Introduction
Let’s face it: statistics can be a bit of a maze. But don’t worry, we’re here to simplify it! So, what’s the deal with statistics and parameters? In the world of data analysis, these two terms often collide, creating confusion. Here’s the scoop: a statistic is derived from a sample, while a parameter describes an entire population.
Understanding these concepts is crucial! Why? Because they help researchers and analysts make sense of vast amounts of data. Properly distinguishing between them can lead to better conclusions and decisions. Imagine trying to guess how many jellybeans are in a jar by counting just a handful—sounds tricky, right? That’s where knowing the difference becomes essential.
This article aims to clarify the differences between a statistic and a parameter. We’ll walk through definitions, roles, and examples, all while keeping it light and engaging. Buckle up; let’s dive into the colorful world of data!
To kickstart your journey into the world of statistics, consider picking up a copy of Statistics for Dummies. This book breaks down complex concepts into bite-sized pieces, making statistics approachable for everyone—yes, even for your Aunt Edna who thinks Excel is a type of pasta!
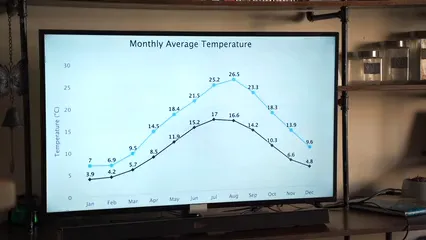
Understanding Statistics and Parameters
What is a Parameter?
A parameter is a numerical characteristic that describes the entire population. Think of it as the “big picture” measure. For instance, consider the average income of all households in a country. This average, denoted as μ (mu), represents the true income level across the entire population. It’s fixed and remains constant—unless, of course, the population changes drastically.
Parameters play a vital role in statistical studies. They help in formulating hypotheses and guiding research design. However, obtaining accurate parameters can be a challenge since it often requires surveying everyone in the population, which is rarely feasible. That’s where sampling comes into play, but we’ll get to that soon!
Parameters are like the gold standard of data measurement. They give a complete view of the population. However, since they are often unknown, researchers typically estimate them using sample statistics. So, while parameters provide clarity, they can also be elusive!
To put it simply: parameters are the fixed values that define population characteristics, like a lighthouse guiding you through the foggy seas of data. They’re essential, but good luck getting your hands on the exact numbers without some clever estimation!
If you’re interested in learning more about how to analyze data effectively, check out The Data Warehouse Toolkit: The Definitive Guide to Dimensional Modeling. This book provides essential strategies for data organization and analysis, perfect for anyone looking to elevate their data game!
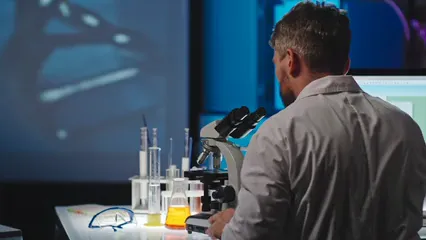
What is a Statistic?
A statistic is a numerical characteristic derived from a sample. It provides insights about a smaller group rather than the entire population. Think of it as a quick snapshot of a broader picture. For example, if you gather data from 100 households and find that their average income is $60,000, that figure is a statistic. We denote it as x̄ (read as “x-bar”). It’s like peeking at a few jellybeans in a jar to guess how many are inside!
Statistics are crucial in data analysis. They help researchers estimate parameters, which are often unknown. By analyzing sample data, statistics allow us to make informed decisions without needing the entire population’s data. So, while a statistic might not capture the whole essence, it sure helps us understand the gist!
Key Differences Between Statistics and Parameters
Now that we know what a statistic is, let’s break down the main differences between statistics and parameters:
- Scope: Parameters describe entire populations, while statistics pertain to samples.
- Value: Parameters are fixed values. In contrast, statistics can vary depending on the sample chosen.
- Estimation: Parameters are often unknown, making statistics essential for estimation.
