Introduction
Statistical validity is the bedrock of sound research. It refers to the degree to which conclusions drawn from statistical analyses are accurate and trustworthy. Imagine you’re a detective, piecing together clues to solve a mystery. If your evidence isn’t valid, you might end up chasing shadows instead of the truth.
In research, statistical validity ensures that the results reflect the reality of the phenomena being studied. It’s not just about crunching numbers; it’s about interpreting them correctly. A study with solid statistical validity gives researchers confidence that their findings are reliable, paving the way for informed decisions and policies.
Understanding statistical validity is crucial for anyone engaged in research, whether in psychology, medicine, or social sciences. It helps ensure that the conclusions drawn from data analyses are not only statistically significant but also practically meaningful. For those looking to deepen their understanding of statistics, Statistics for Dummies is a great starting point!
In this article, readers will learn about the nuances of statistical validity, its significance, and the various types that exist. We’ll break it down into digestible sections to illuminate how statistical validity impacts research outcomes. Get ready to embark on a journey through the realm of statistics where clarity reigns and confusion takes a back seat!
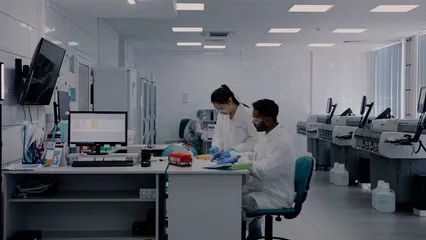
Understanding Statistical Validity
What is Statistical Validity?
Statistical validity is a multi-faceted concept that dictates the accuracy of conclusions derived from research data. To put it simply, it assesses whether the results of a statistical test are reflective of the true relationships among variables. Picture a painter creating a masterpiece. If the colors are misrepresented, the painting fails to convey the intended message. Similarly, if research data lacks statistical validity, the conclusions can be misleading.
The role of statistical validity in research is paramount. It ensures that the data collected and analyzed leads to accurate conclusions about the phenomena being studied. For instance, in psychology, researchers may use surveys to gauge mental health outcomes. If the statistical methods employed are valid, the findings can genuinely reflect the mental health status of the population. If you’re curious about how to measure anything, check out How to Measure Anything.
Let’s take a look at a few examples across different fields. In medicine, consider a clinical trial testing a new drug. Statistical validity ensures that the observed effects of the drug are not just coincidental but truly beneficial to patients. In education, a standardized test may claim to measure student intelligence. If it lacks statistical validity, it might not accurately assess what it purports to measure, leading to erroneous conclusions about student capabilities.
Understanding statistical validity is essential for researchers. It not only enhances the credibility of findings but also influences the broader applicability of research results. When studies are statistically valid, they contribute to the body of knowledge in a meaningful way, helping to shape policies, inform practices, and guide future research. In a world awash with data, ensuring statistical validity is the compass that guides researchers towards truth and accuracy.

Importance of Statistical Validity
Statistical validity is a cornerstone of credible research. Determining it is crucial because it establishes the reliability of research findings. Without it, researchers might as well toss their conclusions into a wishing well. Poor statistical validity can lead to misguided decisions. Imagine a company making a million-dollar investment based on flawed data. Ouch! The implications are significant and can mislead stakeholders, policymakers, and even the public. To help guide your understanding, consider reading Naked Statistics by Charles Wheelan for a more in-depth look at the subject.
Poor statistical validity can also mean that research findings are not reproducible. If studies can’t be replicated, they lose their power. This erodes trust in scientific findings. When results are not trustworthy, how can we expect anyone to act on them? It’s like getting advice from a fortune cookie—fun, but not reliable!
Statistical validity also contributes to research reproducibility. When studies are valid, other researchers can replicate them and arrive at similar conclusions. This creates a supportive web of knowledge, reinforcing the trustworthiness of scientific inquiry. It’s like a group of friends confirming that the pizza you ordered is, indeed, delicious. Everyone agrees, and that makes the experience even better!
