Introduction
Inferential statistics is like the magician of the data world, transforming a small sample into a grand illusion of insights about an entire population. Using principles of probability, inferential statistics allows us to draw conclusions and make predictions based on data collected from a subset of individuals or items. It’s relevant in various fields, from psychology to marketing, where understanding a larger group without examining every single member is essential.
But hold on! Before we get too carried away, let’s clarify our terms. Descriptive statistics simply summarize data—think averages and percentages—while inferential statistics take it a step further. They help us infer or predict characteristics of a larger population from a smaller sample. So, if descriptive statistics are the appetizers, inferential statistics are the main course, complete with a side of hypothesis testing.
This article aims to shine a light on examples of inferential statistics while clearing up misconceptions. We’ll discuss how sampling and hypothesis testing are the bread and butter of inferential statistics. Sampling allows us to gather manageable data, and hypothesis testing helps us determine if observed results are statistically significant. Let’s dive into the fascinating world of inferential statistics and see what treasures await!
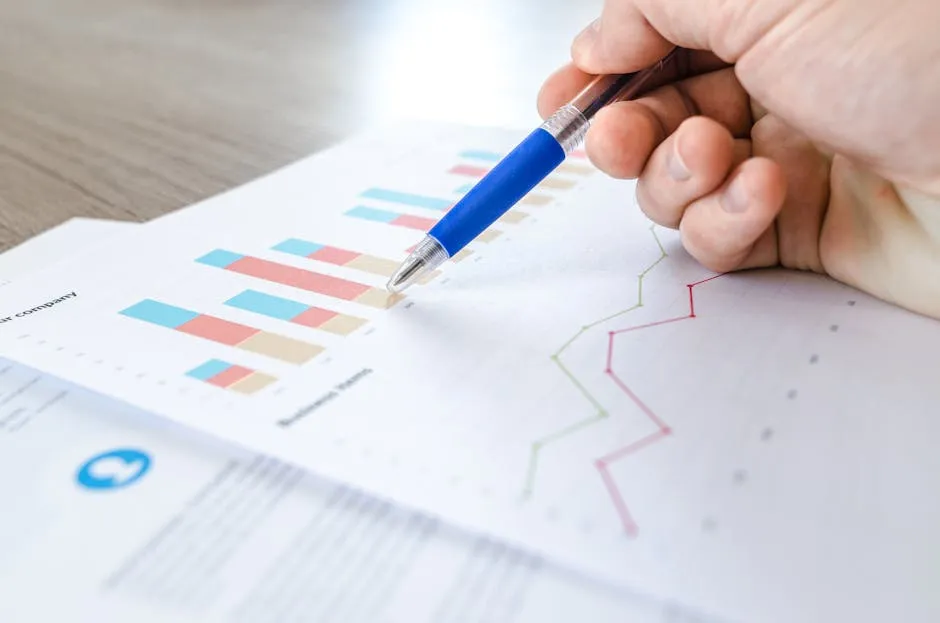
What is Inferential Statistics?
Definition and Overview
Inferential statistics is a branch of statistics that uses sample data to make inferences about a population. It’s not merely about crunching numbers; it’s about drawing conclusions that can help guide decisions and predictions. For instance, if a researcher wants to know the average height of adult males in a country, they wouldn’t measure every single adult. Instead, they’d take a random sample and use inferential methods to estimate the average height for the entire population.
Now, how does this differ from descriptive statistics? While descriptive statistics summarize data from a sample or population, inferential statistics go a step further by using that sample to make predictions or generalizations about a broader group. It’s like looking at a tiny slice of cake and trying to guess the flavor of the whole cake. Not always perfect, but it often works!
If you’re looking to deepen your understanding of statistics, consider picking up Statistics for Dummies (Paperback). It’s a humorous yet informative guide that makes complex concepts accessible, perfect for the novice statistician!