To sum it up, if you’re working with a specific sample, you’re dealing with statistics. If you’re aiming for the whole crowd, you’re talking about parameters. Understanding these differences helps avoid confusion, especially when interpreting data!
For those diving deeper into the realm of data science, The Art of Statistics: Learning from Data is a fantastic resource. It explores how to extract meaningful insights from data, making it a must-read for aspiring analysts!
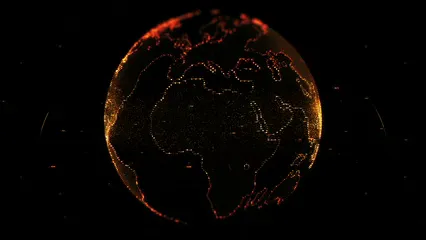
Symbolic Representation
Notation for Parameters
Parameters are represented using specific symbols. Here are some common ones:
- Population mean (μ): This symbol denotes the average of an entire population. Think of it as the ultimate answer based on complete data.
- Population standard deviation (σ): This represents the dispersion or variability of the population data. It’s like measuring how spread out the jellybeans are in the jar!
- Population proportion (P): This is used to denote the fraction of the population with a certain characteristic.
These symbols help statisticians communicate findings clearly. When you see μ or σ, you know they’re talking about the whole shebang, not just a slice of it!
Notation for Statistics
When we talk about statistics, we often use symbols to represent various measures. Here are some common symbols you need to know:
- Sample Mean (x̄): This symbol represents the average value calculated from a sample. It’s like taking a small bite of a cake to guess the whole flavor!
- Sample Standard Deviation (s): This denotes the variability of data points in a sample. Think of it as the measure of how much the jellybeans in your jar differ in size.
- Sample Proportion (p̂): This symbol represents the fraction of the sample that possesses a certain characteristic. It’s like saying, “Out of the 100 jellybeans, 30 are red!”
These symbols help convey statistical data quickly and effectively, making it easier for researchers to communicate their findings.
Comparison Table
Here’s a quick reference table comparing the symbols and descriptions of parameters and statistics:
Measurement | Parameter Notation | Statistic Notation |
---|---|---|
Mean | μ (mu) | x̄ (x-bar) |
Standard Deviation | σ (sigma) | s |
Proportion | P | p̂ (p-hat) |
Variance | σ² (sigma squared) | s² |
Sample Size | N | n |
This table highlights the key differences in notation, helping you understand whether you’re dealing with a parameter or a statistic at a glance!
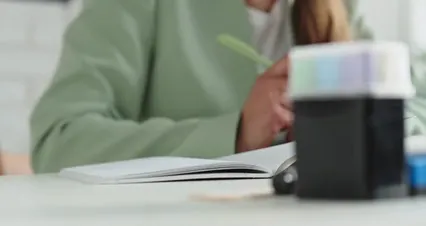
Examples of Parameters and Statistics
Real-World Examples of Parameters
Parameters are the gems of population data. Here are some key examples:
- Proportion of a Characteristic: Imagine a population of all U.S. adults. If we say that 60% of them have a college degree, that percentage is a parameter, represented as P. It reflects the entire population without any guesswork.
- Mean Height of Adult Males: Suppose the average height of all adult males in the U.S. is 5 feet 9 inches, denoted as μ. This parameter gives a complete picture of the population’s height.
Parameters are fixed values and provide a solid foundation for understanding entire populations.
Real-World Examples of Statistics
Statistics come into play when we can’t measure everyone. Here are some examples:
- Survey Results: Let’s say a survey indicates that 55% of respondents support a new policy. This percentage, derived from a sample, is a statistic, represented as p̂. It gives us a glimpse of public opinion without knocking on every door!
- Average Test Score: If a teacher calculates that the average score of 30 students in a class is 78 out of 100, that’s a statistic, denoted as x̄. It helps gauge the performance of that specific group, but it doesn’t reflect the scores of all students in the school.