In summary, understanding and ensuring statistical validity is vital. It protects researchers from erroneous conclusions and safeguards the integrity of their findings. A study with strong statistical validity becomes a beacon of reliability in the vast ocean of research, guiding others safely to shore.

Types of Statistical Validity
Overview of Validity Types
Statistical validity isn’t a one-size-fits-all concept. It encompasses several categories, each playing a unique role in the research process. These categories ensure that findings are not only accurate but also meaningful. Let’s break them down!
1. Construct Validity: This type ensures that the concepts measured align with the theoretical constructs. If a study claims to measure happiness, it should accurately reflect that emotion, not just a random collection of data.
2. Content Validity: This category assesses whether a measurement tool covers all relevant dimensions of the concept. It’s like checking if a buffet has all your favorite dishes before you dig in!
3. Face Validity: While it sounds fancy, face validity is simply about whether a test seems to measure what it’s supposed to measure. If a test claims to measure math skills, but the questions are about cooking, it clearly lacks face validity.
4. Internal Validity: This focuses on the relationship between cause and effect within the study. If researchers don’t control for extraneous variables, they risk drawing incorrect conclusions. Think of it as ensuring your experiment doesn’t have pesky distractions!
5. External Validity: External validity considers how well the findings can be generalized to broader populations. Great research findings shouldn’t just apply to a tiny sample but should resonate with larger groups.
6. Statistical Conclusion Validity: This type evaluates whether the conclusions about relationships among variables are reasonable. It’s all about ensuring that the data analysis process is robust and reliable.
Each type of statistical validity contributes to the overall strength of research findings. By understanding these categories, researchers can better design studies that yield valuable insights.
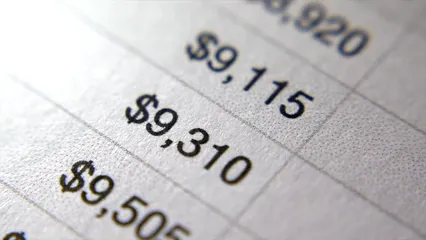
Construct Validity
Construct validity is a critical component of statistical validity. It refers to how well a test or instrument measures the theoretical concept it intends to evaluate. In simpler terms, it checks if the right thing is being measured. It’s like a chef ensuring the recipe has all the right ingredients. To grasp the importance of these ingredients, consider reading The Art of Statistics by David Spiegelhalter.
Construct validity can be further divided into two subtypes: convergent and divergent validity.
– Convergent Validity: This subtype confirms that measures designed to assess the same construct yield similar results. For example, if two different surveys aimed at measuring anxiety show similar results, they exhibit convergent validity.
– Divergent Validity: This focuses on ensuring that measures of different constructs do not correlate. If a test measuring anxiety correlates strongly with a test for happiness, something might be amiss. They should be distinct.
Construct validity is vital for establishing the credibility of research. It ensures that findings truly reflect the concepts being studied, enhancing the integrity of the entire research process. By maintaining strong construct validity, researchers can confidently assert that their conclusions are based on solid foundations. It’s like building a house on a sturdy foundation—everything else can stand tall and proud!
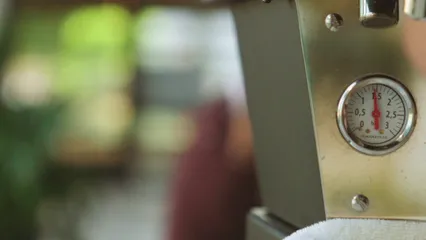
Content Validity
Content validity assesses whether a research instrument effectively covers all aspects of the concept being measured. Imagine you’re baking a cake; if you forget the flour, your dessert won’t rise! Similarly, if a test misses crucial elements, it won’t accurately measure the intended construct. Content validity is vital because it ensures that assessments are comprehensive and relevant, leading to trustworthy conclusions.
Methods for assessing content validity often include expert evaluations and systematic reviews. Researchers may enlist professionals in the field to examine the test items. They check if the content adequately represents the concept. Additionally, a thorough literature review can help identify gaps in the test’s coverage. This process ensures that all dimensions of the concept are captured, maximizing the test’s effectiveness. And for those who want to dive deeper into data science, Data Science for Dummies is a fantastic resource!