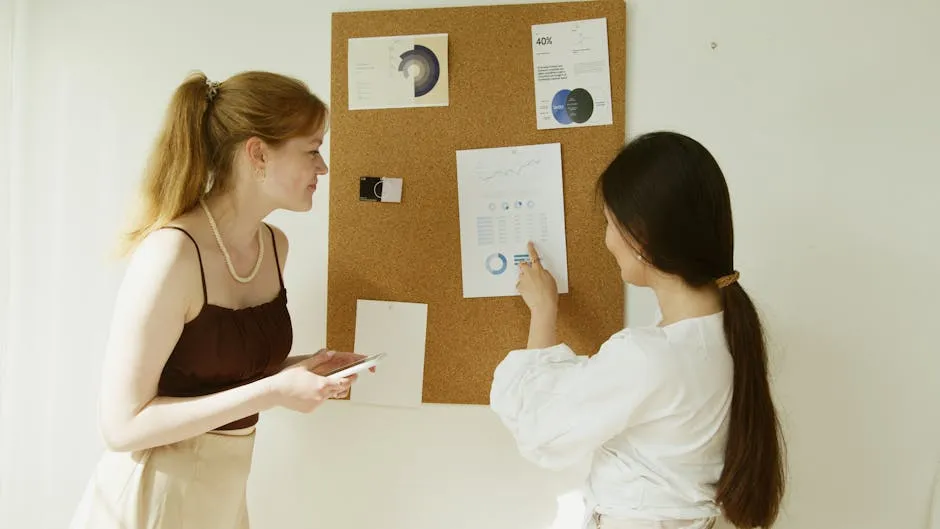
Key Concepts
Several key concepts underpin inferential statistics. First, we have the population, which refers to the entire group of interest. Then, there’s the sample, a smaller subset of the population selected for analysis. The parameter is a characteristic of the population (like the true average height), while a statistic is a characteristic of the sample (like the average height of the sampled individuals). For more information on parameters, check out complete statistics how to prove parameter space contain open set.
Another important concept is the sampling distribution. This distribution describes how a statistic, like the sample mean, varies from sample to sample. It’s crucial for understanding how reliable our inferences are. Lastly, we must not forget the hypothesis testing process, which involves making claims about a population and determining whether there’s enough evidence in the sample data to support those claims.
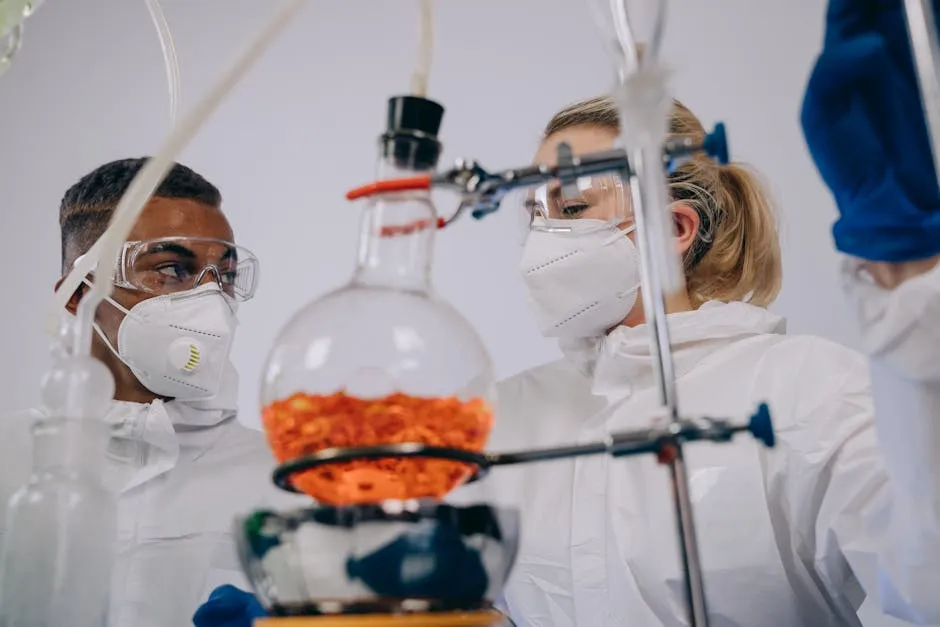
Importance in Research
Inferential statistics plays a pivotal role in various fields. In psychology, researchers may want to understand how a new therapy impacts a population. Instead of testing every individual, they can conduct a study on a sample and make inferences about the larger group. In marketing, companies use inferential statistics to predict consumer behavior based on a survey of a small group. Healthcare researchers often rely on inferential statistics to assess the effectiveness of new treatments through clinical trials.