Statistics are valuable tools for making informed estimates about larger populations based on sample data. They help navigate the vast seas of information when complete data isn’t accessible.
In summary, parameters describe whole populations and remain fixed, while statistics provide insights from samples and can vary. Understanding these distinctions is key for anyone in the world of data analysis!

Differences Between Population Parameters and Sample Statistics
Parameters and statistics are like two sides of the same coin. Parameters provide precise characteristics of entire populations, while statistics offer insights derived from samples. Let’s break this down.
Parameters are the gold standard. They describe the entire population, representing values that are fixed and constant. For instance, consider the average income of all households in a country. This average, denoted as μ (mu), gives a clear picture of the population’s economic status. However, here’s the kicker: parameters often remain elusive. Gathering data from every individual in a population is like trying to catch smoke with your bare hands. Almost impossible!
On the flip side, we have statistics. Statistics come into play when we can’t survey everyone. A statistic is calculated from a sample—think of it as a snapshot rather than a full movie. For example, if you survey 1,000 households and find the average income is $60,000, that’s a statistic, denoted as x̄ (x-bar). It gives us a glimpse of the larger whole but can vary widely depending on the sample chosen. Different samples might yield different averages, leading to variations that affect the reliability of our conclusions.
Here’s where the fun begins: interpreting statistics requires a bit of detective work. You have to consider sampling methods and potential biases. If you use a biased sample, your statistic might lead you astray. Imagine trying to guess the average height of a population by only measuring basketball players. Spoiler alert: you’ll end up with a skewed view!
So, in summary, parameters provide a crystal-clear description of populations, while statistics offer a variable, sample-based insight. Understanding this distinction is crucial for making informed decisions and avoiding the pitfalls of misinterpretation. As you venture further into the world of data, keep these differences in mind; they will guide you through the statistical maze with confidence!
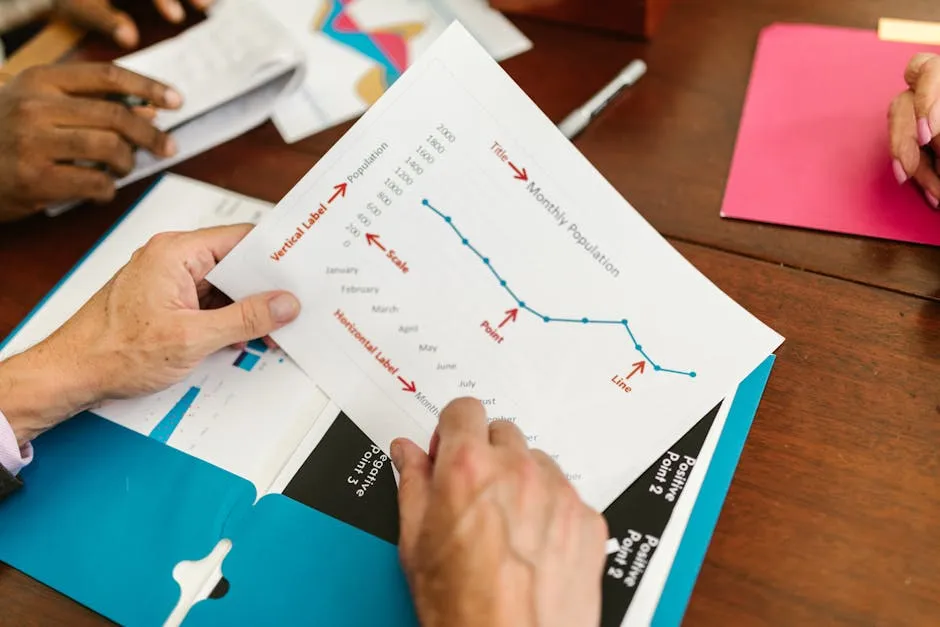
Common Misconceptions
Ah, the classic case of mixing up statistics and parameters! Many folks assume these terms are interchangeable. Spoiler alert: they’re not! Think of it this way: a statistic is like that one friend who only shares their best stories—derived from a sample, it gives a glimpse but not the full picture. A parameter, on the other hand, is more like a family photo—captures the whole gang, all in one frame.