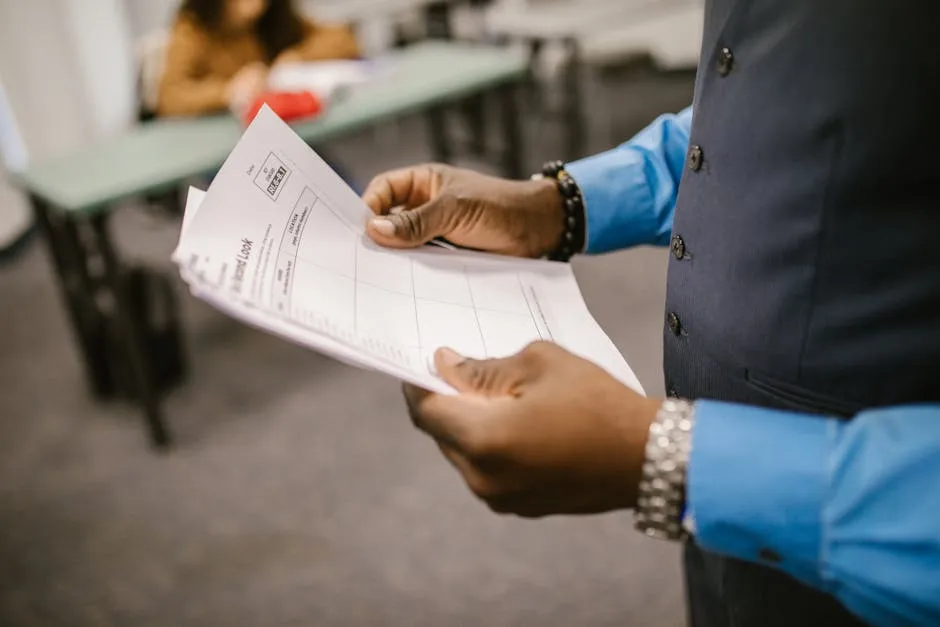
Face Validity
Face validity is a subjective measure of whether a test appears to assess what it claims to measure. Picture a math quiz filled with questions about cooking! If it looks irrelevant, it likely lacks face validity. While face validity is crucial for initial impressions, it’s not foolproof. Just because a test seems valid doesn’t guarantee it accurately measures the construct.
The limitations of relying solely on face validity are significant. It can lead to overconfidence in the test’s effectiveness, despite lacking rigorous validation. Researchers must remember that a test might look good on the surface but may not provide meaningful insights. Thus, while face validity can be a helpful starting point, it should not replace thorough assessments of validity.
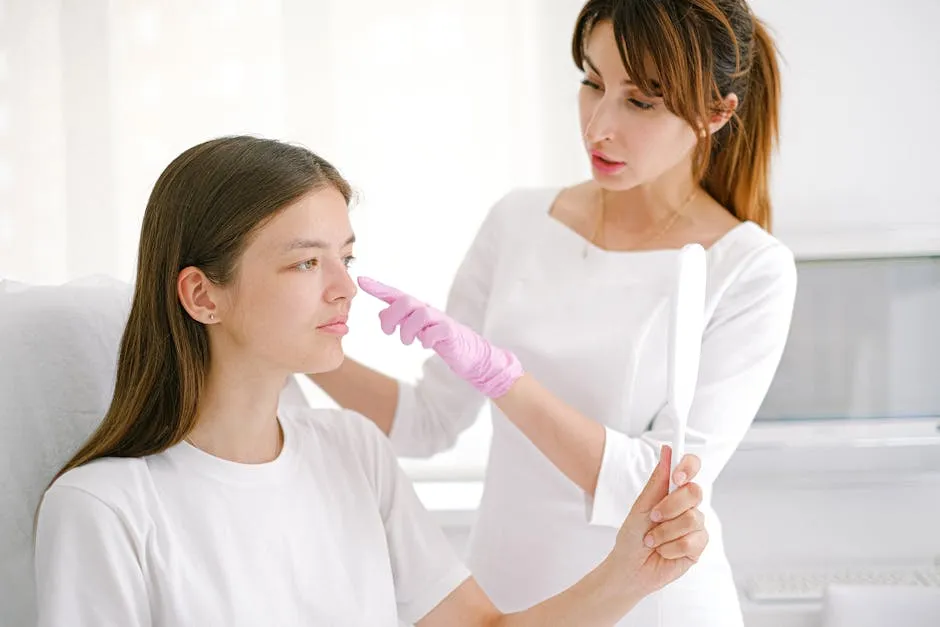
Internal Validity
Internal validity focuses on establishing causal relationships within a study. It answers the question: does the manipulation of one variable directly affect another? Think of it as ensuring the experiment is a well-controlled environment without distractions. High internal validity means researchers can confidently attribute changes in the dependent variable to the independent variable.