In summary, inferential statistics goes beyond mere data collection. It allows us to make informed predictions and decisions, all while acknowledging the limitations and uncertainties inherent in working with samples. With its ability to transform a small amount of data into valuable insights, inferential statistics is an indispensable tool in research and decision-making.

Types of Inferential Statistics
Inferential statistics is a powerful tool that helps us make predictions and decisions about a population based on a sample of data. Let’s break down its main types: hypothesis testing, estimation, and regression analysis.
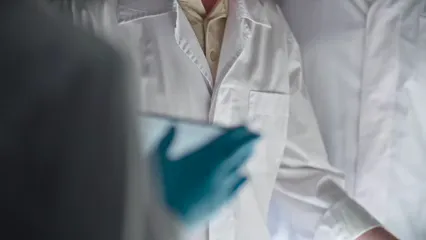
Hypothesis Testing
Hypothesis testing is like being a detective for data. It involves making claims, or hypotheses, about a population and then using sample data to test these claims. In hypothesis testing, we create two opposing statements: the null hypothesis (H0) and the alternative hypothesis (H1).
The null hypothesis usually suggests no effect or no difference. For example, it might state that a new drug has no impact on recovery time. On the other hand, the alternative hypothesis posits that some effect does exist, suggesting that the drug does improve recovery time.
Once we state our hypotheses, we need to assess their validity using a significance level (often set at 0.05). This level indicates the probability of rejecting the null hypothesis when it’s actually true—a mistake called a Type I error. The p-value plays a crucial role here. It tells us the probability of observing our data (or something more extreme) if the null hypothesis is true. If the p-value is less than the significance level, we reject the null hypothesis. Voilà! The data suggests a significant effect!

Estimation
Estimation in inferential statistics helps us make educated guesses about population parameters based on sample statistics. There are two main types of estimates: point estimates and interval estimates.
Point Estimates provide a single value as the best guess for a population parameter. For instance, if we want to know the average height of a population, we might sample 100 people and find their average height is 5’7″. This average is our point estimate for the population mean.
Interval Estimates, on the other hand, give us a range of values within which we expect the true population parameter to fall. This is commonly presented as a confidence interval. For example, if our sample mean is 5’7″ with a 95% confidence interval of [5’5″, 5’9″], we’re saying we are 95% confident that the true average height of the population lies between these two values. To calculate this interval, we consider the sample mean, the standard deviation, and the size of the sample. You can learn more about this in how to calculate test statistic for confidence interval ti84.
Confidence intervals are essential because they provide a measure of uncertainty around our estimate, allowing us to understand the precision of our data. If you’re eager to expand your knowledge further, consider How to Measure Anything: Finding the Value of “Intangibles” in Business (Paperback). This book provides insights into quantifying the unquantifiable!
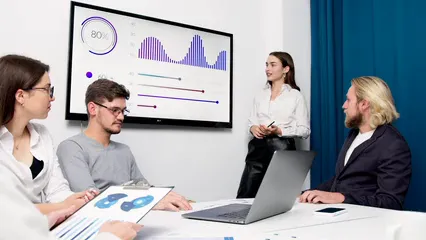
Regression Analysis
Regression analysis is another critical aspect of inferential statistics. It helps us understand relationships between variables. Picture this: you’re trying to predict someone’s height based on their age. Regression lets you model that relationship mathematically.
There are different types of regression analysis. Simple linear regression examines the relationship between two variables, where one is dependent on the other. For example, it might analyze how study time affects test scores.
Multiple regression, on the other hand, looks at the relationship between one dependent variable and multiple independent variables. This is useful when you suspect that several factors influence an outcome. For instance, predicting house prices might involve variables like size, location, and the number of bedrooms.
Regression analysis not only helps us understand relationships but also allows us to make predictions. By fitting a regression model to our data, we can forecast outcomes based on new data points. It’s like having a crystal ball, but one grounded in numbers. For a deeper dive into practical applications, check out The Art of Statistics: Learning from Data (Hardcover). It’s a fantastic resource for anyone looking to sharpen their analytical skills!
In conclusion, understanding these types of inferential statistics equips us with the tools to draw meaningful conclusions from our data. Whether we’re testing hypotheses, estimating population parameters, or analyzing relationships, inferential statistics is key to making informed decisions.
