Introduction
Statistics is the art of turning data into decisions. Whether you’re deciphering sales reports or analyzing scientific research, statistics play a crucial role. It’s like the Swiss Army knife of data—practical, versatile, and occasionally confusing.
Among the many symbols used in statistics, the Greek letter “mu” (μ) holds a special place. It represents the population mean, a fundamental concept that helps us understand the average of a dataset. Essentially, μ is the go-to symbol for the average value of a population, and understanding it is crucial for anyone looking to make sense of data.
In this article, we’ll unravel the mysteries surrounding μ. You’ll learn what it is, how it’s calculated, and why it matters. We’ll also dive into its significance in statistical analyses and explore real-world applications. By the end, you’ll be equipped with the knowledge to wield mu like a pro, ready to tackle your next data challenge.
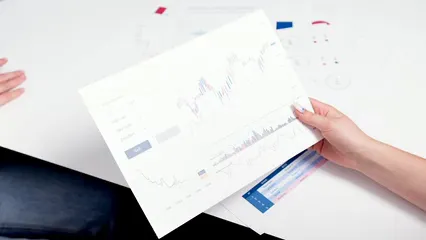
Understanding the Population Mean
What is Mu in Statistics?
Let’s get to the heart of the matter. The symbol μ (pronounced “mew”) represents the population mean, which is essentially the average of a given population. It’s a key player in any statistician’s toolkit. Why? Because it gives us a snapshot of what to expect from a dataset.
The formula for calculating μ is straightforward: \[ μ = \frac{ΣX_i}{N} \] Here, \( ΣX_i \) is the sum of all data points, while N is the total number of observations. This formula sums up all the values in the population and divides by the number of values. Voila! You have the population mean.
To illustrate, consider a classroom of five students with scores of 80, 90, 100, 70, and 60. To find μ, you’ll add those scores: 80 + 90 + 100 + 70 + 60 = 400. Then divide by the number of students (5). So, μ = 400 / 5 = 80. Simple, right?
The beauty of μ lies in its ability to summarize data. It provides a reference point, allowing researchers to gauge how individual observations stack up against the average. However, let’s not forget that while μ is a powerful tool, it can be sensitive to outliers. A single extreme value can skew the mean, leading to a distorted picture of the population.
In summary, understanding μ is essential for grasping statistical concepts. It serves as a foundation for exploring variance, standard deviation, and other critical measures. As we proceed, we’ll see how μ interacts with these elements, enhancing our overall understanding of data analysis. So, grab your calculators and get ready to crunch some numbers!
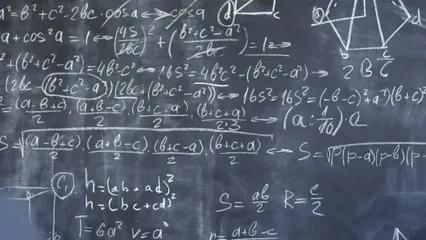
Calculation of the Population Mean
Calculating the population mean (μ) is like baking a cake: follow the recipe, and you’ll have a delicious result! Let’s break it down step by step using an example dataset.
Imagine you have a group of five friends, and you want to know their average age. Here are their ages: 25, 30, 35, 40, and 45.
1. Step 1: Add Up All Values First, we need to sum all the ages. \( 25 + 30 + 35 + 40 + 45 = 175 \).
2. Step 2: Count the Total Number of Observations Count how many friends you have. In this case, it’s 5.
3. Step 3: Divide the Total by the Number of Observations Now, take the sum from Step 1 and divide it by the number of friends from Step 2. \( μ = \frac{175}{5} = 35 \).
So, the population mean age of your friends is 35 years!
Calculating the population mean is crucial in various scenarios. For instance, in surveys, researchers calculate the population mean to summarize data efficiently. In experiments, it helps determine the average effect of a treatment, guiding decisions for future studies. If you’re serious about mastering these concepts, check out Statistics for Dummies for a comprehensive guide to statistical concepts.
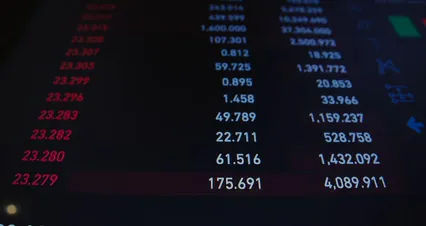
Differences Between Population Mean and Sample Mean
Now, let’s discuss how the population mean (μ) differs from the sample mean (x̄). Think of the population mean as the complete picture, while the sample mean is like a snapshot.