However, threats to internal validity abound. Confounding variables can introduce bias, making it difficult to determine cause and effect. For instance, if a study on exercise and weight loss doesn’t control for diet, the results may be skewed. Other threats include maturation, instrumentation, and selection biases. By carefully designing studies and controlling for these factors, researchers can enhance the internal validity of their findings.

External Validity
External validity is about generalizing research findings to broader populations. It answers the critical question: can the results apply outside the study’s sample? A study conducted with college students may not yield the same results in a diverse community. Ensuring external validity means researchers must consider the representativeness of their samples.
Several factors can affect external validity. Sample size, selection methods, and ecological validity all play a role. For instance, if a study is conducted in a lab setting, its findings may not translate to real-world scenarios. Researchers must carefully consider these factors when interpreting results and making broader claims. If you’re interested in a comprehensive approach to data analysis, Data Analysis Using Regression and Multilevel/Hierarchical Models by David A. Freedman is a solid choice!
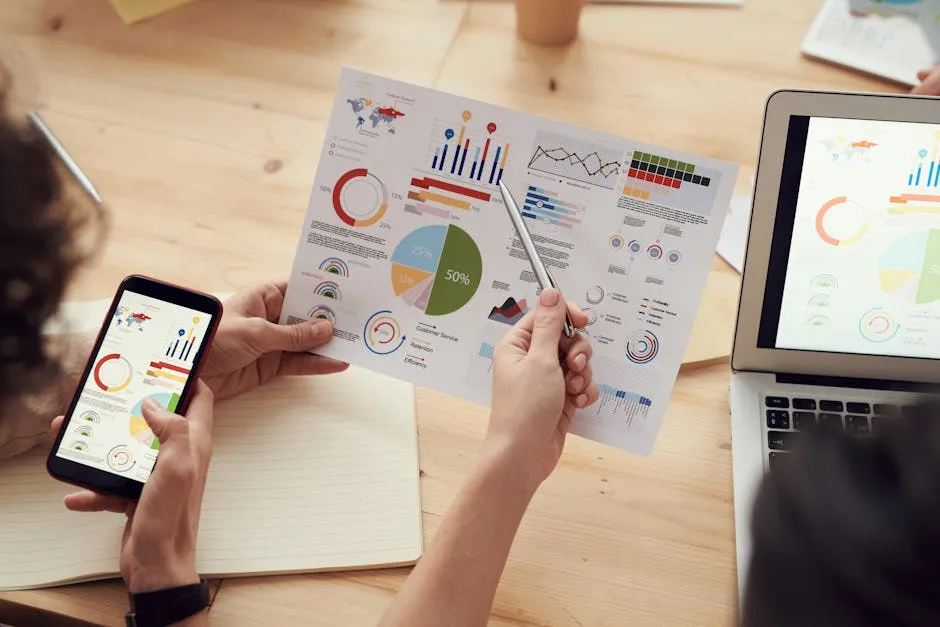
Statistical Conclusion Validity
Statistical conclusion validity refers to the degree to which conclusions drawn from data analysis are accurate. It ensures that the relationships identified among variables are reasonable and well-supported. Think of it as the backbone of effective data interpretation. Without strong statistical conclusion validity, researchers risk making misleading claims.