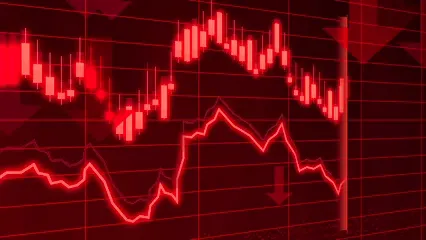
Examples of Inferential Statistics
When it comes to statistics, inferential statistics is the superstar that helps us make educated guesses about a larger group based on a smaller sample. Let’s explore some compelling examples that illustrate this concept.
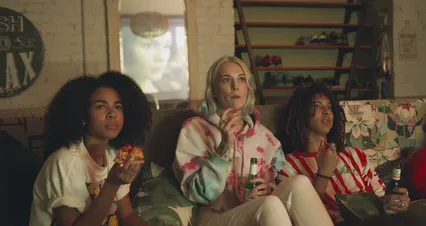
Common Examples
Example 1: Testing Longevity of Light Bulbs
Imagine a manufacturer producing thousands of light bulbs. Testing each bulb for longevity would be a logistical nightmare and an expensive endeavor. Instead, they opt for a clever solution. By testing a sample of 100 light bulbs, they can infer the overall quality of all the bulbs produced.
How does this work? The company runs various tests on the sample to assess factors like brightness and lifespan. If the sample performs well, the manufacturer can confidently conclude that the entire batch should meet quality standards. This approach saves time and resources while still providing reliable insights into product quality. If you’re intrigued by the topic of quality and data, consider The Data Warehouse Toolkit: The Definitive Guide to Dimensional Modeling (Hardcover). It’s a key resource for understanding data management!
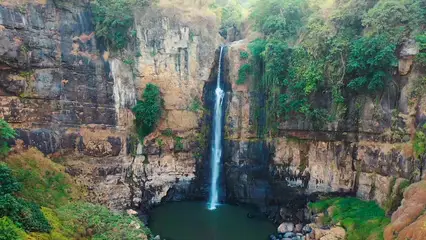
Example 2: Predicting Average Height
Let’s say a researcher wants to know the average height of adult males in a country. Instead of measuring everyone—a task as daunting as herding cats—they randomly select a sample of 200 adult males.
After measuring their heights, the researcher calculates the average, say 5 feet 10 inches. With this sample mean, they can confidently estimate the average height of all adult males in the country. This method, using sample data to predict population parameters, is a hallmark of inferential statistics. If you want to dive deeper into statistical methodologies, check out Inferential Statistics: A Practical Guide (Paperback). It’s a great resource for practical applications!
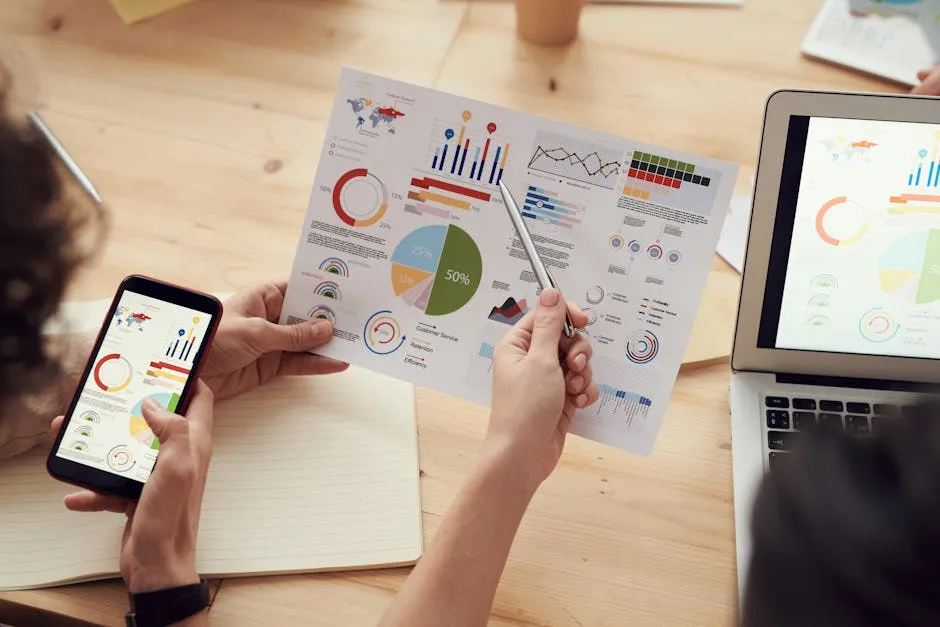
Survey Sampling
Surveys are a powerful tool in research, allowing us to generalize findings from a small sample to a broader population. This is where inferential statistics shines!