The population mean (μ) represents the average of all individuals in a specific population. In contrast, the sample mean (x̄) is the average derived from a smaller subset of that population.
For example, let’s say you want to know the average height of all adults in your city. If you measure every adult, that’s μ. But if you only measure 100 adults, that average is x̄.
Using sample means for estimation comes with implications. The sample mean is subject to sampling variability, which means it can differ based on the individuals selected. If your sample isn’t representative, your estimate might miss the mark. Thus, while x̄ provides valuable insights, it might not always reflect the true population mean (μ).
Understanding these differences is vital for accurate statistical analysis and decision-making. If you want to delve deeper into sampling methods, consider picking up The Data Science Handbook.

Applications of the Population Mean
The population mean, denoted by μ, plays a pivotal role across various fields. From social sciences to quality control, its applications are vast and impactful. Let’s take a closer look at how μ makes a difference in the real world.
In the realm of social sciences, μ is often used to analyze survey data. For instance, consider a study on average income levels in a city. Researchers collect income data from all residents, calculate μ, and gain insights into the economic status of the population. By understanding the average income, policymakers can design targeted programs to address economic disparities. For more on this topic, check out The Art of Statistics: Learning from Data.
Understanding the average income in social sciences can greatly influence policy-making. Agresti Statistical Methods for the Social Sciences provides deeper insights into this area.
Similarly, in education, μ is crucial for interpreting test scores. Schools often calculate the average score (μ) of students in standardized tests to evaluate performance. If the average score improves over the years, it may indicate effective teaching strategies. Conversely, a decline might prompt a review of curricula and teaching methods. To enhance your understanding of educational assessments, consider reading Practical Statistics for Data Scientists.
In economics, μ assists in evaluating macroeconomic indicators. For example, economists calculate the average GDP growth rate of a country over several years using μ. This information is essential for understanding economic trends and making informed policy decisions. A steady increase in average GDP could signal economic health, while stagnation might raise red flags.
Quality control professionals use μ to monitor product performance. By calculating the average output of a manufacturing process, they can ensure that products meet quality standards. If μ falls below a certain threshold, it could indicate a problem in the production line, prompting immediate action to rectify issues. For those in manufacturing, consider exploring Statistical Quality Control: A Modern Introduction.
In healthcare, μ can be used to analyze patient outcomes. Researchers may study the average recovery time (μ) from a specific surgery. If the average recovery time decreases over time, it could suggest improvements in surgical techniques or post-operative care.
These examples illustrate the importance of the population mean. Whether it’s guiding policy decisions, evaluating educational effectiveness, or ensuring product quality, μ is a vital statistical tool that sheds light on average values across various sectors.

Relationship with Other Statistical Measures
Connection to Standard Deviation and Variance
When discussing μ, it’s essential to understand its relationship with standard deviation (σ) and variance (σ²). Standard deviation measures the dispersion of data points relative to the population mean. It provides insight into how much individual observations differ from μ.
Variance, on the other hand, is the square of the standard deviation. It quantifies the average squared deviation of each data point from the population mean. Both of these measures are crucial for interpreting data distribution.
To clarify further, imagine a dataset representing the heights of a group of individuals. If μ is 170 cm, a low standard deviation indicates that most heights are close to this average. Conversely, a high standard deviation suggests a wide range of heights, with some individuals significantly shorter or taller than the average. If you want to dive deeper into statistical theory, consider reading The Elements of Statistical Learning.
Understanding σ and σ² helps in visualizing data spread. In a normal distribution, about 68% of data points fall within one standard deviation of μ. About 95% fall within two standard deviations, and nearly all (99.7%) fall within three. This relationship forms the basis of the empirical rule, which is fundamental in statistics.
The significance of these measures extends to hypothesis testing and confidence intervals. When making inferences about a population based on sample data, knowing the spread of data around μ is crucial. It allows statisticians to assess the reliability of their estimates and conclusions.
In summary, the population mean (μ) is intricately linked to standard deviation and variance. Together, they provide a comprehensive picture of data distribution, enhancing our understanding of statistical analysis. By grasping these relationships, researchers can make informed decisions and interpretations based on their findings.