Common threats to this type of validity include low statistical power and violated assumptions. Low power arises from small sample sizes, increasing the likelihood of Type II errors. On the other hand, violating statistical assumptions can lead to incorrect conclusions about relationships. Researchers must ensure robust data analysis methods to mitigate these threats and enhance the validity of their statistical conclusions. For a deeper dive into statistical methods, consider Statistics: A Very Short Introduction by David Hand.
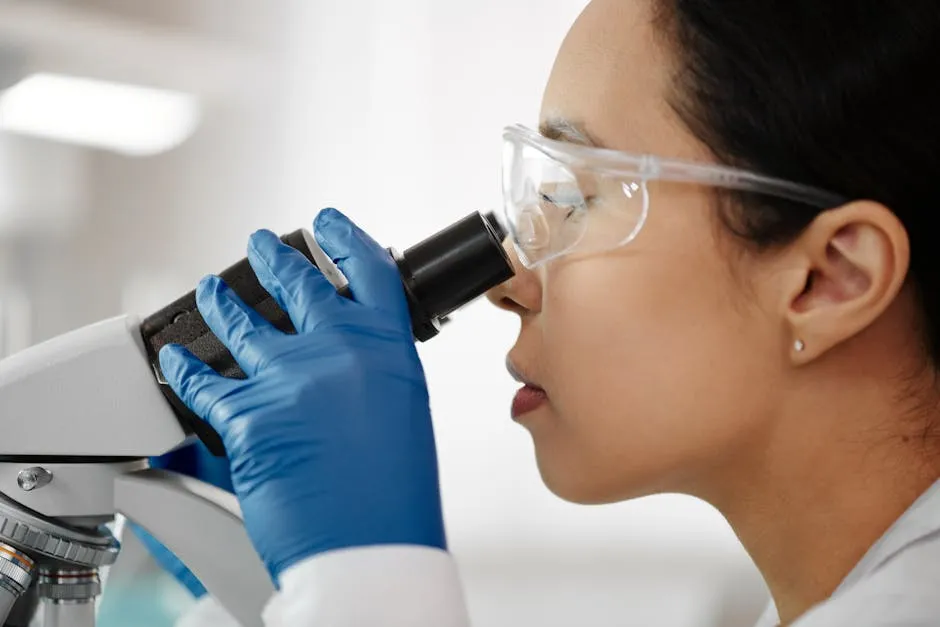
Trends and Challenges in Statistical Validity
Current Trends in Statistical Validity
Statistical validity has seen exciting advancements lately. Researchers are embracing new statistical methods that boost the reliability of their findings. With the explosion of big data, traditional approaches have become the old guard. Enter techniques like Bayesian statistics and machine learning, which offer fresh perspectives on validity. These methods allow for more nuanced analyses, accommodating complex data structures that were once too cumbersome for classic methods.
The integration of Bayesian statistics is revolutionizing how researchers approach validity in their studies.
Technology plays a starring role in this evolution. Sophisticated software packages now automate rigorous statistical analyses. Imagine a researcher, coffee in hand, clicking a few buttons to run advanced models. This not only saves time but also minimizes human error. As a result, researchers can focus on interpreting results instead of getting lost in the nitty-gritty of calculations.
Robust statistical practices are now more important than ever. Researchers are increasingly aware that valid conclusions stem from solid methodologies. This means larger sample sizes, better measurement tools, and comprehensive designs. The emphasis on reproducibility has led to a renaissance in meticulous study planning. Making findings reproducible is the gold standard, ensuring that results can stand the test of time, similar to a classic movie that never gets old.
Moreover, interdisciplinary collaboration is on the rise. Statisticians are teaming up with domain experts to craft studies that are both valid and relevant. Think of it as a culinary team where chefs and nutritionists co-create a dish that’s not only tasty but also healthy. These collaborative efforts yield richer insights and more dependable outcomes. If you want to delve into the art of data science, The Art of Data Science by Roger D. Peng and Elizabeth Matsui is a must-read!