For instance, in market research, a company might survey 1,000 customers to gauge satisfaction with a new product. By analyzing the survey results, they can infer how the entire customer base might feel about the product. If 80% of respondents are satisfied, the company can confidently project that a similar percentage of all customers would feel the same way.
However, the success of this approach hinges on proper sampling techniques. If the sample isn’t representative—say, if it consists solely of loyal customers—the results may lead to erroneous conclusions. So, the next time you fill out a survey, remember: your responses could influence decisions affecting many!
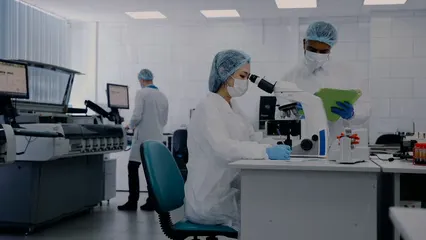
Clinical Trials
Clinical trials are perhaps the most critical application of inferential statistics. When testing a new drug, researchers can’t possibly give it to every patient out there. Instead, they select a sample of patients to determine the drug’s efficacy and safety.
For example, suppose a pharmaceutical company wants to test a new flu vaccine. They recruit 1,000 participants and randomly assign half to receive the vaccine and the other half to receive a placebo. By analyzing the health outcomes of both groups, they can infer the vaccine’s effectiveness for the general population. If the vaccinated group shows significantly fewer flu cases, the researchers can conclude that the vaccine is likely effective.
Thus, clinical trials provide essential insights that guide healthcare decisions, all thanks to the power of inferential statistics. For those interested in the intersection of health and data, consider Clinical Trials: A Practical Guide (Paperback). It’s a valuable resource for understanding trial methodologies!
By utilizing these examples, we see how inferential statistics allows us to make well-informed predictions and decisions based on limited data. Whether we’re testing light bulbs, measuring heights, conducting surveys, or testing drugs, inferential statistics helps us draw valuable conclusions that guide our understanding of larger populations.
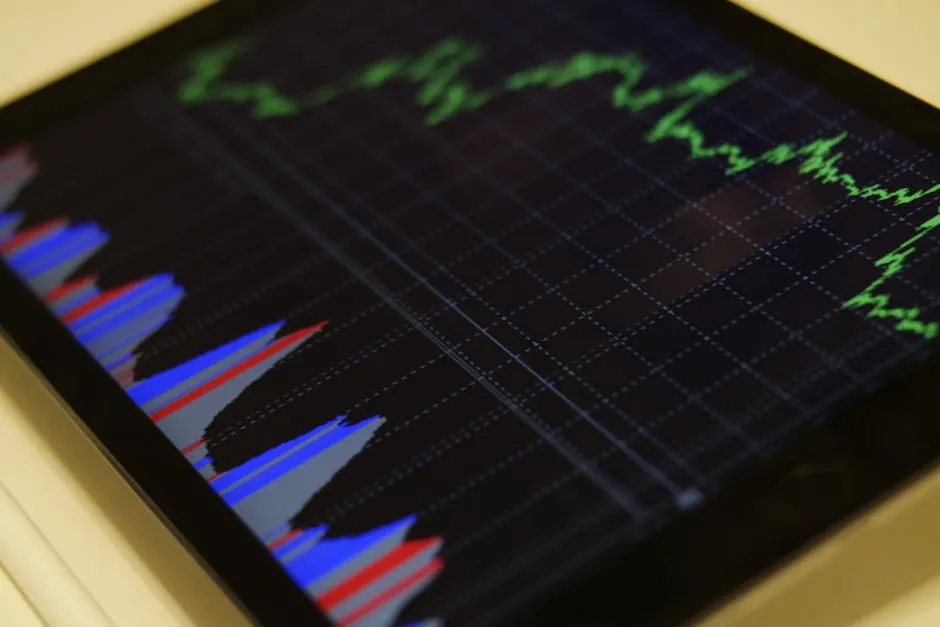
Misconceptions About Inferential Statistics
Inferential statistics is often misunderstood, leading to confusion with descriptive statistics. Let’s clear up a few of these common misconceptions.