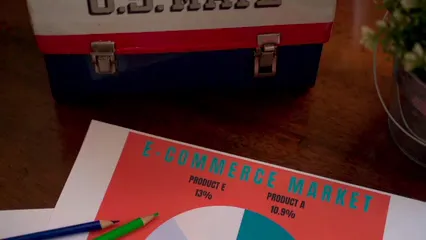
Central Limit Theorem and Its Implications
The Central Limit Theorem (CLT) is a cornerstone of statistics. It states that as sample sizes increase, the distribution of sample means approaches a normal distribution, regardless of the population’s shape. This is significant because it allows statisticians to make inferences about a population based on sample data.
Imagine you’re at a party. You decide to ask everyone their age but only manage to ask a few. The ages you collect might look scattered and chaotic—much like a toddler’s art project. However, if you keep asking more people (increasing your sample size), the average of those ages will begin to cluster around the actual population mean (μ). This clustering effect is the beauty of the CLT!
As sample sizes grow, the sample mean (x̄) becomes a reliable estimator of the population mean (μ). The larger the sample, the closer x̄ will be to μ, making the estimates more dependable. Plus, the variability of the sample mean decreases, leading to tighter confidence intervals and more robust conclusions.
In essence, the CLT empowers researchers. It reassures them that with enough data, they can make accurate predictions about the broader population. So, if you’re ever doubting the power of numbers, remember the CLT—it’s like a magic trick that reveals the hidden order in chaos!
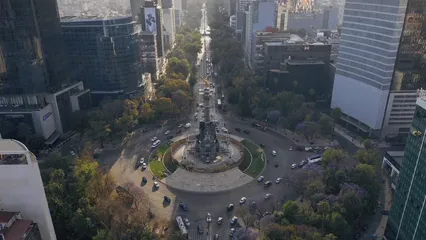
Practical Applications and Limitations
Applications of the Population Mean in Different Fields
The population mean (μ) isn’t just a statistical abstract; it’s a practical tool used across various fields. From research to market analysis, μ helps professionals make informed decisions. Let’s look at some real-world applications.
In research, μ plays a crucial role in understanding data trends. For instance, in a health study examining blood pressure levels across a city, researchers collect data from all residents. By calculating μ, they identify the average blood pressure in the population, guiding public health initiatives.
Consider market analysis, where businesses leverage μ to assess consumer behavior. Companies often conduct surveys to gather customer feedback on products. By calculating the average satisfaction score (μ), they can gauge overall product performance. If the mean score dips, it’s a signal to investigate potential improvements. To get a deeper understanding of market analysis, consider How to Measure Anything: Finding the Value of “Intangibles” in Business.
Quality control in manufacturing also relies heavily on μ. Factories monitor product dimensions, weight, or other critical metrics to ensure consistency. By calculating the average of these measurements, companies can quickly detect deviations from quality standards. If μ shifts unexpectedly, it could indicate a problem in the production process, prompting immediate corrective action.
A fascinating case study comes from an automotive manufacturer. They tracked the average fuel efficiency (μ) of their vehicles. When they noticed a drop in μ, they investigated. Turns out, a specific model had a faulty component affecting performance. By addressing the issue, they improved customer satisfaction and regained market trust.
In education, μ is used to evaluate student performance. Schools calculate the average test scores of students to assess overall academic health. If the average score drops, it could signal the need for curriculum adjustments or additional support for struggling students. For more insights into educational statistics, consider Statistics in Plain English.
In the realm of economics, μ assists in understanding national indicators. Economists calculate the average income (μ) of citizens to analyze economic health. If μ trends up, it may suggest a growing economy; if μ trends down, it could raise concerns about rising poverty levels. For insights on data interpretation in economics, see Inferential Statistics vs Descriptive Statistics in Data Interpretation.
Understanding how to interpret data is essential in economics. Inferential Statistics vs Descriptive Statistics in Data Interpretation provides valuable insights on this topic.
While the applications of μ are vast, it’s essential to recognize its limitations. Relying solely on the population mean can be misleading, especially in datasets with outliers. A few extreme values can skew μ, presenting a distorted view of the population. Therefore, it’s vital to consider the data’s distribution shape and other statistical measures like median and mode to gain a comprehensive understanding.
In summary, the population mean (μ) is indispensable across multiple sectors. Whether in research, business, or education, μ helps illuminate trends and inform decision-making. Just remember to tread carefully when interpreting its value, as the broader context can significantly influence its implications.