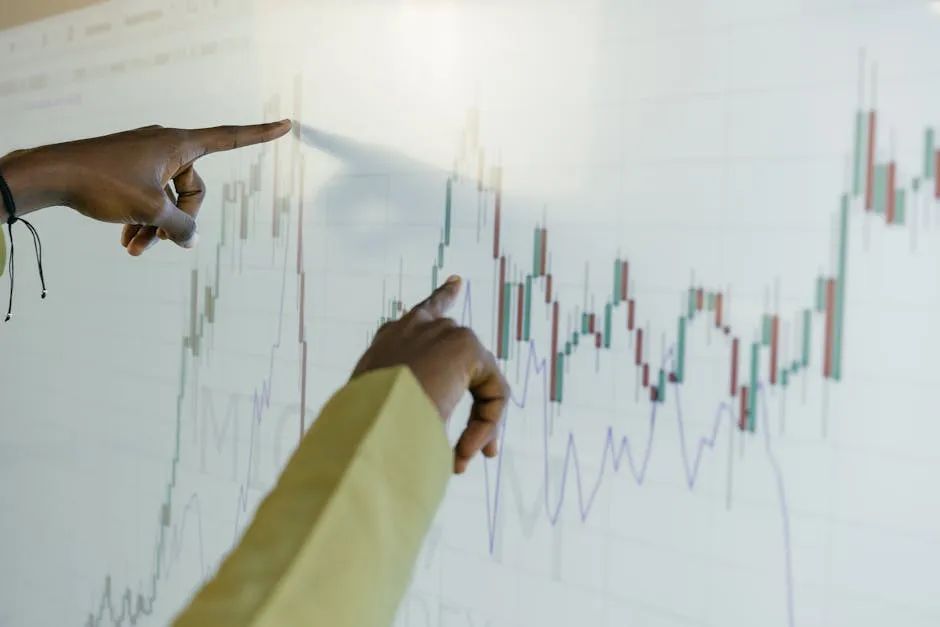
Challenges in Achieving Statistical Validity
While the advancements are promising, challenges remain. One of the most significant hurdles is ensuring adequate sample sizes. Researchers often struggle to gather enough data, especially in niche fields. A small sample size can lead to unreliable conclusions, much like trying to predict the weather based on one day’s observation. When results are based on scant data, they may not reflect broader trends.
Measurement errors are another common pitfall. Researchers must ensure that the tools they use accurately capture the constructs they aim to study. If a survey designed to assess happiness instead measures stress levels, conclusions could go awry. This is like trying to catch a fish with a net full of holes—ineffective and frustrating!
Data analysis techniques also pose challenges. Many researchers still rely on outdated methods or fail to check statistical assumptions. Ignoring these assumptions can skew results. It’s akin to baking a cake without measuring ingredients. You might end up with something edible, but not the scrumptious treat you envisioned. To improve your analysis skills, consider Python for Data Analysis by Wes McKinney.
So, how can researchers overcome these challenges? First, they should prioritize well-powered studies. Increasing sample sizes can bolster statistical validity and provide more reliable insights. Additionally, investing in high-quality measurement tools is crucial. The right instruments can reduce measurement errors and enhance the accuracy of findings.
Furthermore, researchers should continually educate themselves about current statistical techniques. Staying informed about the latest developments empowers them to choose the best methods for their studies. Collaboration with statisticians can also provide valuable insights into the design and analysis phases, ensuring that the research stands on solid ground.
In summary, while the landscape of statistical validity is evolving, it’s essential to navigate the challenges with care. By embracing robust practices and prioritizing education, researchers can ensure that their findings are not just valid but truly meaningful. After all, the pursuit of knowledge should be as enjoyable as a good book—full of twists, insights, and a satisfying conclusion. For those looking for insightful reads, Thinking, Fast and Slow by Daniel Kahneman is highly recommended!

Conclusion
Understanding statistical validity is not just an academic exercise; it’s a crucial pillar of credible research. Throughout this article, we’ve unpacked the concept of statistical validity, emphasizing its role in ensuring that conclusions drawn from research are accurate and meaningful. Think of statistical validity as the GPS of research—without it, you might find yourself wandering aimlessly, lost in a sea of numbers and misinterpretations.