First off, not everything derived from a sample is inferential. Many people think that simply calculating averages or percentages from a sample means they’re using inferential statistics. Wrong! If you find the average height of 50 students, you’re simply summarizing that specific group. That’s descriptive statistics, my friend! Inferential statistics requires you to make predictions or generalizations about a larger population based on that sample data.
Another misconception is the belief that any sampling method will yield valid results. The truth is, how you select your sample is crucial. Random sampling is essential for inferential statistics to work effectively. If your sample is biased—say, all tall people or only those who love basketball—your conclusions about the entire population will likely be off the mark. So, you see, sampling methods matter more than you think.
People also often assume that inferential statistics guarantees accuracy. Spoiler alert: it doesn’t! In fact, all inferential statistics involve a degree of uncertainty. That’s the nature of working with samples instead of entire populations. Just because you have a statistically significant result doesn’t mean it’s the gospel truth. It merely indicates that your results are likely representative of the population, given the sample you analyzed.
Moreover, inferential statistics does not mean you can predict the future with certainty. It allows you to make educated guesses, but those guesses come with margins of error. It’s like trying to predict if it will rain tomorrow based on today’s weather. Sure, you can make an educated guess, but don’t forget to bring an umbrella just in case!
Finally, many believe that inferential statistics is only applicable in research settings. However, businesses, marketers, and even everyday decision-makers use it to draw conclusions from small data sets. Whether it’s estimating customer satisfaction or predicting market trends, inferential statistics is a tool for anyone making decisions based on data.
Understanding these misconceptions helps you appreciate the real power of inferential statistics. It’s a bridge between the sample and the larger population, but only if navigated wisely!

Best Practices in Inferential Statistics
When it comes to using inferential statistics effectively, adhering to best practices is essential. Here are some key steps to ensure your analyses yield meaningful results.
First, random sampling is non-negotiable. It’s the gold standard for ensuring your sample accurately represents the population. If you’re conducting a survey, don’t just ask your friends or the first 50 people you see. Instead, use methods like stratified sampling to ensure diversity. This way, you can be more confident that your conclusions apply to the broader population.
Next up is sample size. A tiny sample might lead to misleading results. Think about it: a sample of 10 people can’t possibly represent a city of a million! Larger samples reduce variability and improve the reliability of your estimates. But remember, bigger isn’t always better. You want a sample size that balances accuracy with practicality.
Avoiding biases in data collection is another critical practice. Bias can creep in at any stage, from how you select participants to how you frame your questions. For example, leading questions can influence responses, skewing your data. So, keep your questions neutral and straightforward!
It’s also vital to understand the limitations of your inferential statistics. Remember, results are based on probabilities, not certainties. Confidence intervals provide a range where the true population parameter is likely to fall, but they don’t guarantee that the parameter lies within that range. Always interpret your results with caution and communicate these limitations when sharing findings.
Lastly, continually educate yourself about the tools and techniques in inferential statistics. The field is always evolving. New methods and practices emerge regularly, and keeping up with them will sharpen your analytical skills. If you’re interested in the latest in data science, check out The Data Science Handbook (Paperback). It’s a comprehensive guide to modern data analysis!
In conclusion, following these best practices ensures your use of inferential statistics is robust and reliable. By adhering to sound sampling methods, understanding biases, and recognizing limitations, you can draw meaningful conclusions that stand the test of scrutiny. Now, go forth and conquer your data with confidence!
