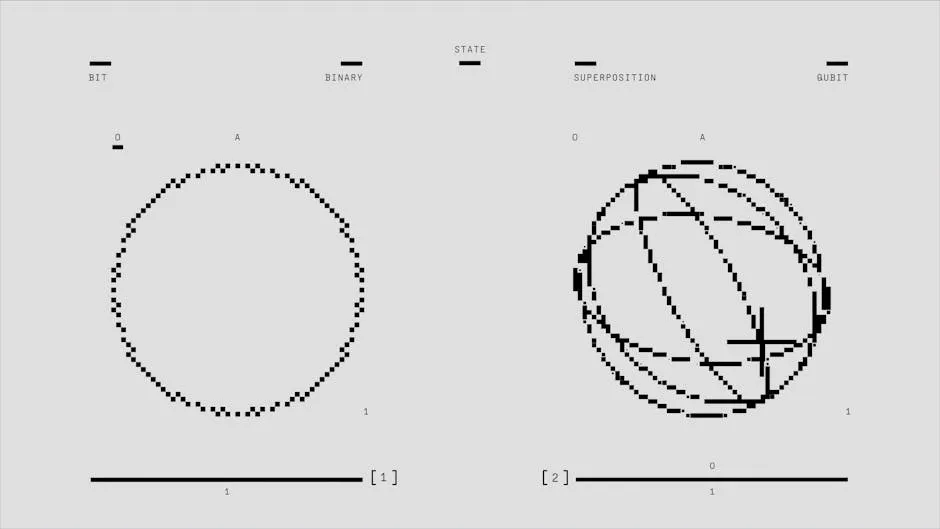
Inferential Statistics
Inferential statistics takes us a step further. While descriptive statistics summarizes data, inferential statistics helps us draw conclusions about a population based on a sample. Think of it like a detective solving a mystery—gathering clues (data) and making inferences about the bigger picture.
The primary purpose of inferential statistics is to make predictions or generalizations about a population. For example, if a pollster surveys 1,000 people about their voting preferences, they can use inferential statistics to estimate how the entire voting population might behave. This method relies on sampling—the process of selecting a subset from a larger group.
Hypothesis testing is a crucial concept within inferential statistics. It involves making claims (hypotheses) about a population and testing them against sample data. For instance, a manufacturer may claim that their light bulbs last an average of 1,000 hours. To test this, researchers can sample a batch and measure their lifespans. If the sample average is significantly different from 1,000 hours, they may reject the manufacturer’s claim.
Confidence intervals are another essential aspect of inferential statistics. They provide a range of values likely to contain the true population parameter. For instance, if a survey estimates that 60% of voters support a candidate with a 95% confidence interval of (55%, 65%), it means we can be 95% confident that the actual support lies within that range.
The beauty of inferential statistics lies in its ability to provide insights with limited data. However, it’s vital to ensure that the sample is representative of the population. A poorly chosen sample can lead to misleading conclusions.
In conclusion, inferential statistics empowers us to make informed predictions and decisions based on sample data. By understanding hypothesis testing and confidence intervals, we can analyze trends and draw conclusions that reflect the larger population. Whether in healthcare studies, market research, or social sciences, inferential statistics is an invaluable tool for understanding and interpreting data.