Statistical validity is essential for building confidence in research findings. It protects researchers from the pitfalls of faulty conclusions that could mislead stakeholders and the public. A study devoid of statistical validity is like a house built on sand—no matter how beautiful it looks, it’s bound to collapse when the tides of scrutiny come in. By ensuring that studies are statistically valid, researchers contribute to a body of knowledge that is reliable and useful.
Moreover, the implications of statistical validity extend beyond academia. In fields like medicine, education, and public policy, the consequences of poor statistical validity can be significant. Decisions based on flawed research can lead to ineffective treatments, misguided educational policies, and unwise regulatory measures. Therefore, understanding and applying statistical validity should be a priority for all researchers.
In conclusion, as you venture into your own research endeavors, remember the importance of statistical validity. Take the time to rigorously assess and ensure that your findings are grounded in robust statistical practices. The integrity of your research—and its impact on the world—depends on it. So, go forth and let statistical validity guide you, ensuring your research stands the test of time!

FAQs
What is the difference between reliability and validity?
Reliability and validity are both crucial concepts in research, but they refer to different aspects. Reliability is all about consistency. It measures how dependable a particular measurement is over time or across different conditions. If you take a test multiple times and get similar results, that test is reliable. Validity, on the other hand, is about accuracy. It assesses whether a test measures what it claims to measure. A valid test will provide true reflections of the concept being investigated. For example, a scale may consistently show the same weight (reliable) but could be miscalibrated, leading to inaccurate readings (not valid). In short, a measurement can be reliable without being valid, but it cannot be valid unless it is reliable.
How can researchers ensure their study has high statistical validity?
Researchers can enhance statistical validity by following a few best practices. First, they should ensure an adequate sample size. A larger sample size generally leads to more reliable results and reduces the risk of Type I and Type II errors. Secondly, selecting the appropriate statistical tests based on the research design and data characteristics is crucial. Using the right tools ensures that the data analysis accurately reflects the relationships among variables. Additionally, researchers should control for confounding variables. This means keeping external factors that could influence the results in check. Lastly, conducting pilot tests can help identify potential issues in the methodology before the main study. These practices contribute significantly to achieving high statistical validity.
What are common mistakes that affect statistical validity?
Several frequent errors can jeopardize statistical validity. One common mistake is using a sample size that is too small, which increases the likelihood of drawing incorrect conclusions. Another pitfall is failing to meet the assumptions required for specific statistical tests; violating these assumptions can lead to skewed results. Additionally, researchers sometimes overlook confounding variables, which can obscure true relationships between data points. Lastly, relying too heavily on p-values without considering effect sizes or confidence intervals can lead to misleading interpretations. Awareness of these pitfalls can help researchers navigate the intricate landscape of statistical validity.
Why is statistical validity important in policy-making?
Statistical validity plays a vital role in policy-making. Decisions based on research that lacks statistical validity can lead to ineffective or harmful policies. For instance, if a study claims that a specific intervention improves public health but lacks validity, policymakers might allocate resources ineffectively, ultimately harming the population they intend to help. Moreover, valid research fosters public trust. When studies are conducted with robust statistical practices, they produce reliable findings that can be confidently shared with stakeholders. Policymakers rely on these findings to make informed decisions that affect communities, economies, and public welfare. Thus, ensuring statistical validity is essential for responsible governance and effective policy development.
Can a study be valid but not reliable?
Yes, a study can be valid but not reliable. For instance, imagine a test that measures creativity with a one-time assessment. If the test accurately captures the essence of creativity at that moment, it is valid. However, if the same test yields drastically different results when taken again later, it is not reliable. In this case, while the test may measure creativity accurately, it fails to provide consistent results over time. Validity focuses on whether a test measures what it’s supposed to measure, while reliability assesses the consistency of those measurements. Therefore, a measure can be valid in a specific context but not reliable across different situations or times.
Please let us know what you think about our content by leaving a comment down below!
Thank you for reading till here 🙂
All images from Pexels