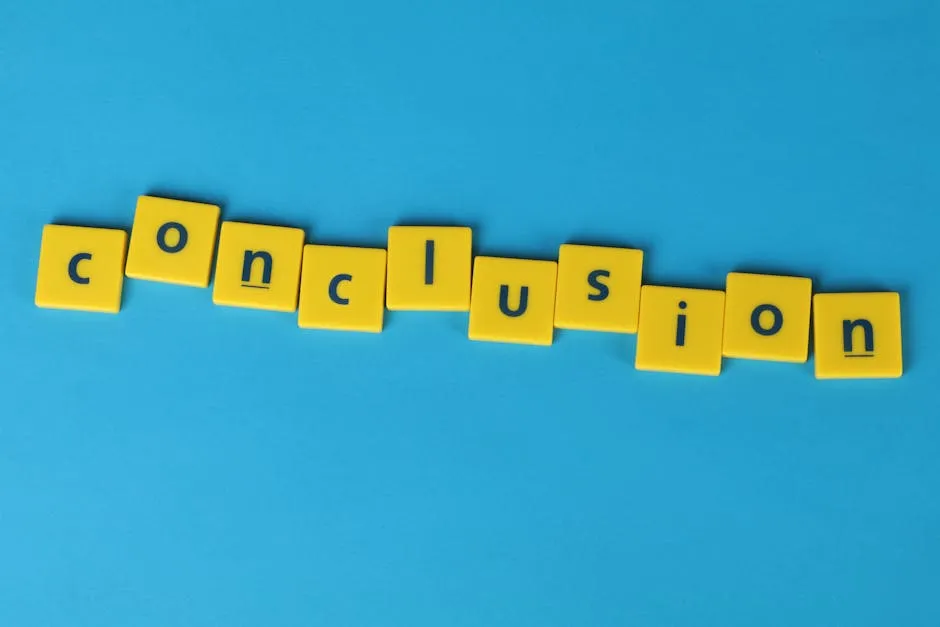
Conclusion
In this article, we journeyed through the fascinating world of inferential statistics. We learned how this branch of statistics enables us to make predictions and draw conclusions about larger populations based on sample data. By understanding key concepts such as population, sample, parameters, and statistics, we laid a solid foundation for our exploration.
We emphasized the importance of hypothesis testing and estimation. Hypothesis testing allows researchers to make evidence-based claims, while estimation provides a range of values, offering insight into population parameters. Regression analysis was also highlighted as a powerful tool to understand relationships between variables.
Inferential statistics plays a pivotal role in various fields, from healthcare to marketing. Researchers and decision-makers rely on these techniques to make informed choices based on limited data. The ability to generalize findings helps organizations assess trends, customer preferences, and the effectiveness of interventions.
It is crucial to apply these concepts in your own statistical analyses. Whether you’re conducting a survey, running a clinical trial, or analyzing market data, inferential statistics can provide valuable insights. Remember to use appropriate sampling methods and recognize the limitations of your data.
As you venture into the realm of statistics, keep the principles of inferential statistics close. They are not just tools for researchers; they are keys to unlocking the mysteries hidden within data. Embrace the power of inference, and let your analyses lead you to meaningful conclusions that can drive progress and innovation.
FAQs
What is the difference between descriptive and inferential statistics?
Descriptive statistics summarizes data characteristics, such as averages and percentages. It provides a clear picture of a specific dataset without making predictions about a larger population. In contrast, inferential statistics uses sample data to infer or generalize characteristics of a broader population. This process involves hypothesis testing and estimation, allowing researchers to draw conclusions beyond the immediate data.
Why is sampling important in inferential statistics?
Sampling is crucial because it allows researchers to make inferences about a population without examining every individual. A well-chosen sample represents the larger group, providing valid results. If a sample is biased or too small, the conclusions drawn may be inaccurate. Thus, proper sampling techniques ensure that findings can be generalized to the entire population.
Can you give an example of a hypothesis test?
Certainly! Imagine a new medication is developed to reduce blood pressure. Researchers want to test if the medication is effective. They randomly assign 100 patients to receive either the medication or a placebo. The null hypothesis (H0) states that the medication has no effect on blood pressure, while the alternative hypothesis (H1) posits that it does. After conducting the study, researchers analyze the data to determine if there’s enough evidence to reject the null hypothesis.
What are some common statistical tests used in inferential statistics?
Several statistical tests are frequently used: – **t-tests**: Compare the means of two groups to see if they differ significantly. – **ANOVA (Analysis of Variance)**: Assesses differences between three or more groups. – **Chi-square tests**: Examines the relationship between categorical variables. – **Regression analysis**: Evaluates the relationship between variables and makes predictions about outcomes.
How do confidence intervals work?
Confidence intervals provide a range of values within which a population parameter is likely to fall. For instance, if a sample mean is calculated as 50 with a 95% confidence interval of [48, 52], it suggests that if the study were repeated, 95% of the time, the true population mean would lie between 48 and 52. Confidence intervals reflect the uncertainty inherent in using sample data to estimate population parameters.
Please let us know what you think about our content by leaving a comment down below!
Thank you for reading till here 🙂
All images from Pexels