Understanding these distinctions is crucial in data analysis. Why? Because using statistics when you should be referencing parameters can lead to misconceptions. For instance, if you claim a statistic represents a population parameter, you might be misleading your audience. And nobody likes a party pooper!
Recognizing that statistics stem from samples while parameters describe entire populations can help clarify your analysis. Keeping these definitions straight ensures you communicate effectively and accurately. So, the next time you hear someone toss around these terms, remember: they’re not just numbers; they’re distinct concepts that play different roles in the statistical world!
Conclusion
In the grand scheme of data analysis, distinguishing between statistics and parameters is paramount. Each serves a unique purpose, and understanding their differences can elevate your analytical game.
To recap, a parameter is a fixed value that describes a whole population, while a statistic is a variable measure derived from a sample. Parameters provide the complete picture, but they often remain elusive. We estimate them using statistics, which can change from sample to sample. This dynamic interplay is what makes data analysis both intriguing and challenging.
Emphasizing these distinctions is crucial for anyone involved in research or analysis. Misinterpreting or misapplying these concepts can lead to flawed conclusions. So, whether you’re a seasoned analyst or a curious newbie, always remember to ask yourself: am I referencing a statistic or a parameter? Your conclusions depend on it!
As you engage with data in your own practices, lean on this understanding. It can guide your decisions, improve your interpretations, and ultimately enhance your insights. Statistics and parameters are not just academic terms; they’re the building blocks of informed decision-making in our data-driven world. So go forth and analyze wisely!
For those looking to harness the power of data science, I recommend Data Science for Business: What You Need to Know about Data Mining and Data-Analytic Thinking. It’s a fantastic resource that bridges the gap between data science and practical business applications!
FAQs
What is the difference between a statistic and a parameter?
A statistic refers to a numerical value derived from a sample, while a parameter is a numerical value that describes an entire population. In other words, statistics are estimates based on sample data, while parameters give you the true characteristics of the entire group.
Are statistics and parameters used for different purposes?
Absolutely! Statistics help estimate population parameters based on sample data. They provide insights about a specific group, while parameters represent fixed values that describe the entire population.
Can statistics accurately estimate parameters?
Statistics can estimate parameters, but their accuracy depends on several factors. Sample size, data quality, and the sampling method all play significant roles. Larger and well-chosen samples generally yield more accurate estimates.
Why are statistics vital in data analysis?
Statistics are essential because they allow researchers to draw conclusions and make informed decisions without needing data from an entire population. They simplify complex data into understandable metrics, aiding in effective communication and analysis.
How do I know if I should use a statistic or a parameter?
When working with a sample, use statistics. If you’re looking at the entire population and have complete data, parameters are your go-to. Assess your data context to determine which to apply in your analysis.
Please let us know what you think about our content by leaving a comment down below!
Thank you for reading till here 🙂
For a deeper understanding of the differences between inferential and descriptive statistics, check out this article on inferential statistics vs descriptive statistics in data interpretation.
If you’re interested in understanding what “identically distributed” means in statistics, you can read more about it here.
For tips on effective data analysis, especially in economics and statistics, visit this guide.
For a comprehensive overview on statistical learning with Python, including book length and content, check out this introduction.
And for those looking to incorporate statistical analysis into their work, I highly recommend Statistical Methods for the Social Sciences. This book provides a robust foundation for applying statistical methods in social research, making it a valuable addition to your library!
Lastly, if you need to keep your data organized, a reliable USB Flash Drive can be a lifesaver. Store your datasets and analysis in one place, ensuring you always have your work at your fingertips!
All images from Pexels