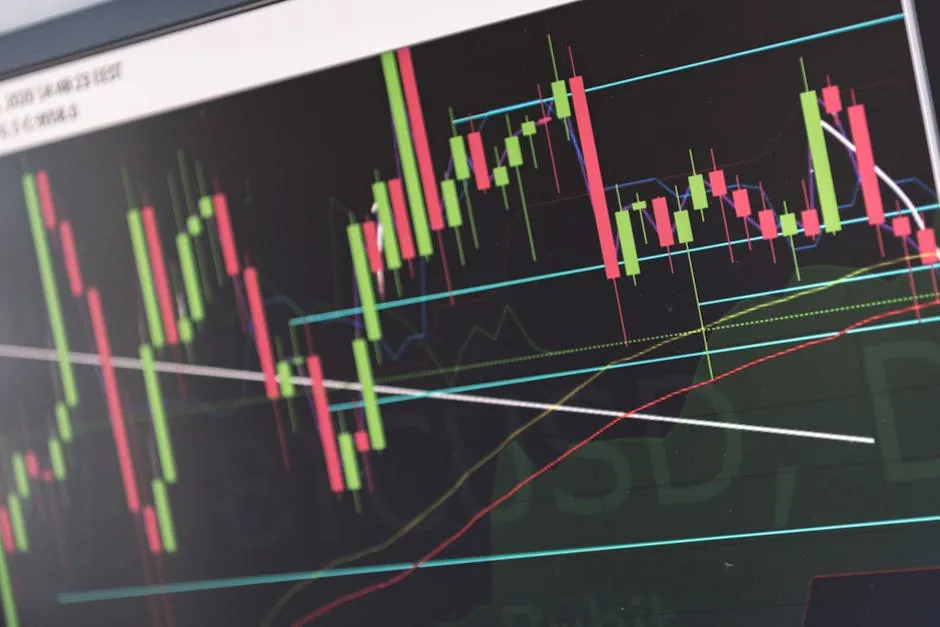
Probability
Probability is the backbone of statistics. It helps us measure uncertainty and predict outcomes. Think of probability as a crystal ball, but one that’s grounded in mathematics. In simple terms, probability tells us how likely an event is to occur.
To grasp basic probability, we start with the fundamental concept: the likelihood of an event happening. This is expressed as a number between 0 and 1. A probability of 0 means the event will never happen, while a probability of 1 means it’s a sure thing. For example, flipping a coin gives a 50% chance, or a probability of 0.5, of landing on heads.
Now, let’s sprinkle some fun into it! Imagine you’re at a party with a bowl of assorted candies. If you know there are 10 chocolates and 5 gummies, the probability of grabbing a chocolate is 10 out of 15, or roughly 0.67. So, you’re more likely to enjoy a chocolate treat than a gummy. Sweet, right?
Probability distributions take this a step further. They’re mathematical functions that provide the probabilities of occurrence of different possible outcomes. The most common distribution is the normal distribution, often depicted as a bell curve. It shows that most occurrences take place near the average, with fewer happening as you move away from it. For instance, in a classroom, if most students score around 75% on a test, but a few score 30% or 100%, we see a normal distribution at play.
But why should we care? Well, probability distributions are significant because they help us understand real-world phenomena! For example, in finance, they allow analysts to predict stock performance. In healthcare, they assist in estimating the spread of diseases. For a comprehensive guide on this topic, refer to mendelian genetics probability pedigrees and chi-square statistics.
Probability distributions are essential for understanding statistical trends. Explore mendelian genetics probability pedigrees and chi-square statistics for a deeper understanding.
Another notable probability distribution is the binomial distribution, which models the number of successes in a fixed number of independent trials, like flipping a coin multiple times. If you were to flip a coin 10 times, the binomial distribution helps you calculate the probability of getting a certain number of heads, say 6.
Understanding these concepts is essential, especially when making informed decisions. Whether you’re betting on a horse race or predicting the weather, probability gives you the tools to assess risks and rewards. For an excellent read on the nuances of probability and decision-making, check out How to Measure Anything: Finding the Value of ‘Intangibles’ in Business.
In summary, probability is not just a mathematical abstraction; it’s a practical tool that permeates our daily lives. From predicting the likelihood of events to analyzing data trends, mastering probability and its distributions equips us to navigate an uncertain world with confidence. So next time you wonder about the chances of your favorite team winning, remember: it’s all about probability!
Please let us know what you think about our content by leaving a comment down below!
Thank you for reading till here 🙂
All images from Pexels