Limitations and Challenges
When it comes to the population mean (μ), it’s essential to approach it with a discerning eye. While μ is a fantastic tool, relying on it exclusively can lead to some pitfalls. One of the most significant challenges is its sensitivity to outliers. Imagine a classroom where most students score between 70 and 85 on a test. Now, one student scores a staggering 99. The mean suddenly jumps, giving an inaccurate representation of the overall performance. This sneaky little number can distort the reality of your data, making it seem like everyone aced the test.
Furthermore, understanding the shape of the data distribution is crucial when interpreting μ. Data can be skewed, symmetric, or have multiple peaks—oh, the drama! If the data distribution is skewed, the population mean can be misleading. For instance, in a right-skewed distribution, the mean will be higher than the median. This can create a false impression of the central tendency. Ignoring the distribution’s shape may lead to misguided conclusions about the data and can affect decision-making.
In short, while μ is a powerful statistical measure, it’s vital to consider outliers and the data distribution shape. Ignoring these factors can lead to distorted insights, much like trying to solve a puzzle with missing pieces. Always be sure to take a step back and assess the bigger picture before making conclusions based on the population mean.
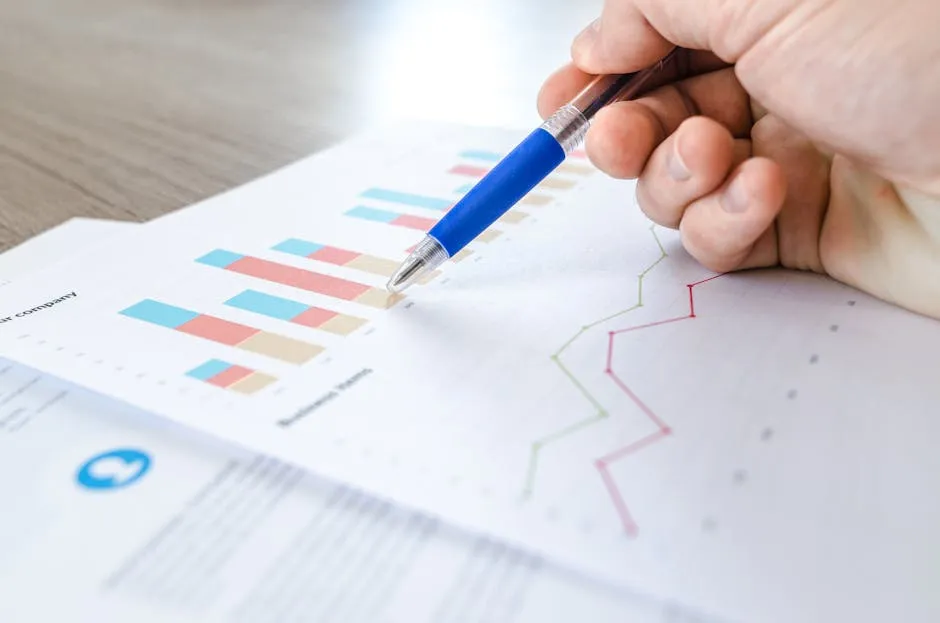
Conclusion
Understanding the population mean (μ) is a cornerstone of statistical analysis. Throughout this article, we’ve explored its definition, calculation, and significance. We’ve seen that μ serves not just as a number but as a vital reference point that helps us comprehend data trends.
We began by defining μ as the average of a population, calculated through a straightforward formula. However, the journey didn’t stop there. We discovered its role in determining central tendency, guiding data interpretation, and aiding in hypothesis testing. In essence, μ is the compass that helps navigate the sometimes murky waters of data analysis.
We also touched on the applications of μ in fields like social sciences, economics, and quality control. By calculating μ, researchers and professionals gain insights into average behaviors and outcomes, allowing for informed decision-making. Whether assessing average incomes or evaluating student performance, μ is invaluable.
Yet, it’s crucial to remember the limitations associated with μ. Outliers and the shape of data distribution can distort its true meaning. Therefore, a comprehensive analysis should consider these factors to avoid misleading conclusions.
In conclusion, understanding the population mean (μ) is essential for anyone venturing into the world of statistics. It lays the groundwork for further exploration of statistical concepts and their practical applications. So, whether you’re a student, researcher, or data enthusiast, take the time to grasp μ and its implications. The world of statistics is vast, and μ is just the beginning. Dive deeper into other statistical concepts, and you’ll find a treasure trove of insights waiting to be uncovered!

FAQs
Please let us know what you think about our content by leaving a comment down below!
Thank you for reading till here 🙂
All images from Pexels